Log-regularly varying scale mixture of normals for robust regression
COMPUTATIONAL STATISTICS & DATA ANALYSIS(2022)
摘要
Linear regression that employs the assumption of normality for the error distribution may lead to an undesirable posterior inference of regression coefficients due to potential outliers. A finite mixture of two components, one with thin and one with heavy tails, is considered as the error distribution in this study. For the heavily-tailed component, the novel class of distributions is introduced; their densities are log-regularly varying and have heavier tails than the Cauchy distribution. Yet, they are expressed as a scale mixture of normals which enables the efficient posterior inference when using a Gibbs sampler. The robustness of the posterior distributions is proved under the proposed models using a minimal set of assumptions, which justifies the use of shrinkage priors with unbounded densities for the coefficient vector in the presence of outliers. An extensive comparison with the existing methods via simulation study shows the improved performance of the proposed model in point and interval estimation, as well as its computational efficiency. Further, the posterior robustness of the proposed method is confirmed in an empirical study with shrinkage priors for regression coefficients. (c) 2022 Elsevier B.V. All rights reserved.
更多查看译文
关键词
Robust statistics, Linear regression, Heavily -tailed distribution, Scale mixture of normals, Log -regularly varying density, Gibbs sampler
AI 理解论文
溯源树
样例
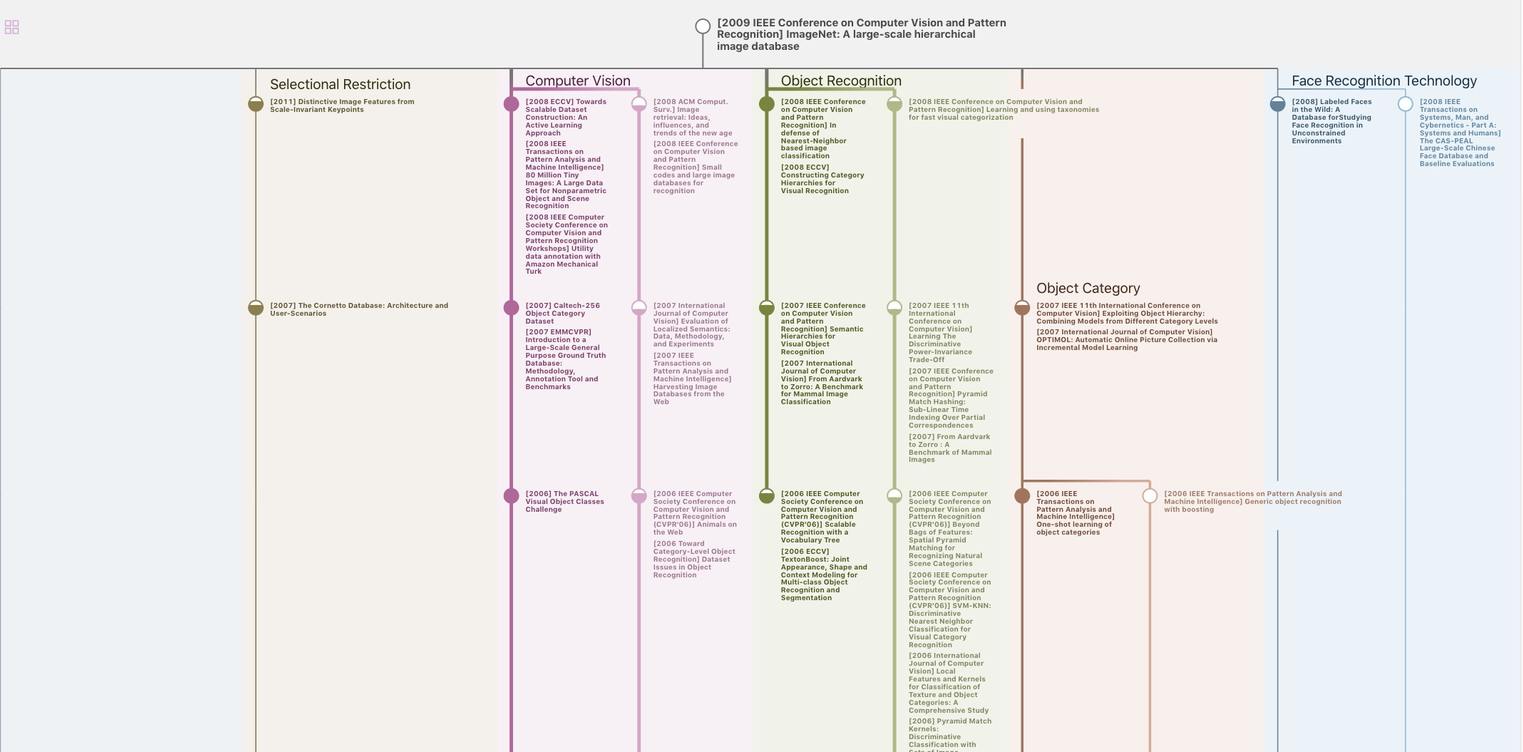
生成溯源树,研究论文发展脉络
Chat Paper
正在生成论文摘要