Cross-Dataset Variability Problem in EEG Decoding With Deep Learning.
FRONTIERS IN HUMAN NEUROSCIENCE(2020)
摘要
Cross-subject variability problems hinder practical usages of Brain-Computer Interfaces. Recently, deep learning has been introduced into the BCI community due to its better generalization and feature representation abilities. However, most studies currently only have validated deep learning models for single datasets, and the generalization ability for other datasets still needs to be further verified. In this paper, we validated deep learning models for eight MI datasets and demonstrated that the cross-dataset variability problem weakened the generalization ability of models. To alleviate the impact of cross-dataset variability, we proposed an online pre-alignment strategy for aligning the EEG distributions of different subjects before training and inference processes. The results of this study show that deep learning models with online pre-alignment strategies could significantly improve the generalization ability across datasets without any additional calibration data.
更多查看译文
关键词
brain-computer interface,cross-subject variability,cross-dataset variability,deep learning,transfer learning,EEG
AI 理解论文
溯源树
样例
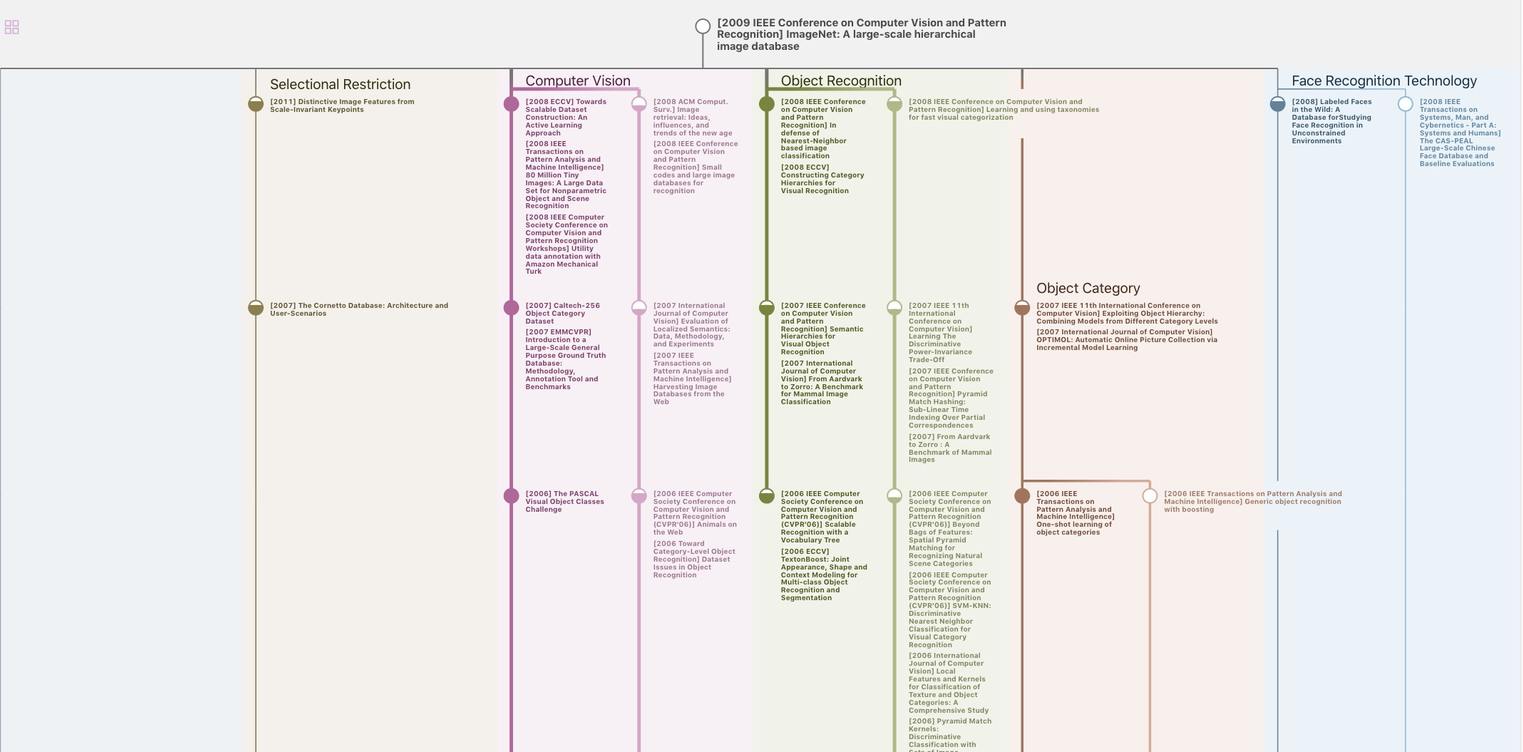
生成溯源树,研究论文发展脉络
Chat Paper
正在生成论文摘要