Sparsity-Based Naive Bayes Approach for Anomaly Detection in Real Surveillance Videos.
IEEE Conference Proceedings(2019)
摘要
Anomaly detection in video surveillance data is a challenging tasks due to the dynamic environment of the surveillance area. Multiple solutions have been proposed to tackle this problem ranging from using handcrafted features to end-to-end deep leaning methods. This paper proposes a model-based approach to anomaly detection for surveillance video data. This solution is based on sparsity estimation of pre-trained deep features. We aim to harvest the sparsity information of C3D deep features and use it as an additional information to differentiate the normal and anomalous event in a video. The proposed approach uses the naïve Bayes model within multiple instance learning framework. Experimental results shows that using sparsity information of C3D deep features could improve naive Bayes approach and enhance the accuracy of the decision boundary.
更多查看译文
关键词
anomaly detection,video surveillance data,model-based approach,sparsity estimation,multiple instance learning framework,sparsity-based Naive Bayes approach,C3D deep features,deep learning
AI 理解论文
溯源树
样例
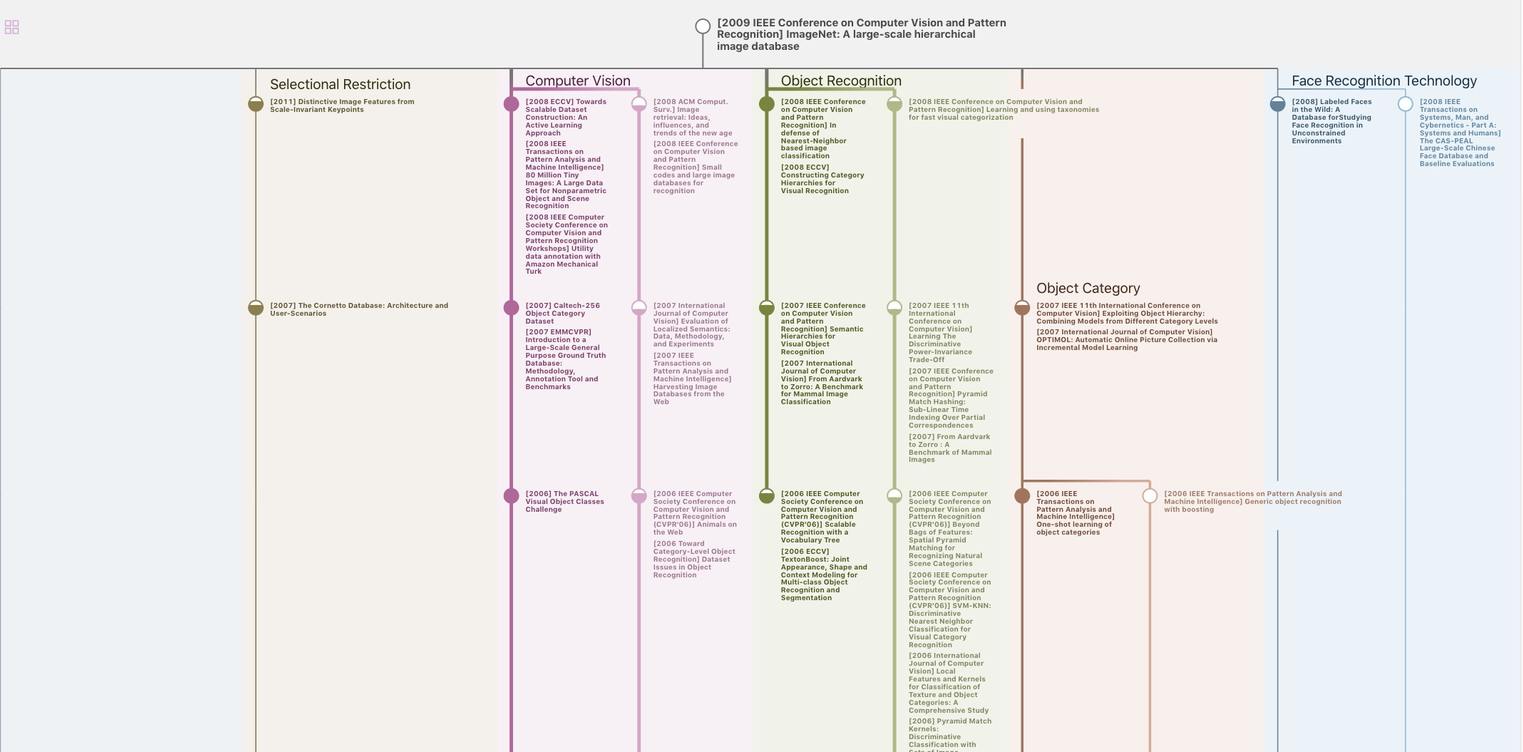
生成溯源树,研究论文发展脉络
Chat Paper
正在生成论文摘要