On Detecting Hidden Third-Party Web Trackers with a Wide Dependency Chain Graph: A Representation Learning Approach
arxiv(2020)
摘要
Websites use third-party ads and tracking services to deliver targeted ads and collect information about users that visit them. These services put users privacy at risk and that's why users demand to block these services is growing. Most of the blocking solutions rely on crowd-sourced filter lists that are built and maintained manually by a large community of users. In this work, we seek to simplify the update of these filter lists by automatic detection of hidden advertisements. Existing tracker detection approaches generally focus on each individual website's URL patterns, code structure and/or DOM structure of website. Our work differs from existing approaches by combining different websites through a large scale graph connecting all resource requests made over a large set of sites. This graph is thereafter used to train a machine learning model, through graph representation learning to detect ads and tracking resources. As our approach combines different sources of information, it is more robust toward evasion techniques that use obfuscation or change usage patterns. We evaluate our work over the Alexa top-10K websites, and find its accuracy to be 90.9% also it can block new ads and tracking services which would necessitate to be blocked further crowd-sourced existing filter lists. Moreover, the approach followed in this paper sheds light on the ecosystem of third party tracking and advertising
更多查看译文
关键词
wide dependency chain graph,third-party
AI 理解论文
溯源树
样例
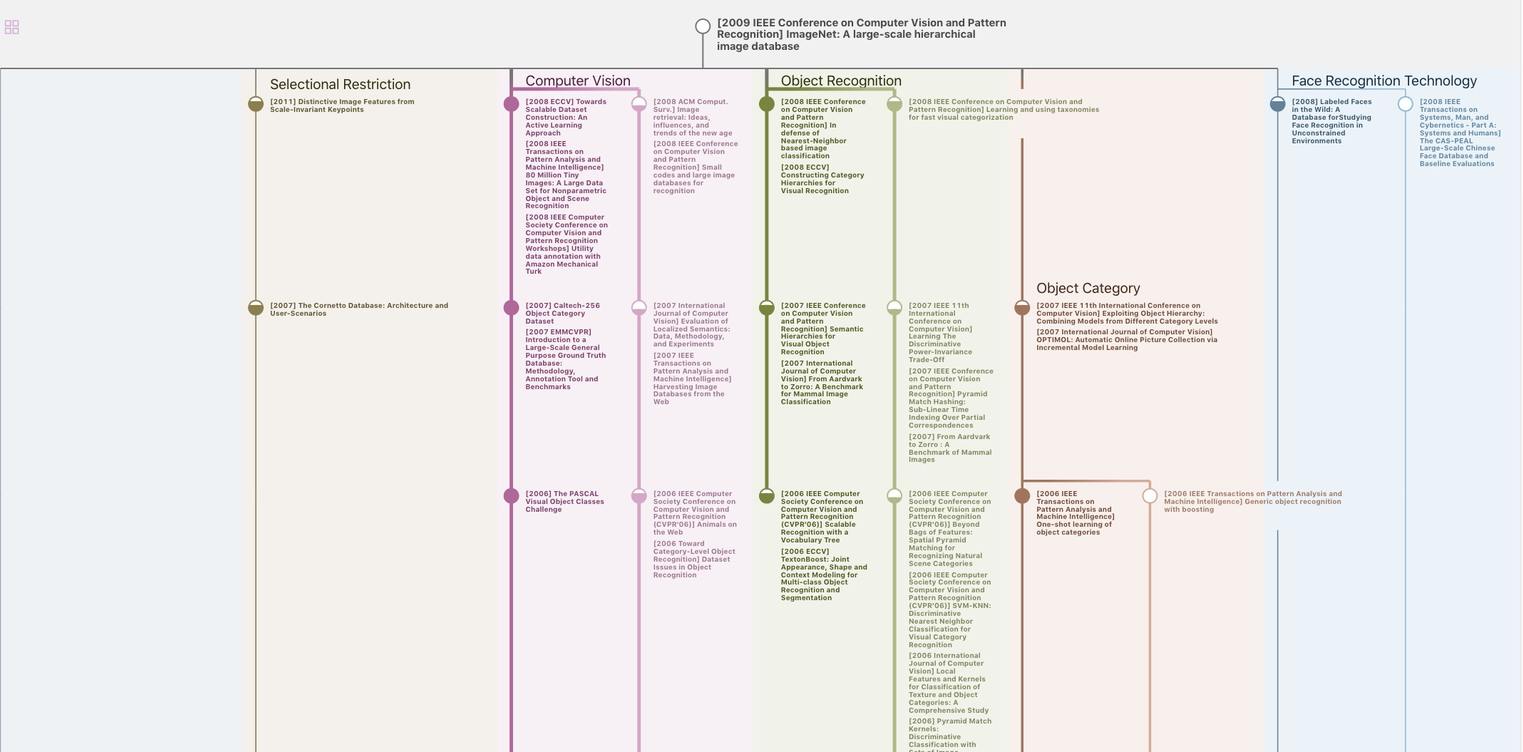
生成溯源树,研究论文发展脉络
Chat Paper
正在生成论文摘要