Selection of Eye-Tracking Stimuli for Prediction by Sparsely Grouped Input Variables for Neural Networks: towards Biomarker Refinement for Autism
ETRA '20: 2020 Symposium on Eye Tracking Research and Applications Stuttgart Germany June, 2020(2020)
摘要
Eye tracking has become a powerful tool in the study of autism spectrum disorder (ASD). Current, large-scale efforts aim to identify specific eye-tracking stimuli to be used as biomarkers for ASD, with the intention of informing the diagnostic process, monitoring therapeutic response, predicting outcomes, or identifying subgroups with the spectrum. However, there are hundreds of candidate experimental paradigms, each of which contains dozens or even hundreds of individual stimuli. Each stimuli is associated with an array of potential derived outcome variables, thus the number of variables to consider can be enormous. Standard variable selection techniques are not applicable to this problem, because selection must be done at the level of stimuli and not individual variables. In other words, this is a grouped variable selection problem. In this work, we apply lasso, group lasso, and a new technique, Sparsely Grouped Input Variables for Neural Network (SGIN), to select experimental stimuli for group discrimination and regression with clinical variables. Using a dataset obtained from children with and without ASD who were administered a battery containing 109 different stimuli presentations involving 9647 features, we are able to retain strong group separation even with only 11 out of the 109 stimuli. This work sets the stage for concerted techniques designed around engines to iteratively refine and define next-generation biomarkers using eye tracking for psychiatric conditions. http://github.com/beibinli/SGIN
更多查看译文
关键词
eye-tracking, feature selection, group selection, stimuli selection, paradigm selection, neural network, autism
AI 理解论文
溯源树
样例
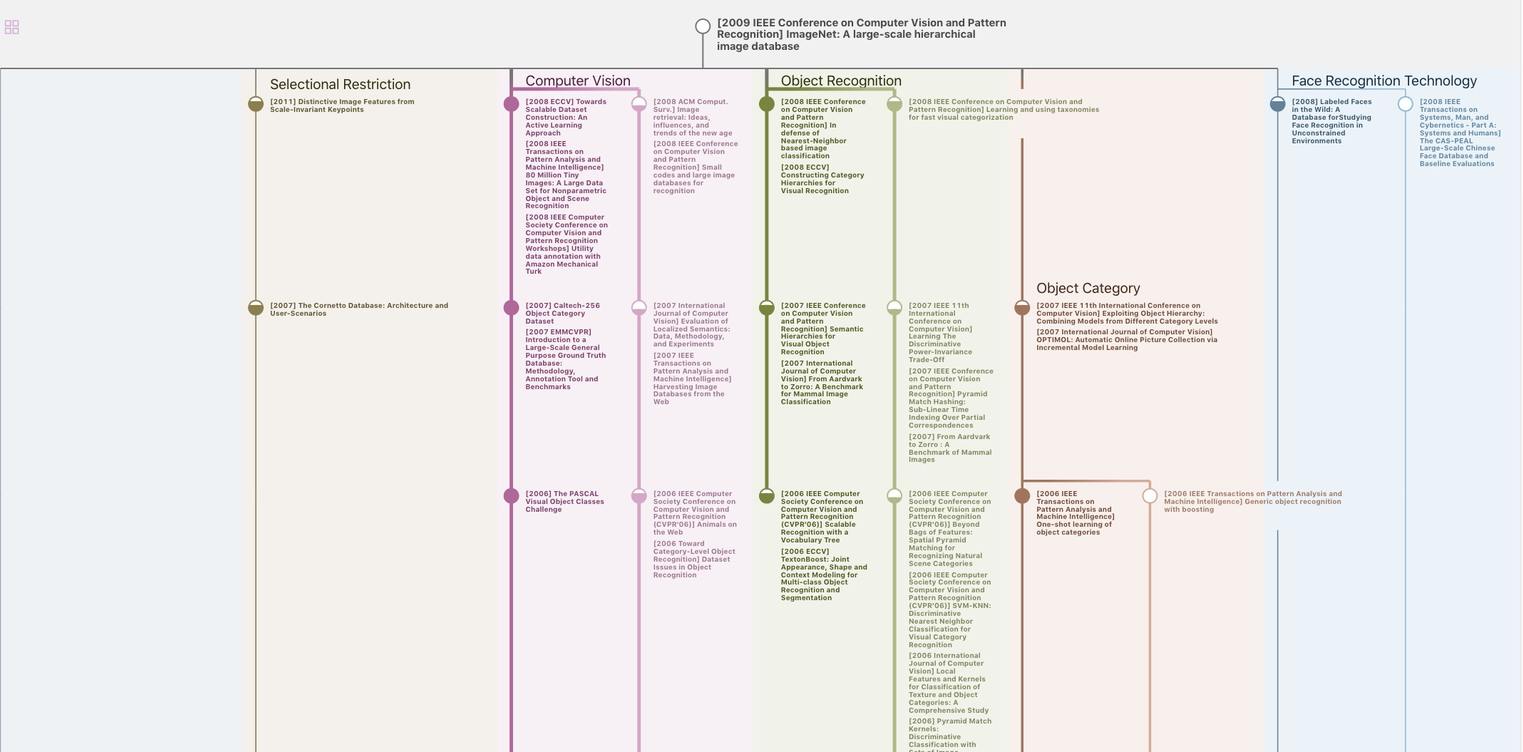
生成溯源树,研究论文发展脉络
Chat Paper
正在生成论文摘要