TransOMCS: From Linguistic Graphs to Commonsense Knowledge
IJCAI 2020(2020)
摘要
Commonsense knowledge acquisition is a key problem for artificial intelligence. Conventional methods of acquiring commonsense knowledge generally require laborious and costly human annotations, which are not feasible on a large scale. In this paper, we explore a practical way of mining commonsense knowledge from linguistic graphs, with the goal of transferring cheap knowledge obtained with linguistic patterns into expensive commonsense knowledge. The result is a conversion of ASER [Zhang et al., 2020], a large-scale selectional preference knowledge resource, into TransOMCS, of the same representation as ConceptNet [Liu and Singh, 2004] but two orders of magnitude larger. Experimental results demonstrate the transferability of linguistic knowledge to commonsense knowledge and the effectiveness of the proposed approach in terms of quantity, novelty, and quality. TransOMCS is publicly available at: https://github.com/HKUST-KnowComp/TransOMCS.
更多查看译文
关键词
linguistic graphs,knowledge
AI 理解论文
溯源树
样例
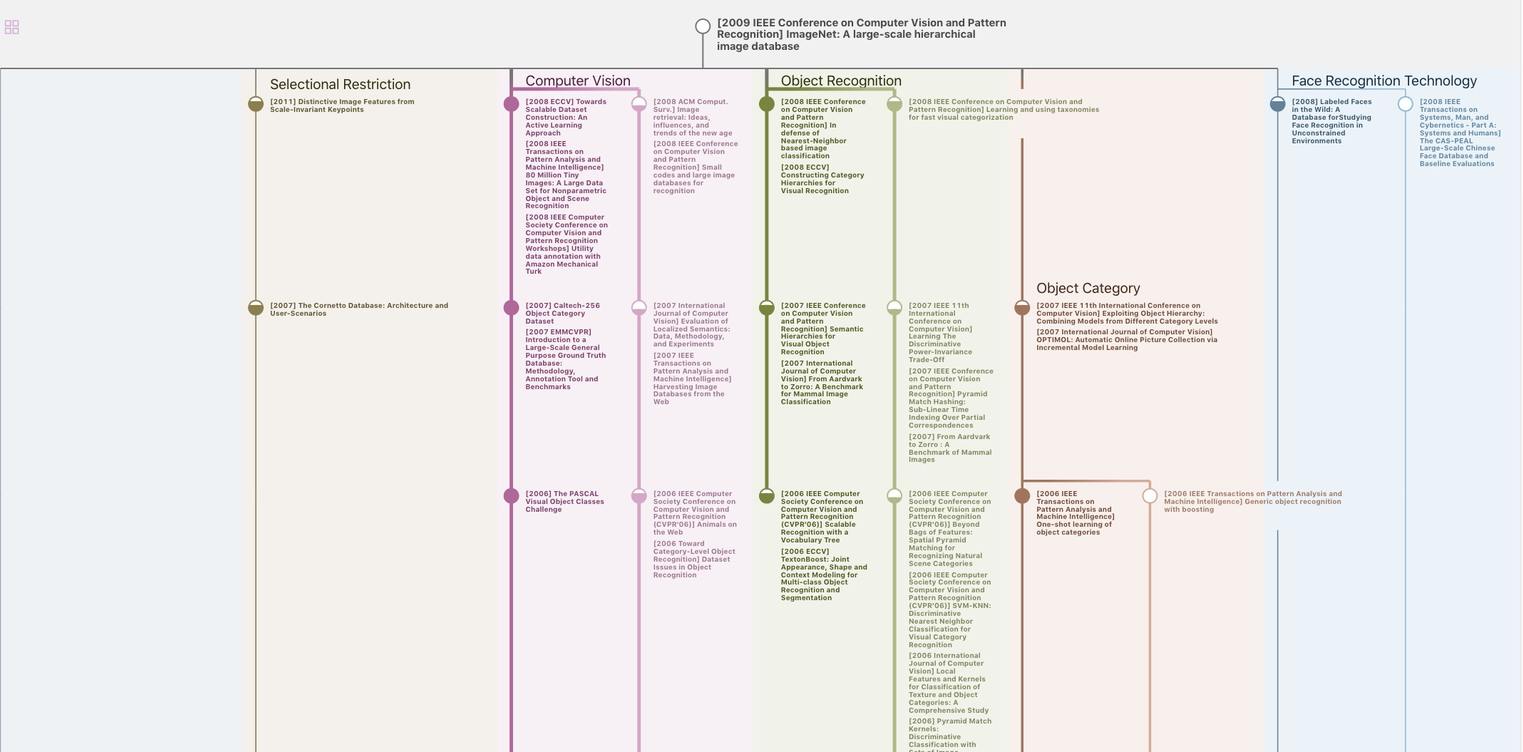
生成溯源树,研究论文发展脉络
Chat Paper
正在生成论文摘要