Insider threat detection using supervised machine learning algorithms
Telecommunication Systems(2023)
摘要
Insider threats refer to abnormal actions taken by individuals with privileged access, compromising system data’s confidentiality, integrity, and availability. They pose significant cybersecurity risks, leading to substantial losses for several organizations. Detecting insider threats is crucial due to the imbalance in their datasets. Moreover, the performance of existing works has been evaluated on various datasets and problem settings, making it challenging to compare the effectiveness of different algorithms and offer recommendations to decision-makers. Furthermore, no existing work investigates the impact of changing hyperparameters. This paper aims to objectively assess the performance of various supervised machine learning algorithms for detecting insider threats under the same setting. We precisely evaluate the performance of various supervised machine learning algorithms on a balanced dataset using the same feature extraction method. Additionally, we explore the impact of hyperparameter tuning on performance within the balanced dataset. Finally, we investigate the performance of different algorithms in the context of imbalanced datasets under various conditions. We conduct all the experiments in the publicly available CERT r4.2 dataset. The results show that supervised learning with a balanced dataset in RF obtains the best accuracy and F1-score of 95.9% compared with existing works, such as, DNN, LSTM Autoencoder and User Behavior Analysis.
更多查看译文
关键词
Cybersecurity,Insider threat,Imbalanced dataset,Supervised learning
AI 理解论文
溯源树
样例
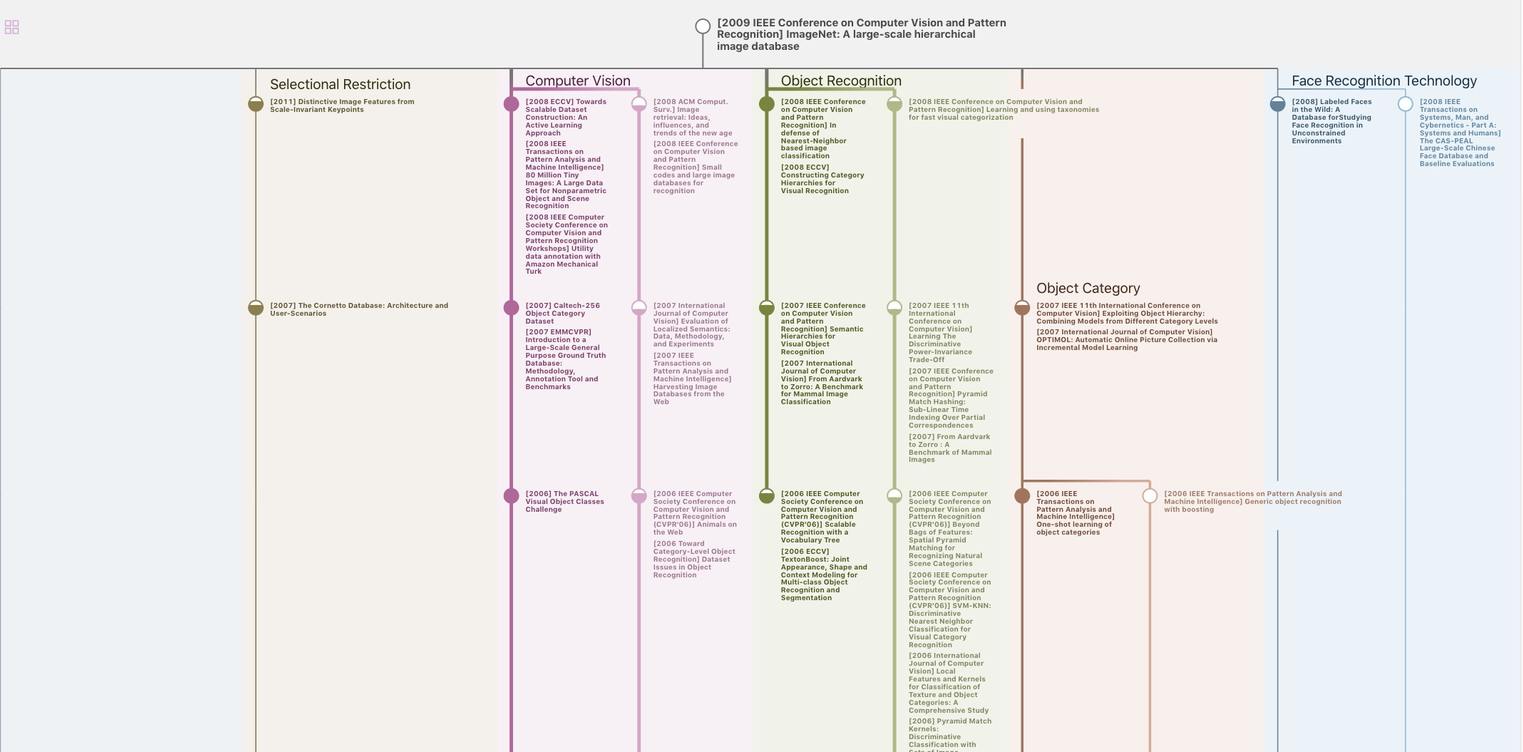
生成溯源树,研究论文发展脉络
Chat Paper
正在生成论文摘要