Progressive Adversarial Semantic Segmentation
2020 25TH INTERNATIONAL CONFERENCE ON PATTERN RECOGNITION (ICPR)(2021)
摘要
Medical image computing has advanced rapidly with the advent of deep learning techniques. Deep convolutional neural networks can perform well given full supervision. However, the success of such fully-supervised models in various image analysis tasks (e.g., anatomy or lesion segmentation from medical images) depends on the availability of massive quantities of labeled data. Given small sample sizes, such models are prohibitively data biased with large domain shifts. To tackle this problem, we propose a novel end-to-end medical image segmentation model, namely Progressive Adversarial Semantic Segmentation (PASS), which can make improved and consistent pixel-wise segmentation predictions without requiring any domain-specific data during training. Our extensive experimentation with 8 public diabetic retinopathy and chest X-ray datasets confirms the effectiveness of PASS in accurate vascular and pulmonary segmentation, both for in-domain and cross-domain evaluations.
更多查看译文
关键词
segmentation, adversarial learning, domain-shift, diabetic retinopathy, retinal vasculature, pulmonary X-ray
AI 理解论文
溯源树
样例
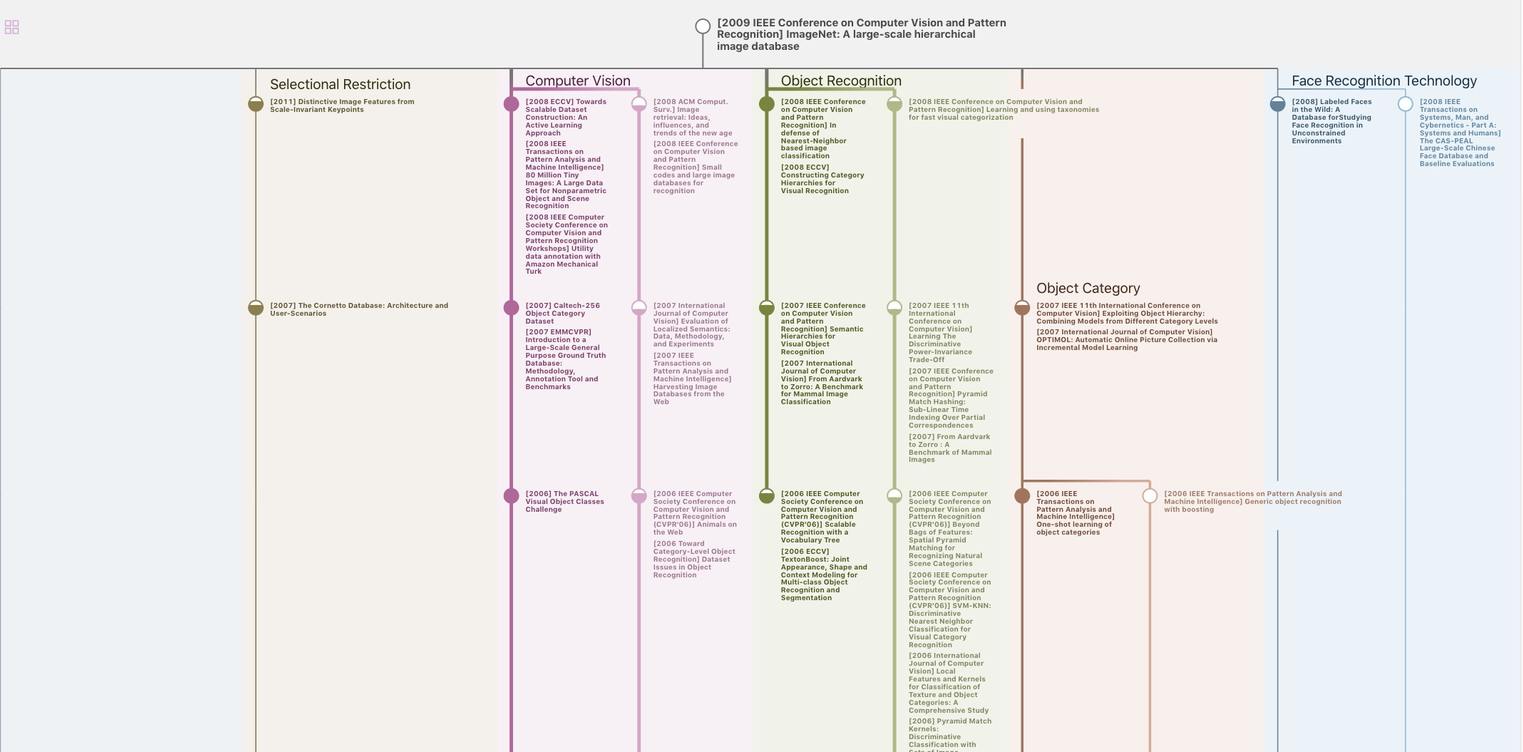
生成溯源树,研究论文发展脉络
Chat Paper
正在生成论文摘要