Artificial Neural Network Inspired By Neuroimaging Connectivity: Application In Autism Spectrum Disorder
2020 IEEE INTERNATIONAL CONFERENCE ON BIG DATA AND SMART COMPUTING (BIGCOMP 2020)(2020)
摘要
Distinguishing the autism spectrum disorder (ASD) from typical control (TC) using resting-state functional magnetic resonance imaging (rs-fMRI) is very difficult because ASD has heterogenetic properties and induce small changes in the brain structure. Moreover, distinguishing ASD from TC using the data obtained from many sites is even more difficult because many factors might negatively affect the classification model leading to unstable results. This difficulty is especially true for existing rs-fMRI analysis methods such as functional connectivity analysis. Recent studies have shown better ASD classification performance using models constructed using recurrent neural network (RNN). However, a blinded application of RNN not considering the multi-site factors is sub-optimal. In this paper, we present an artificial neural network model inspired by the existing functional connectivity analysis modeling. Our model includes layers that play the role of spatial reduction, temporal feature extraction, and combining phenotypic data (inclusive of multi-site data) for classifying ASD. We applied the cross-validation framework to the multi-site rs-fMRI dataset to test the proposed model. Our best model showed an accuracy of 74.54%, which is superior to the existing functional connectivity analysis with an accuracy of 54.05%.
更多查看译文
关键词
Autism Spectrum Disorders, Classification, Neural Networks, Resting-state fMRI
AI 理解论文
溯源树
样例
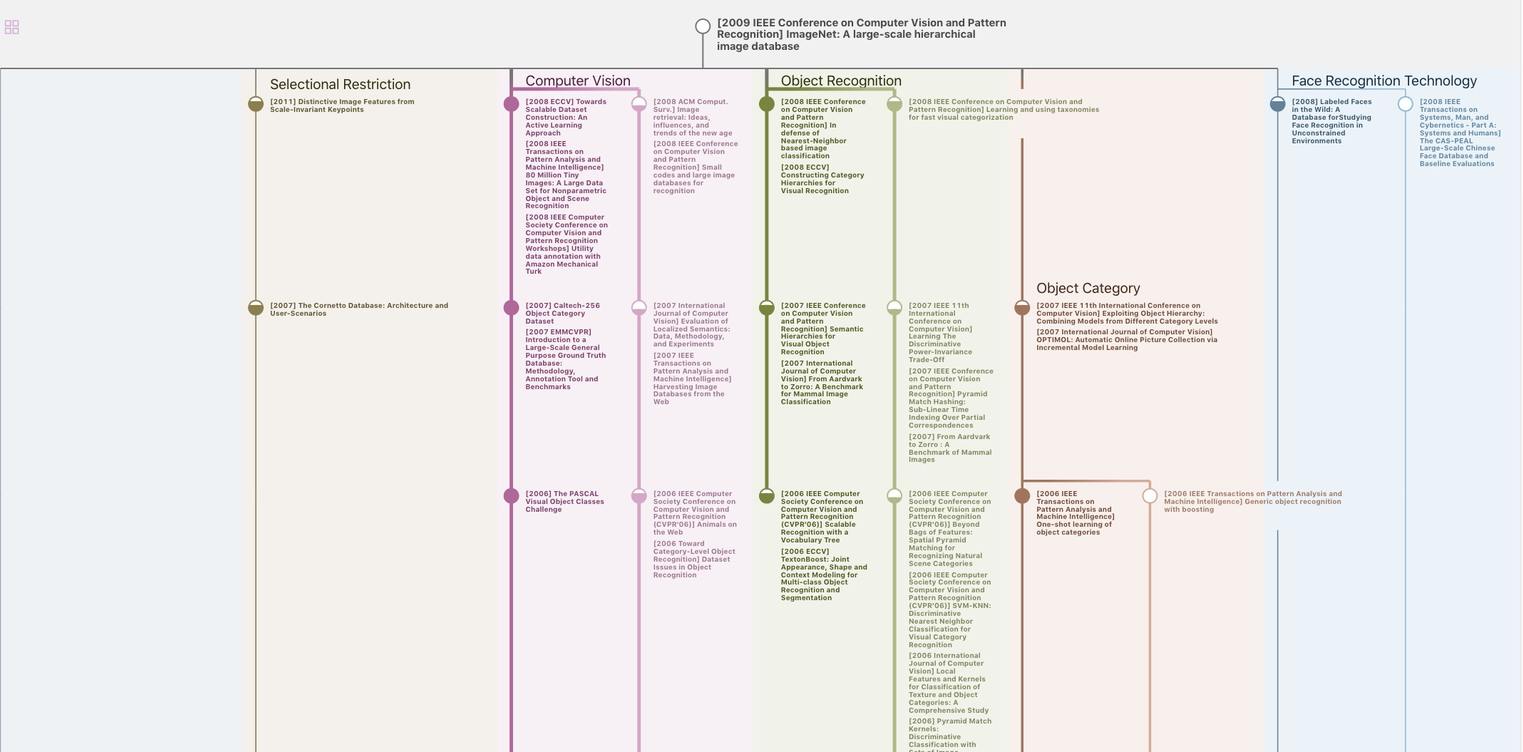
生成溯源树,研究论文发展脉络
Chat Paper
正在生成论文摘要