Adaptive Dialog Policy Learning with Hindsight and User Modeling
SIGdial(2020)
摘要
Reinforcement learning methods have been used to compute dialog policies from language-based interaction experiences. Efficiency is of particular importance in dialog policy learning, because of the considerable cost of interacting with people, and the very poor user experience from low-quality conversations. Aiming at improving the efficiency of dialog policy learning, we develop algorithm LHUA (Learning with Hindsight, User modeling, and Adaptation) that, for the first time, enables dialog agents to adaptively learn with hindsight from both simulated and real users. Simulation and hindsight provide the dialog agent with more experience and more (positive) reinforcements respectively. Experimental results suggest that, in success rate and policy quality, LHUA outperforms competitive baselines from the literature, including its no-simulation, no-adaptation, and no-hindsight counterparts.
更多查看译文
关键词
adaptive,policy,learning,hindsight
AI 理解论文
溯源树
样例
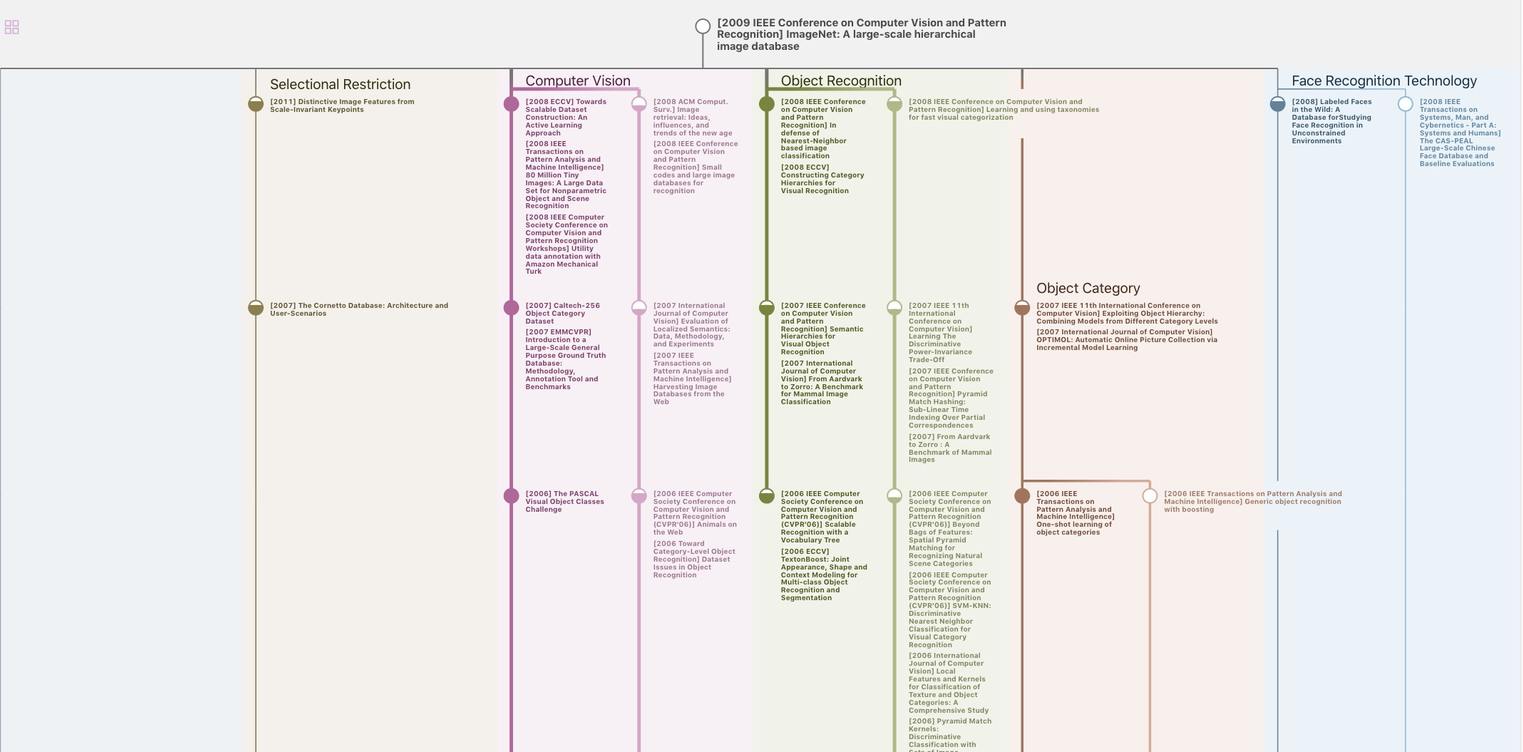
生成溯源树,研究论文发展脉络
Chat Paper
正在生成论文摘要