A Systematic Assessment Of Syntactic Generalization In Neural Language Models
58TH ANNUAL MEETING OF THE ASSOCIATION FOR COMPUTATIONAL LINGUISTICS (ACL 2020)(2020)
摘要
While state-of-the-art neural network models continue to achieve lower perplexity scores on language modeling benchmarks, it remains unknown whether optimizing for broad-coverage predictive performance leads to human-like syntactic knowledge. Furthermore, existing work has not provided a clear picture about the model properties required to produce proper syntactic generalizations. We present a systematic evaluation of the syntactic knowledge of neural language models, testing 20 combinations of model types and data sizes on a set of 34 English-language syntactic test suites. We find substantial differences in syntactic generalization performance by model architecture, with sequential models underperforming other architectures. Factorially manipulating model architecture and training dataset size (1M-40M words), we find that variability in syntactic generalization performance is substantially greater by architecture than by dataset size for the corpora tested in our experiments. Our results also reveal a dissociation between perplexity and syntactic generalization performance.
更多查看译文
关键词
syntactic generalization,neural language
AI 理解论文
溯源树
样例
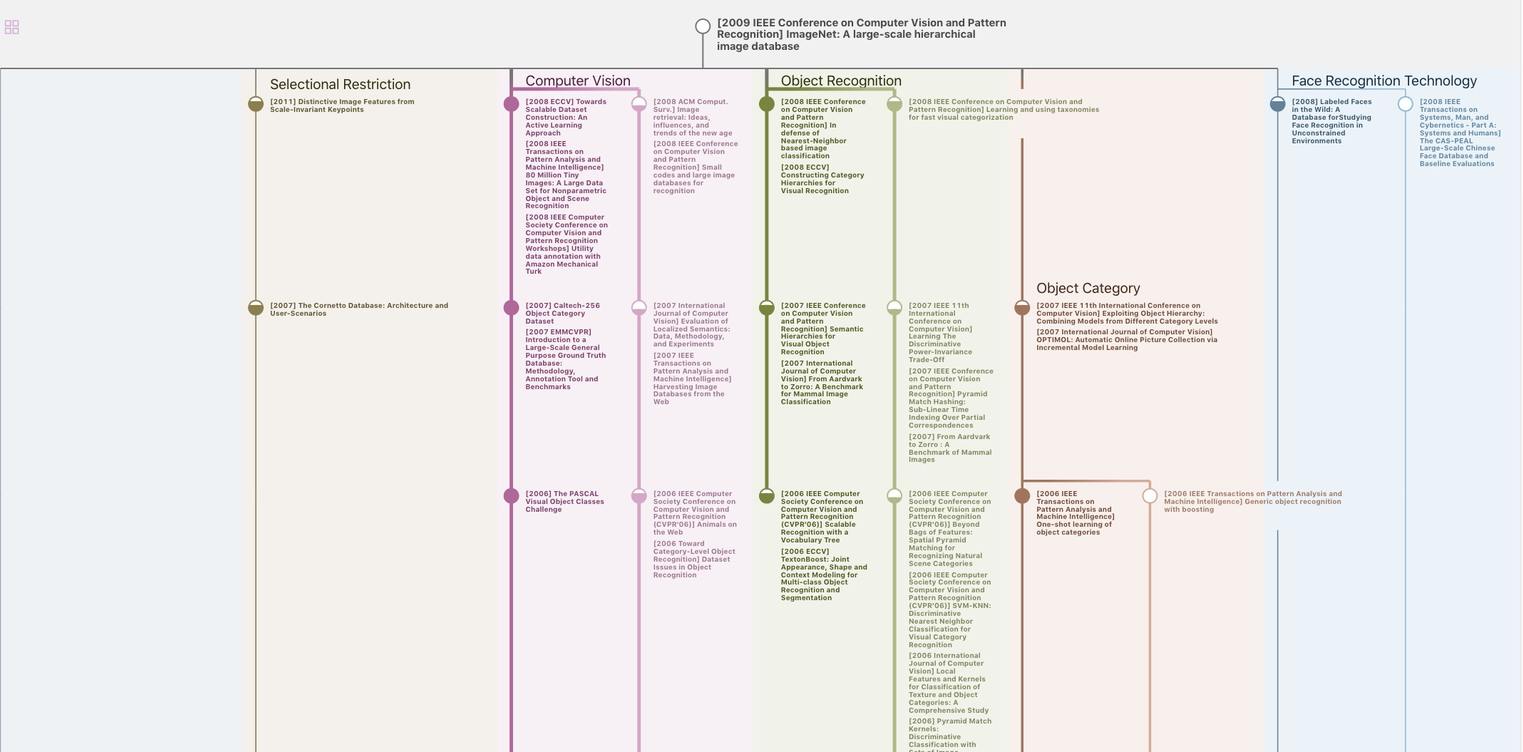
生成溯源树,研究论文发展脉络
Chat Paper
正在生成论文摘要