Accounting for Range Uncertainties in the Optimization of Combined Proton-Photon Treatments Via Stochastic Optimization
International Journal of Radiation Oncology*Biology*Physics(2020)
摘要
Purpose: Proton treatment slots are a limited resource. Combined proton-photon treatments, in which most fractions are delivered with photons and only a few with protons, may represent a practical solution to optimize the allocation of proton resources over the patient population. We demonstrate how a limited number of proton fractions can be optimally used in multimodality treatments and address the issue of the robustness of combined treatments against proton range uncertainties.Methods and Materials: Combined proton-photon treatments are planned by simultaneously optimizing intensity modulated radiation therapy and proton therapy plans while accounting for the fractionation effect through the biologically effective dose model. The method was investigated for different tumor sites (a spinal metastasis, a sacral chordoma, and an atypical meningioma) in which organs at risk (OARs) were located within or near the tumor. Stochastic optimization was applied to mitigate range uncertainties.Results: In optimal combinations, proton and photon fractions deliver similar doses to OARs overlaying the target volume to protect these dose-limiting normal tissues through fractionation. Meanwhile, parts of the tumor are hypofractionated with protons. Thus, the total dose delivered with photons is reduced compared with simple combinations in which each modality delivers the prescribed dose per fraction to the target volume. The benefit of optimal combinations persists when range errors are accounted for via stochastic optimization.Conclusions: Limited proton resources are optimally used in combined treatments if parts of the tumor are hypofractionated with protons and near-uniform fractionation is maintained in serial OARs. Proton range uncertainties can be efficiently accounted for through stochastic optimization and are not an obstacle for clinical application. (C) 2020 Elsevier Inc. All rights reserved.
更多查看译文
AI 理解论文
溯源树
样例
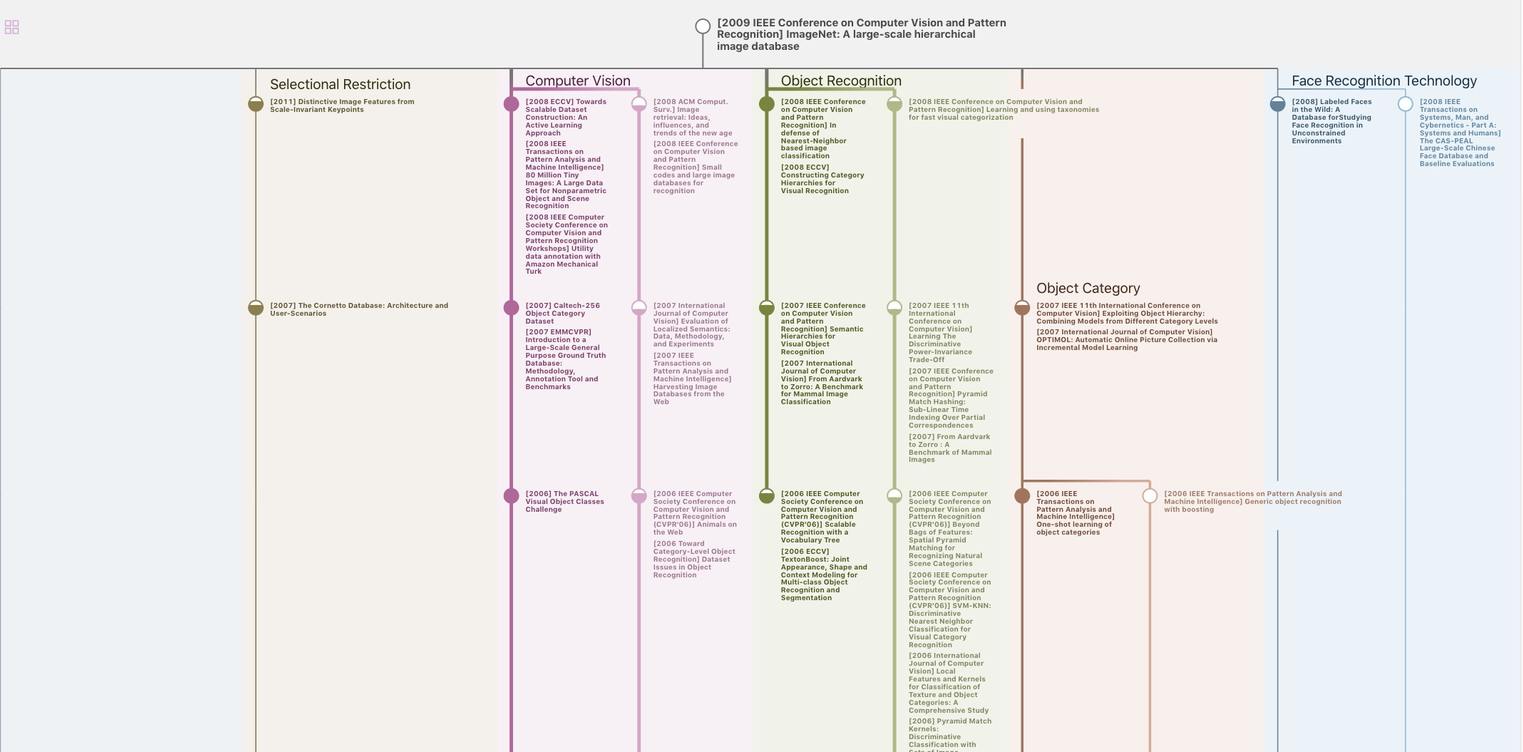
生成溯源树,研究论文发展脉络
Chat Paper
正在生成论文摘要