Affordance-based Generation of Pretend Object Interaction Variants for Human-Computer Improvisational Theater.
ICCC(2019)
摘要
This paper describes DeepIMAGINATION, a neural architecture to generate variants of movement-based object interactions with props using the physical attributes of props while playing the Props game. The agent can generate these action variants while searching a learned action space in real-time to provide improvised responses to its human partner. Convolutional and recurrent variants of CVAEs are used for experimentation. The paper presents an evaluation of the architecture by benchmarking its ability to learn the human data set and generate believable, recognizable, and high-quality action variants from it. Results showed that the agent could generate believable, high-quality action variants. but that recognizability requires improvement.
更多查看译文
AI 理解论文
溯源树
样例
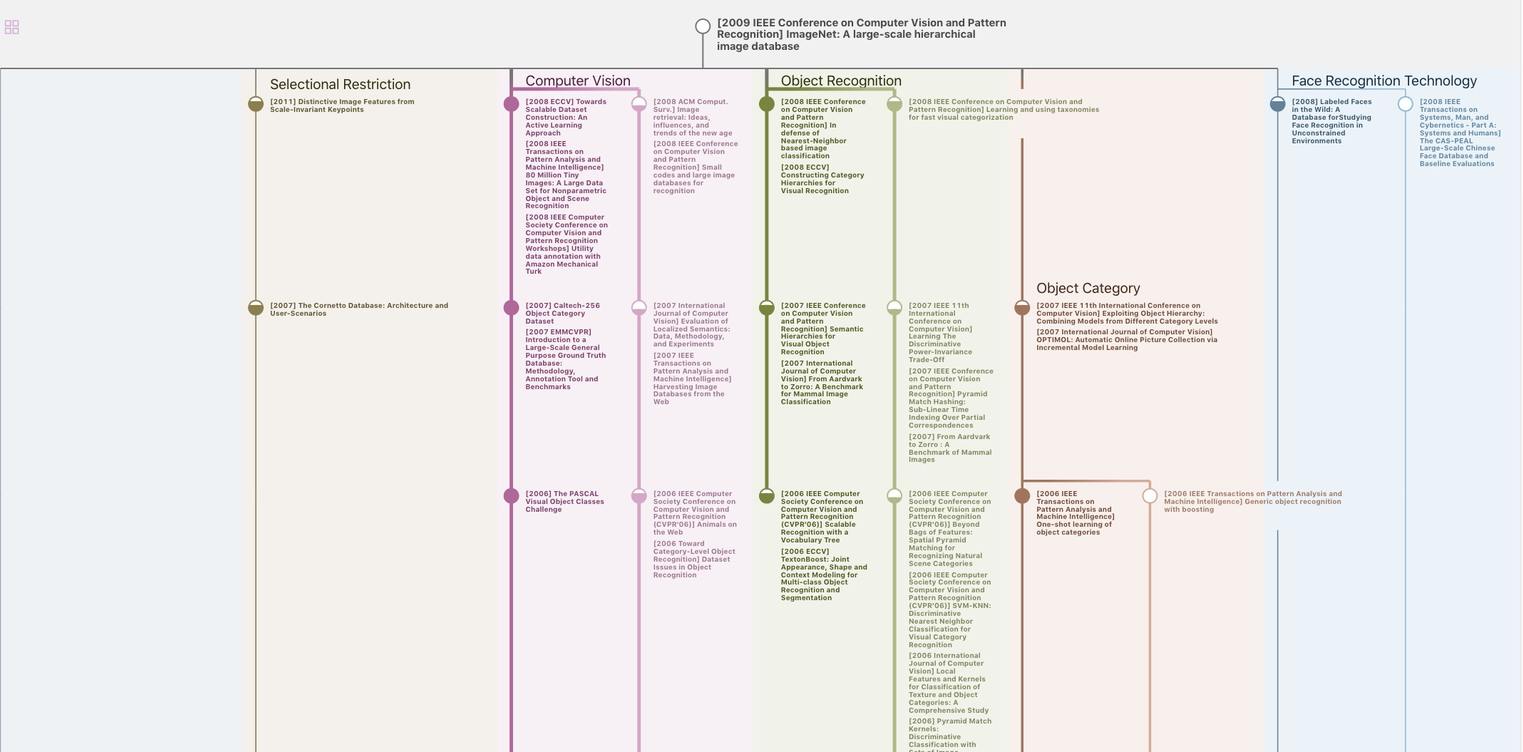
生成溯源树,研究论文发展脉络
Chat Paper
正在生成论文摘要