Selecting Backtranslated Data from Multiple Sources for Improved Neural Machine Translation
58TH ANNUAL MEETING OF THE ASSOCIATION FOR COMPUTATIONAL LINGUISTICS (ACL 2020)(2020)
摘要
Machine translation (MT) has benefited from using synthetic training data originating from translating monolingual corpora, a technique known as backtranslation. Combining backtranslated data from different sources has led to better results than when using such data in isolation. In this work we analyse the impact that data translated with rule-based, phrase-based statistical and neural MT systems has on new MT systems. We use a real-world low-resource use-case (Basque-to-Spanish in the clinical domain) as well as a high-resource language pair (German-to-English) to test different scenarios with backtranslation and employ data selection to optimise the synthetic corpora. We exploit different data selection strategies in order to reduce the amount of data used, while at the same time maintaining high-quality MT systems. We further tune the data selection method by taking into account the quality of the MT systems used for backtranslation and lexical diversity of the resulting corpora. Our experiments show that incorporating backtranslated data from different sources can be beneficial, and that availing of data selection can yield improved performance.
更多查看译文
关键词
backtranslated data,multiple sources,translation
AI 理解论文
溯源树
样例
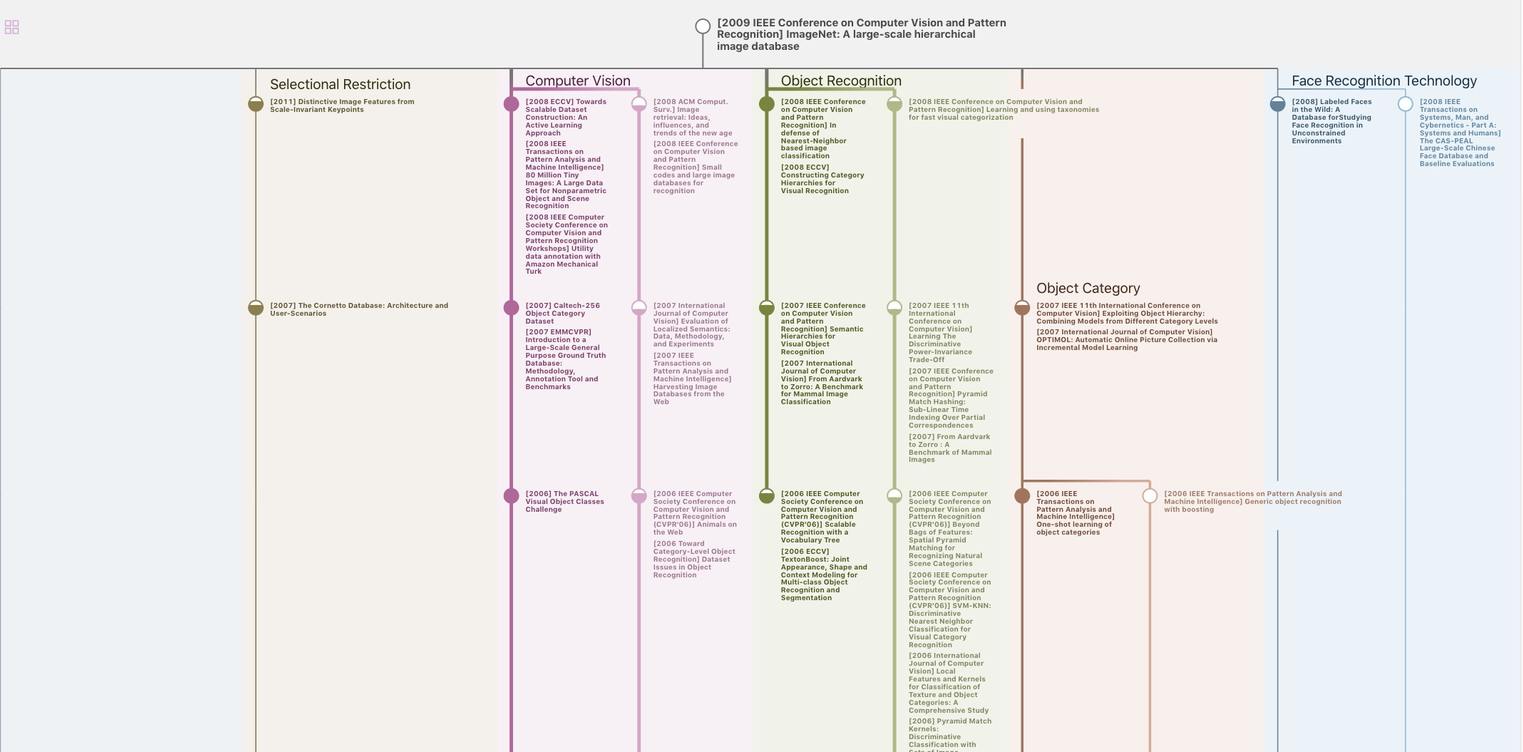
生成溯源树,研究论文发展脉络
Chat Paper
正在生成论文摘要