Face Recognition - A One-Shot Learning Perspective
2019 15th International Conference on Signal-Image Technology & Internet-Based Systems (SITIS)(2019)
摘要
Ability to learn from a single instance is something unique to the human species and One-shot learning algorithms try to mimic this special capability. On the other hand, despite the fantastic performance of Deep Learning-based methods on various image classification problems, performance often depends having on a huge number of annotated training samples per class. This fact is certainly a hindrance in deploying deep neural network-based systems in many real-life applications like face recognition. Furthermore, an addition of a new class to the system will require the need to re-train the whole system from scratch. Nevertheless, the prowess of deep learned features could also not be ignored. This research aims to combine the best of deep learned features with a traditional One-Shot learning framework. Results obtained on 2 publicly available datasets are very encouraging achieving over 90% accuracy on 5-way One-Shot tasks, and 84% on 50-way One-Shot problems.
更多查看译文
关键词
One-Shot Learning, Face recognition, Siamese Networks, Image Classification.
AI 理解论文
溯源树
样例
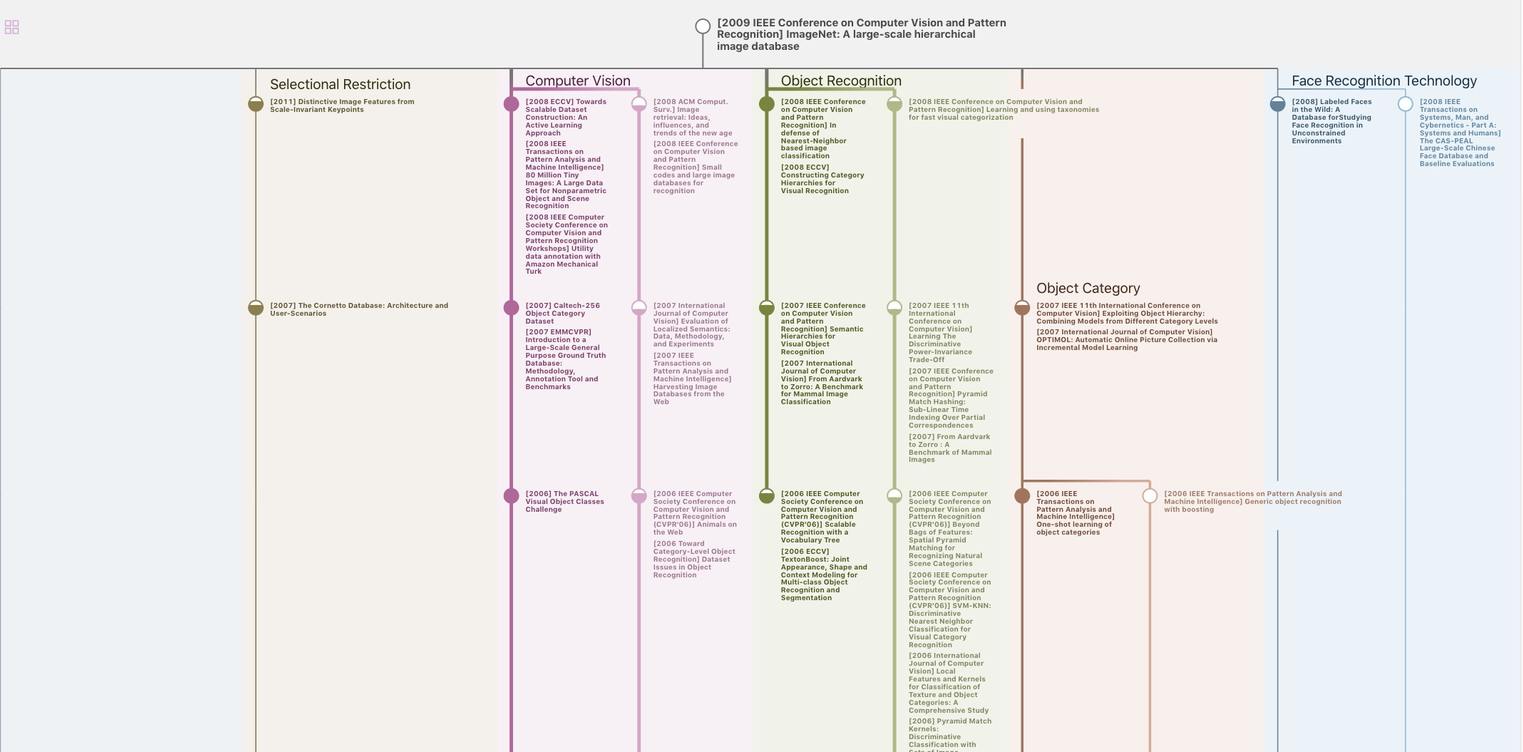
生成溯源树,研究论文发展脉络
Chat Paper
正在生成论文摘要