CoMet: A Meta Learning-Based Approach for Cross-Dataset Labeling Using Co-Training
AAMAS '19: International Conference on Autonomous Agents and Multiagent Systems Auckland New Zealand May, 2020(2020)
摘要
In many practical domains, applying machine learning is challenging not due to the lack of available data, but because labeled samples are in short supply. A common approach for obtaining additional labeled samples is co-training, a semi-supervised learning setting where two learners (agents), trained on different perspectives of the data, iteratively label additional samples. The rationale of this approach is that the different learner perspectives will produce a more diverse labeled set, resulting in more effective classifiers. While co-training proved effective in multiple cases, the labeling mechanisms used by existing approaches are heuristic and error-prone. We propose CoMet, a meta learning-based co-training algorithm. CoMet utilizes meta-models trained on previously-analyzed datasets to select the samples to be labeled for the current dataset. Our experiments, conducted on 35 datasets, show that CoMet significantly outperforms the standard co-training approach.
更多查看译文
AI 理解论文
溯源树
样例
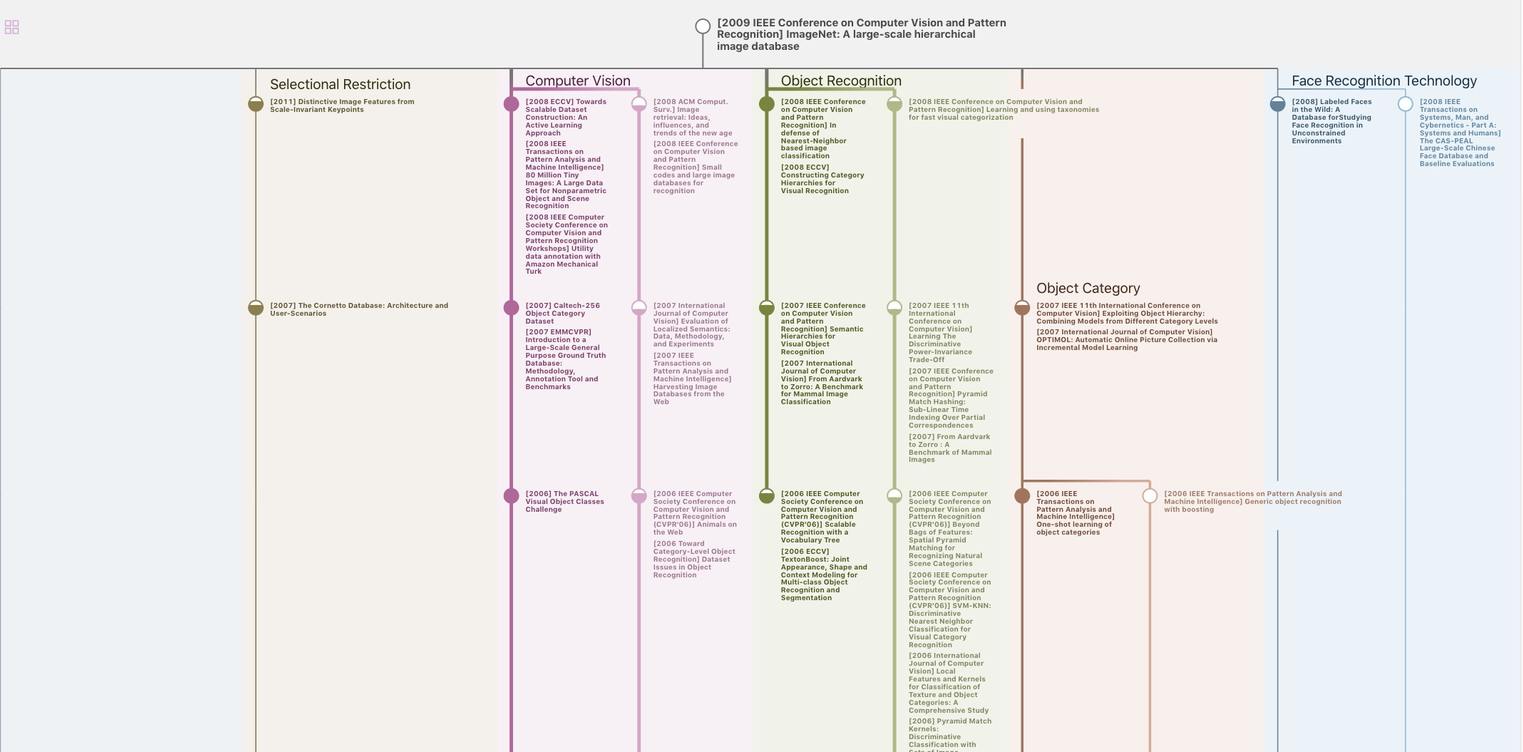
生成溯源树,研究论文发展脉络
Chat Paper
正在生成论文摘要