Learning From Synthetic Images Via Active Pseudo-Labeling
IEEE TRANSACTIONS ON IMAGE PROCESSING(2020)
摘要
Synthetic visual data refers to the data automatically rendered by the mature computer graphic algorithms. With the rapid development of these techniques, we can now collect photo-realistic synthetic images with accurate pixel-level annotations without much effort. However, due to the domain gaps between synthetic data and real data, in terms of not only visual appearance but also label distribution, directly applying models trained on synthetic images to real ones can hardly yield satisfactory performance. Since the collection of accurate labels for real images is very laborious and time-consuming, developing algorithms which can learn from synthetic images is of great significance. In this paper, we propose a novel framework, namely Active Pseudo-Labeling (APL), to reduce the domain gaps between synthetic images and real images. In APL framework, we first predict pseudo-labels for the unlabeled real images in the target domain by actively adapting the style of the real images to source domain. Specifically, the style of real images is adjusted via a novel task guided generative model, and then pseudo-labels are predicted for these actively adapted images. Lastly, we fine-tune the source-trained model in the pseudo-labeled target domain, which helps to fit the distribution of the real data. Experiments on both semantic segmentation and object detection tasks with several challenging benchmark data sets demonstrate the priority of our proposed method compared to the existing state-of-the-art approaches.
更多查看译文
关键词
Task analysis, Data models, Training, Visualization, Adaptation models, Neural networks, Predictive models, Deep learning, domain adaptation, style transfer, pseudo-labeling, semantic segmentation, object detection
AI 理解论文
溯源树
样例
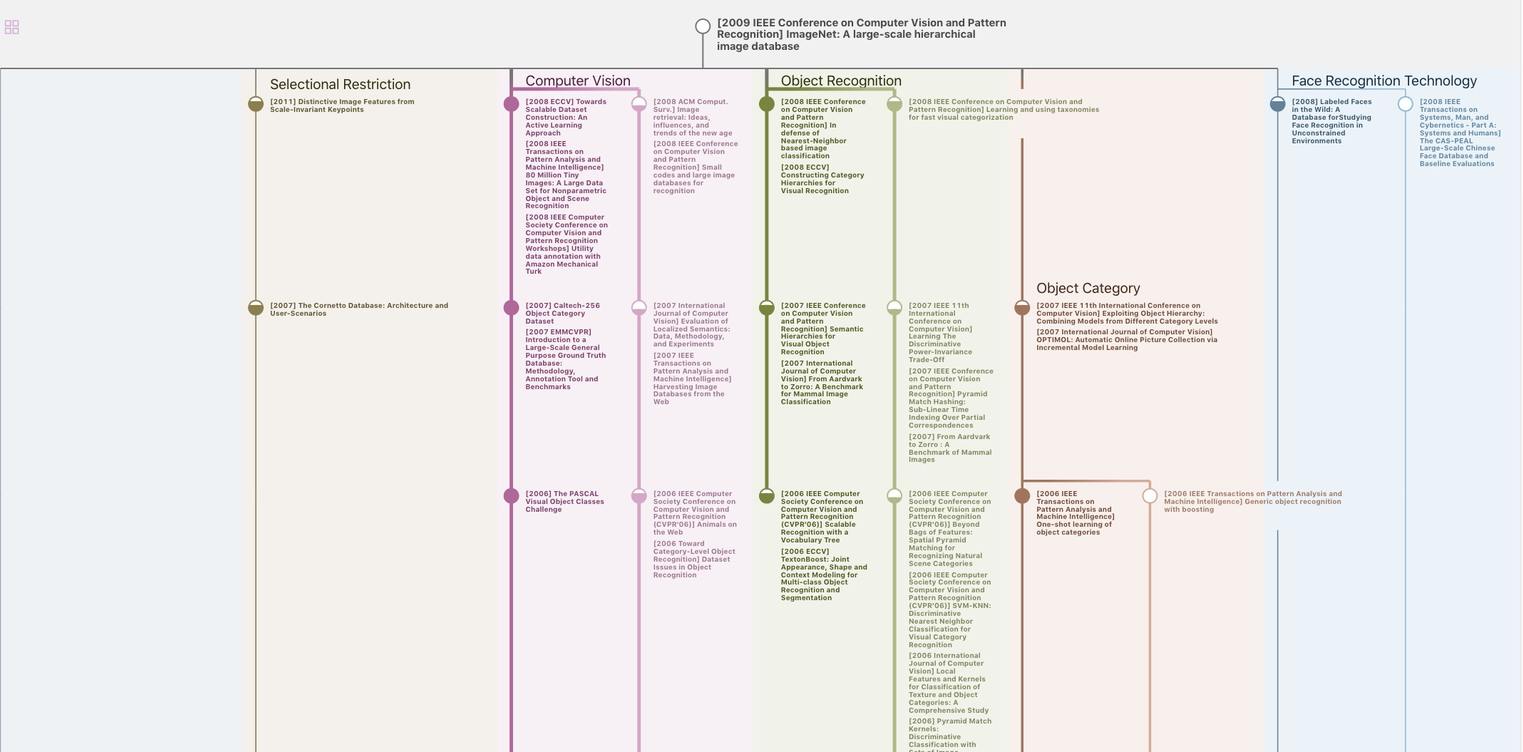
生成溯源树,研究论文发展脉络
Chat Paper
正在生成论文摘要