Automatic Segmentation Of Multiple Cardiovascular Structures From Cardiac Computed Tomography Angiography Images Using Deep Learning
PLOS ONE(2020)
摘要
BackgroundSegmentation of cardiovascular images is resource-intensive. We design an automated deep learning method for the segmentation of multiple structures from Coronary Computed Tomography Angiography (CCTA) images.MethodsImages from a multicenter registry of patients that underwent clinically-indicated CCTA were used. The proximal ascending and descending aorta (PAA, DA), superior and inferior vena cavae (SVC, IVC), pulmonary artery (PA), coronary sinus (CS), right ventricular wall (RVW) and left atrial wall (LAW) were annotated as ground truth. The U-net-derived deep learning model was trained, validated and tested in a 70:20:10 split.ResultsThe dataset comprised 206 patients, with 5.130 billion pixels. Mean age was 59.9 +/- 9.4 yrs., and was 42.7% female. An overall median Dice score of 0.820 (0.782, 0.843) was achieved. Median Dice scores for PAA, DA, SVC, IVC, PA, CS, RVW and LAW were 0.969 (0.979, 0.988), 0.953 (0.955, 0.983), 0.937 (0.934, 0.965), 0.903 (0.897, 0.948), 0.775 (0.724, 0.925), 0.720 (0.642, 0.809), 0.685 (0.631, 0.761) and 0.625 (0.596, 0.749) respectively. Apart from the CS, there were no significant differences in performance between sexes or age groups.ConclusionsAn automated deep learning model demonstrated segmentation of multiple cardiovascular structures from CCTA images with reasonable overall accuracy when evaluated on a pixel level.
更多查看译文
AI 理解论文
溯源树
样例
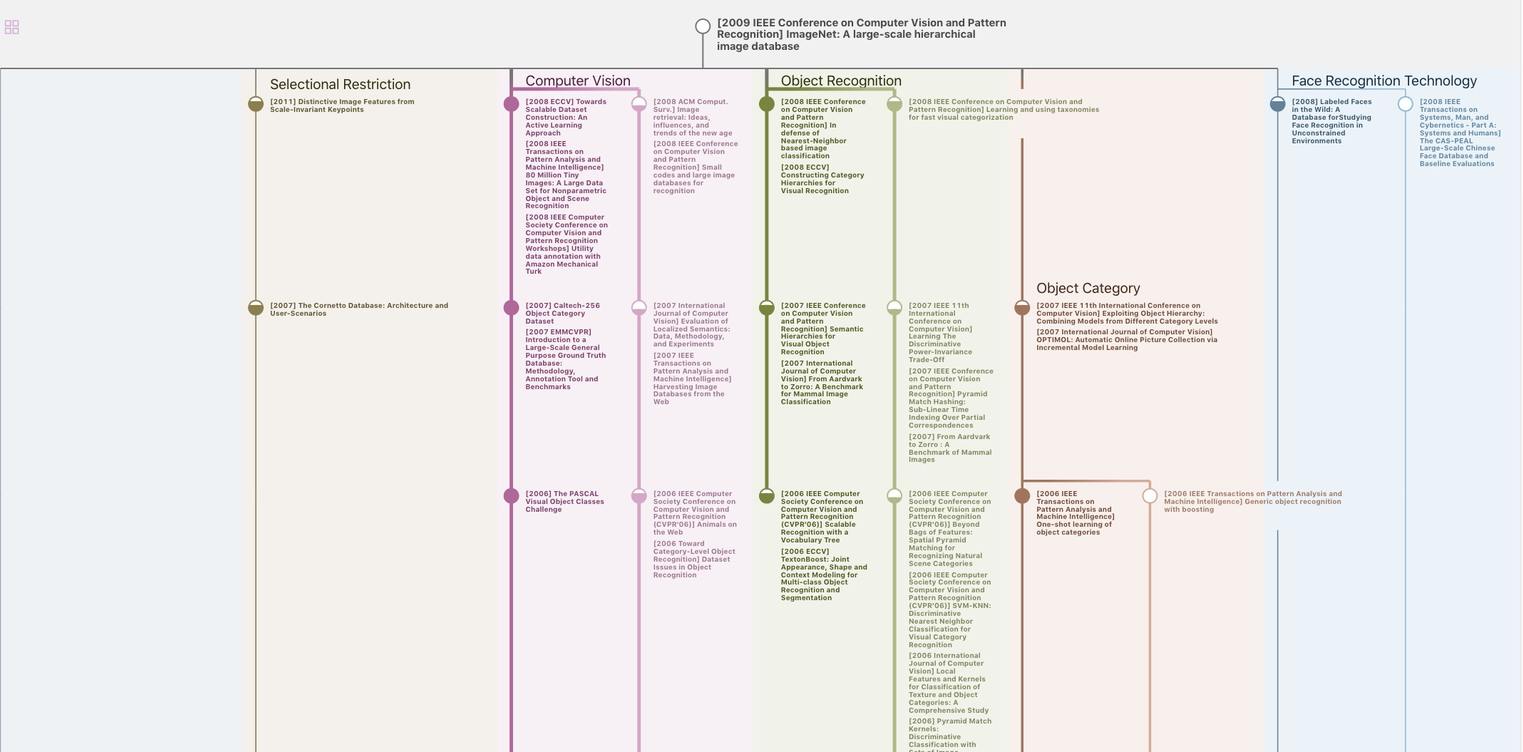
生成溯源树,研究论文发展脉络
Chat Paper
正在生成论文摘要