Relatedness measures to aid the transfer of building blocks among multiple tasks
Genetic and Evolutionary Computation Conference(2020)
摘要
ABSTRACTMultitask Learning is a learning paradigm that deals with multiple different tasks in parallel and transfers knowledge among them. XOF, a Learning Classifier System using tree-based programs to encode building blocks (meta-features), constructs and collects features with rich discriminative information for classification tasks in an Observed List. This paper seeks to facilitate the automation of feature transferring in between tasks by utilising the Observed List. We hypothesise that the best discriminative features of a classification task carry its characteristics. Therefore, the relatedness between any two tasks can be estimated by comparing their most appropriate patterns. We propose a multiple-XOF system, called mXOF, that can dynamically adapt feature transfer among XOFs. This system utilises the Observed List to estimate the task relatedness. This method enables the automation of transferring features. In terms of knowledge discovery, the resemblance estimation provides insightful relations among multiple data. We experimented mXOF on various scenarios, e.g. representative Hierarchical Boolean problems, classification of distinct classes in the UCI Zoo dataset, and unrelated tasks, to validate its abilities of automatic knowledgetransfer and estimating task relatedness. Results show that mXOF can estimate the relatedness reasonably between multiple tasks to aid the learning performance with the dynamic feature transferring.
更多查看译文
关键词
LCS, XCS, Code Fragments, XOF
AI 理解论文
溯源树
样例
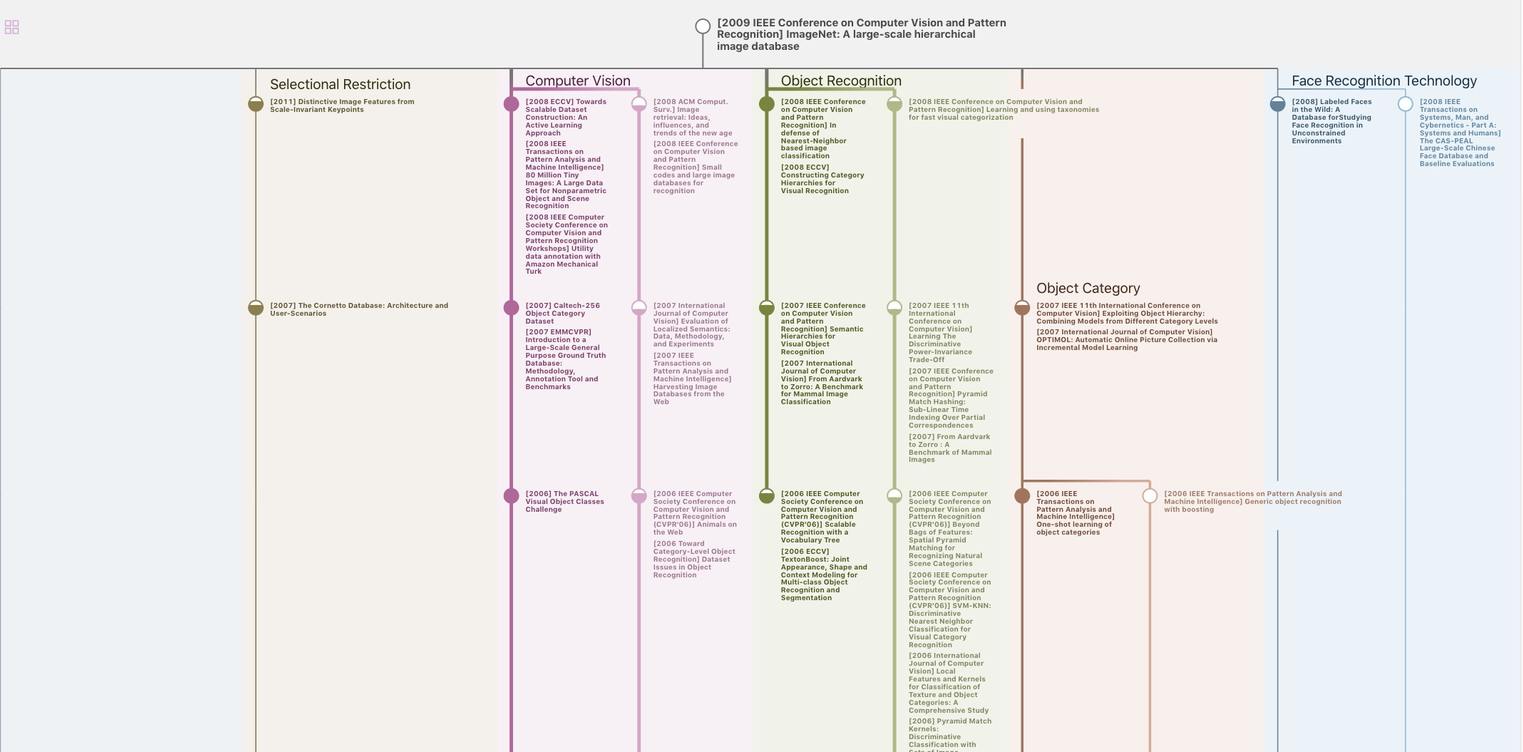
生成溯源树,研究论文发展脉络
Chat Paper
正在生成论文摘要