Candidate selections with proportional fairness constraints
AAMAS '19: International Conference on Autonomous Agents and Multiagent Systems Auckland New Zealand May, 2020(2021)
摘要
Selecting a subset of candidates with various attributes under fairness constraints has been attracting considerable attention from the AI community, with applications ranging from school admissions to committee selections. The fairness constraints are usually captured by absolute upper bounds and/or lower bounds on the number of selected candidates in specific attributes. In many scenarios, however, the total number of selected candidates is not predetermined. It is, therefore, more natural to express these fairness constraints in terms of proportions of the final selection size. In this paper, we study the proportional candidate selection problem, where the goal is to select a subset of candidates with maximum cardinality while meeting certain proportional fairness constraints. We first analyze the computational complexity of the problem and show strong inapproximability results. Next, we investigate the algorithmic aspects of the problem in two directions. First, by treating the proportional fairness constraints as soft constraints, we devise two polynomial-time algorithms that could return (near) optimal solutions with bounded violations on each fairness constraint. Second, we design an exact algorithm with a fast running time in practice. Simulations based on both synthetic and publicly available data confirm the effectiveness and efficiency of our proposed algorithms.
更多查看译文
关键词
Multi-winner selections,Proportional fairness constraints,Variable number of winners,Soft constraints
AI 理解论文
溯源树
样例
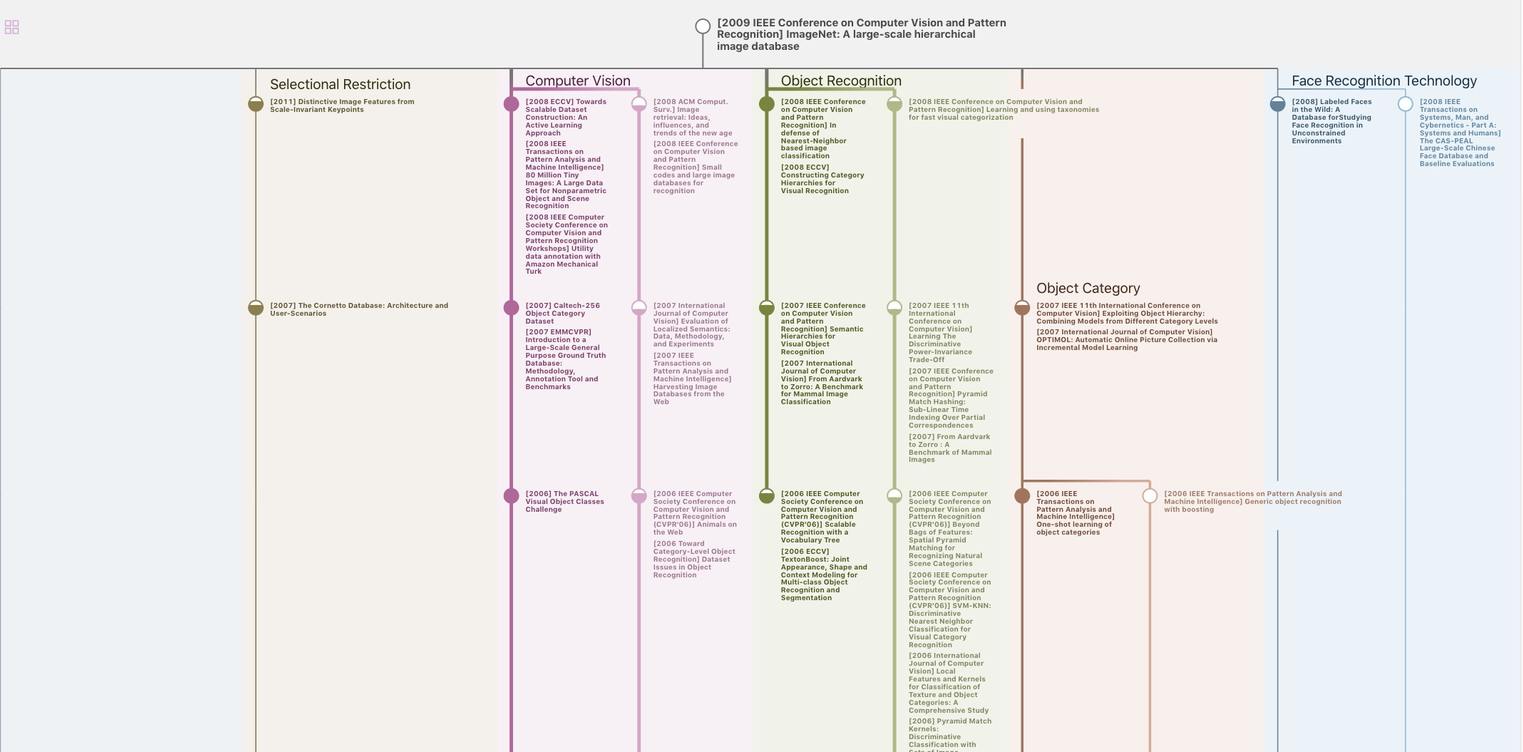
生成溯源树,研究论文发展脉络
Chat Paper
正在生成论文摘要