Supert: Towards New Frontiers In Unsupervised Evaluation Metrics For Multi-Document Summarization
58TH ANNUAL MEETING OF THE ASSOCIATION FOR COMPUTATIONAL LINGUISTICS (ACL 2020)(2020)
摘要
We study unsupervised multi-document summarization evaluation metrics, which require neither human-written reference summaries nor human annotations (e.g. preferences, ratings, etc.). We propose SUPERT, which rates the quality of a summary by measuring its semantic similarity with a pseudo reference summary, i.e. selected salient sentences from the source documents, using contextualized embeddings and soft token alignment techniques. Compared to the state-of-the-art unsupervised evaluation metrics, SUPERT correlates better with human ratings by 1839%. Furthermore, we use SUPERT as rewards to guide a neural-based reinforcement learning summarizer, yielding favorable performance compared to the state-of-the-art unsupervised summarizers. All source code is available at https://github.com/yg211/acl20-ref-free-eval.
更多查看译文
关键词
unsupervised evaluation metrics,supert,multi-document
AI 理解论文
溯源树
样例
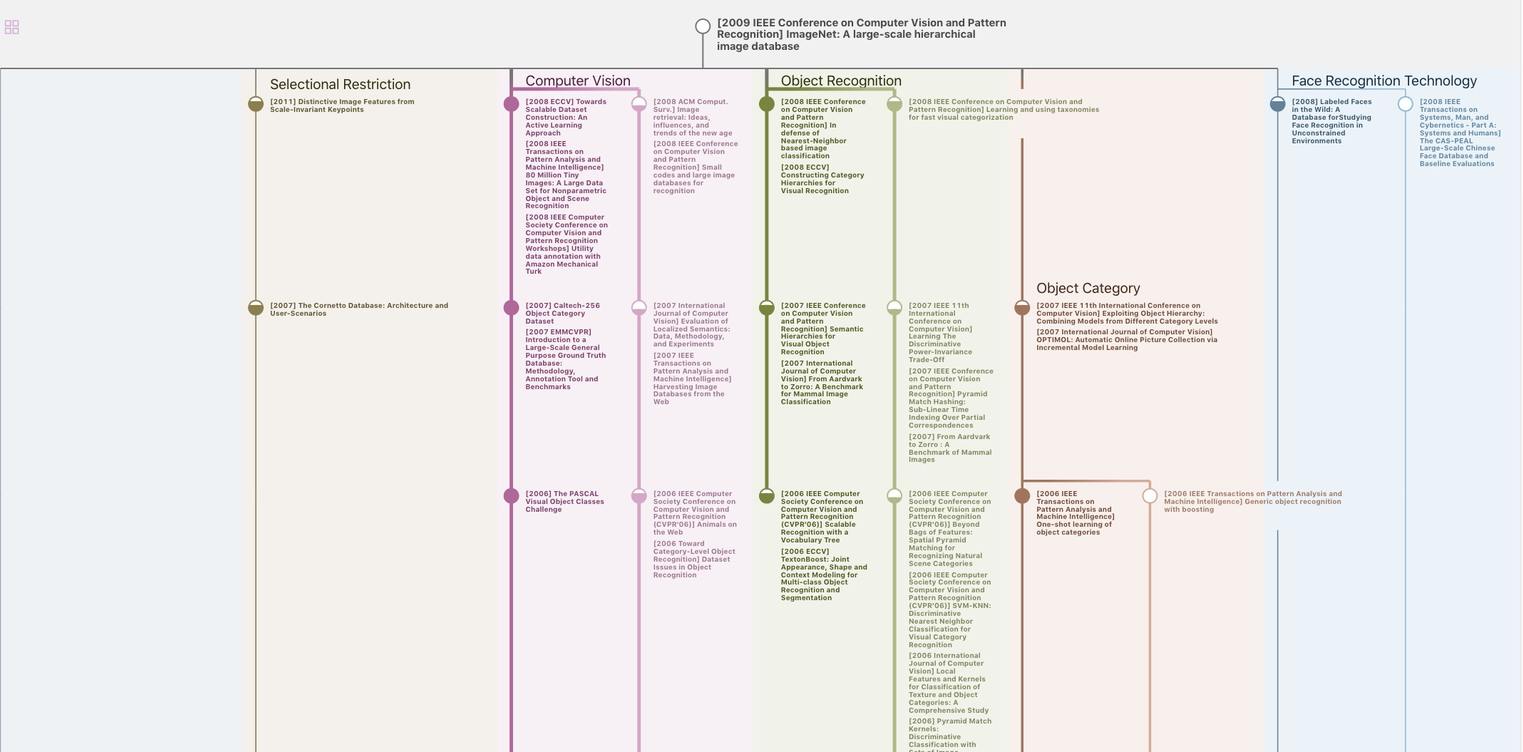
生成溯源树,研究论文发展脉络
Chat Paper
正在生成论文摘要