A Dynamical Mean-Field Theory for Learning in Restricted Boltzmann Machines
JOURNAL OF STATISTICAL MECHANICS-THEORY AND EXPERIMENT(2020)
摘要
We define a message-passing algorithm for computing magnetizations in restricted Boltzmann machines, which are Ising models on bipartite graphs introduced as neural network models for probability distributions over spin configurations. To model nontrivial statistical dependencies between the spins' couplings, we assume that the rectangular coupling matrix is drawn from an arbitrary bi-rotation invariant random matrix ensemble. Using the dynamical functional method of statistical mechanics we exactly analyze the dynamics of the algorithm in the large system limit. We prove the global convergence of the algorithm under a stability criterion and compute asymptotic convergence rates showing excellent agreement with numerical simulations.
更多查看译文
关键词
cavity and replica method,machine learning,message-passing algorithms,spin glasses
AI 理解论文
溯源树
样例
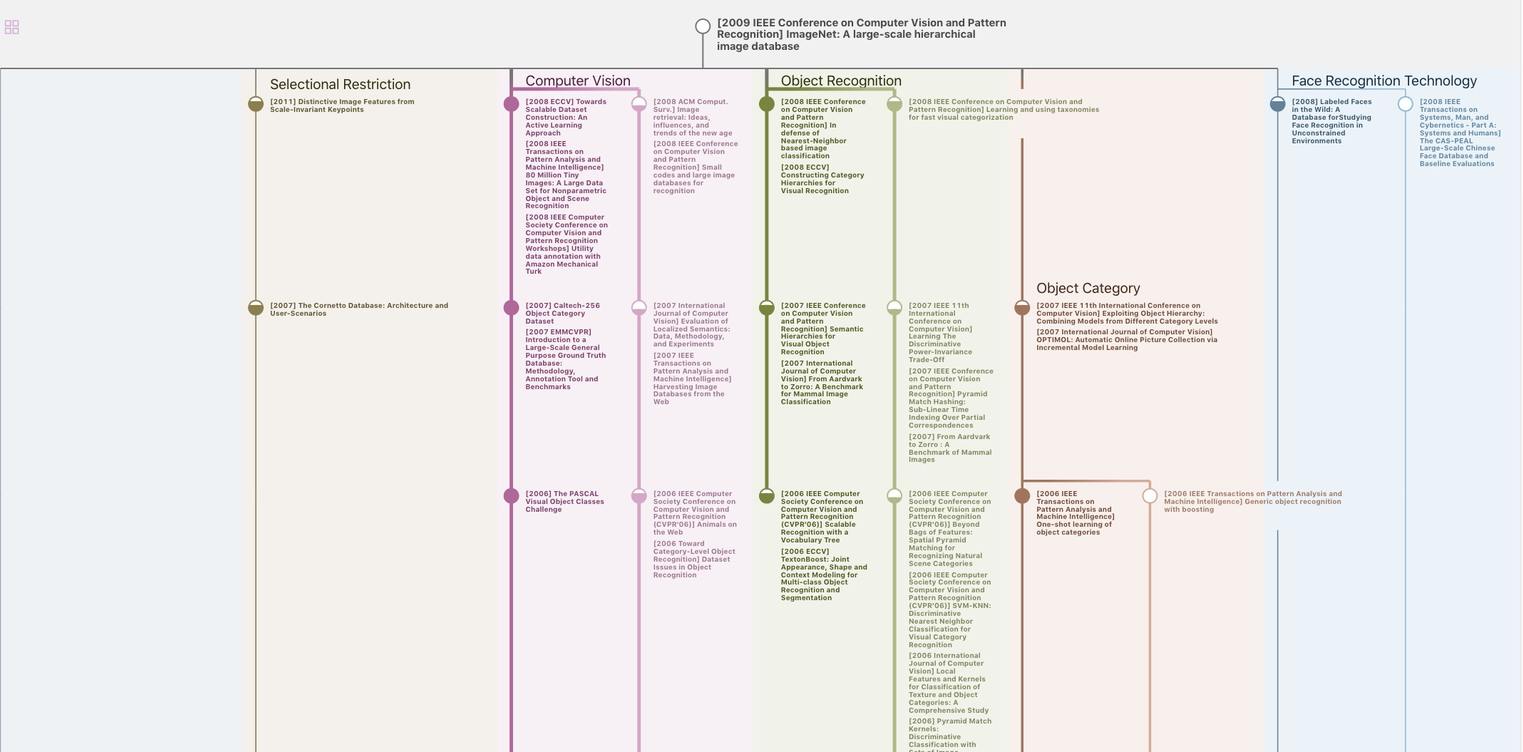
生成溯源树,研究论文发展脉络
Chat Paper
正在生成论文摘要