Towards Deployment of Robust Cooperative AI Agents: An Algorithmic Framework for Learning Adaptive Policies
AAMAS '19: International Conference on Autonomous Agents and Multiagent Systems Auckland New Zealand May, 2020(2020)
摘要
We study the problem of designing an AI agent that can robustly cooperate with agents of unknown type (i.e., previously unobserved behavior) in multi-agent scenarios. Our work is inspired by real-world applications in which an AI agent, e.g., a virtual assistant, has to cooperate with new types of agents/users after its deployment. We model this problem via parametric Markov Decision Processes where the parameters correspond to a user's type and characterize her behavior. In the test phase, the AI agent has to interact with a user of an unknown type. We develop an algorithmic framework for learning adaptive policies: our approach relies on observing the user's actions to make inferences about the user's type and adapting the policy to facilitate efficient cooperation. We show that without being adaptive, an AI agent can end up performing arbitrarily bad in the test phase. Using our framework, we propose two concrete algorithms for computing policies that automatically adapt to the user in the test phase. We demonstrate the effectiveness of our algorithms in a cooperative gathering game environment for two agents.
更多查看译文
AI 理解论文
溯源树
样例
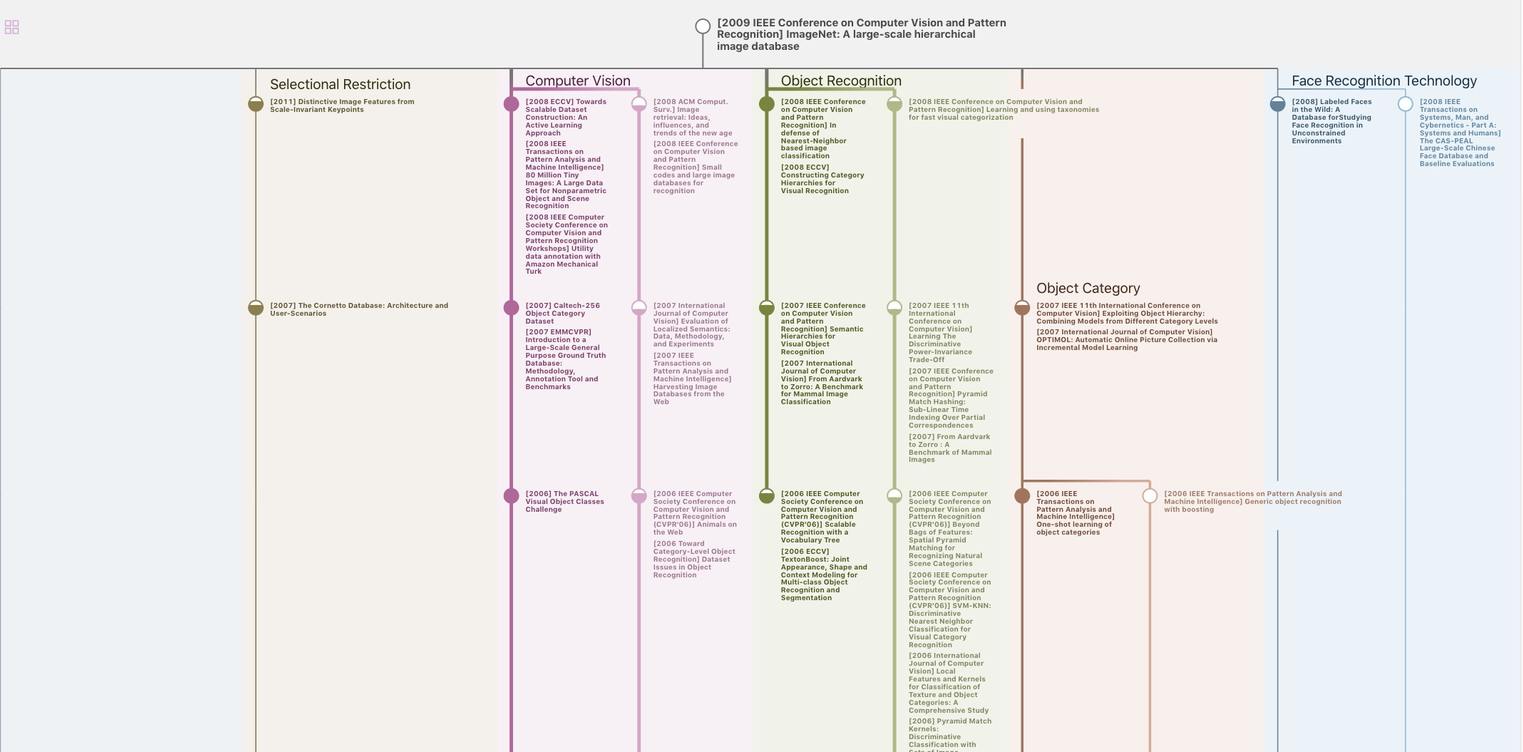
生成溯源树,研究论文发展脉络
Chat Paper
正在生成论文摘要