Sequence-Aware Factored Mixed Similarity Model for Next-Item Recommendation
2020 IEEE International Conference on Big Data and Smart Computing (BigComp)(2020)
摘要
Sequence-aware next-item recommendation has recently been studied because of the noteworthy usefulness of the sequential information integrated into recommendation algorithms. Following the development thread of sequential recommendation methods, especially the factored Markov chains segment, we propose a novel sequence-aware factored mixed similarity model (S-FMSM) based on a well-designed fusing similarity model with factored high-order Markov chains (i.e., Fossil). Unlike the baseline methods that model the general representation and the sequential representation in two divided factorization components, we use a factored mixed similarity model that unites the general similarity and the sequential relationship between two successive items for their sequential representation learning. Experiments on six datasets show that our newly introduced general similarity can notably improve the results of the recommended ranking lists. And a study on tuning the prior tradeoff parameter indicates the importance of the general similarity on different datasets.
更多查看译文
关键词
sequential recommendation, factored Markov chains, factored mixed similarity model.
AI 理解论文
溯源树
样例
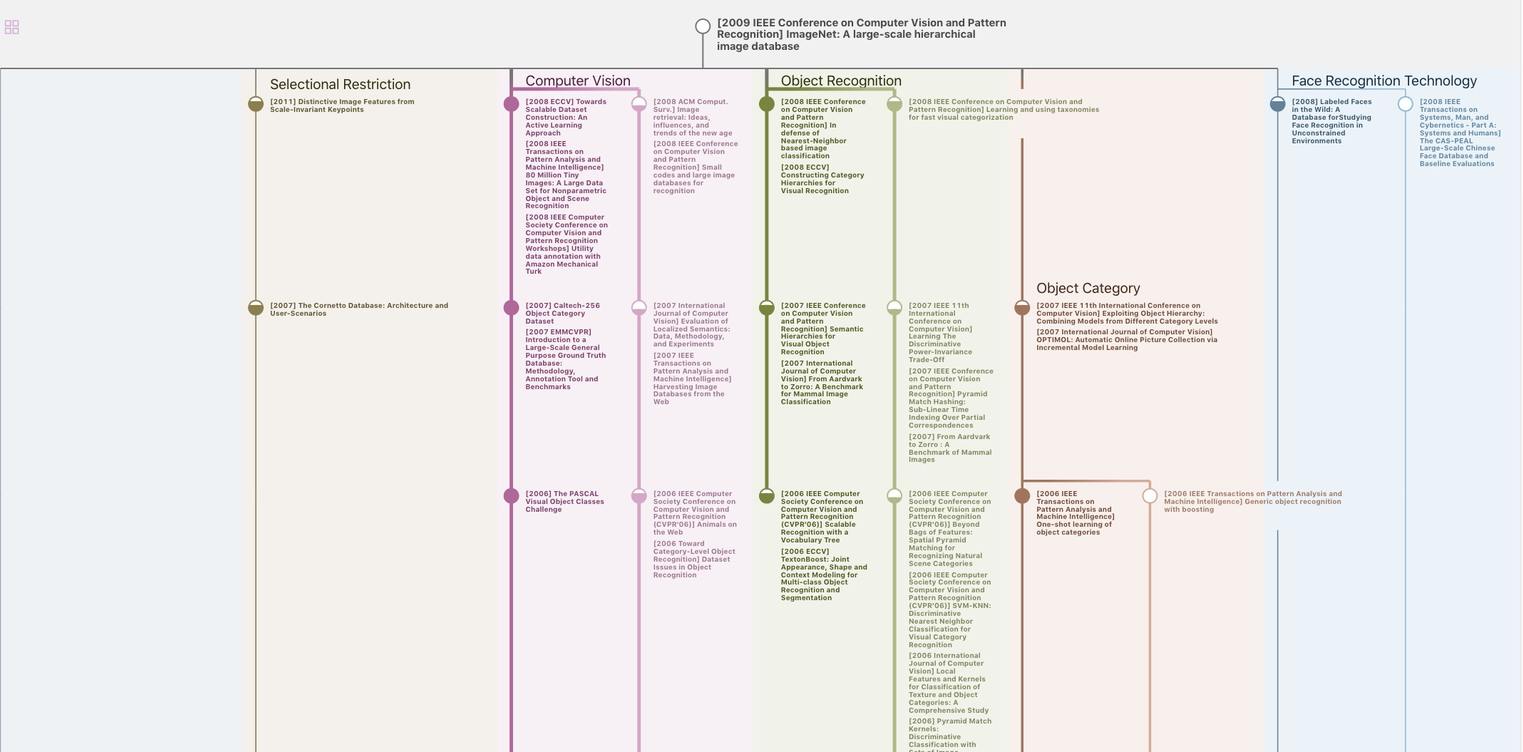
生成溯源树,研究论文发展脉络
Chat Paper
正在生成论文摘要