Learning by Reusing Previous Advice in Teacher-Student Paradigm
AAMAS '19: International Conference on Autonomous Agents and Multiagent Systems Auckland New Zealand May, 2020(2020)
摘要
Reinforcement Learning (RL) has been widely used to solve sequential decision-making problems. However, RL algorithms suffer from poor sample efficiency and require a long time to learn a suitable policy, especially when multiple agents are learning without prior knowledge. This problem can be alleviated through reusing knowledge from other agents during the learning process. One notable approach is advising actions based on a teacher-student relationship, where the decision of a student agent during learning is aided by an experienced teacher agent. A critical assumption in teacher-student paradigm is that the communication may be limited, so that a student may wait for a while and learn by itself before receiving the next advice. More importantly, in some noisy or stochastic environments, the student may not be able to master the advised actions when they are only performed once. We propose three methods for agents choosing between learning by exploration, asking for advice and reusing previous advice. The results show that our approaches significantly outperform existing advising methods without reusing advice.
更多查看译文
AI 理解论文
溯源树
样例
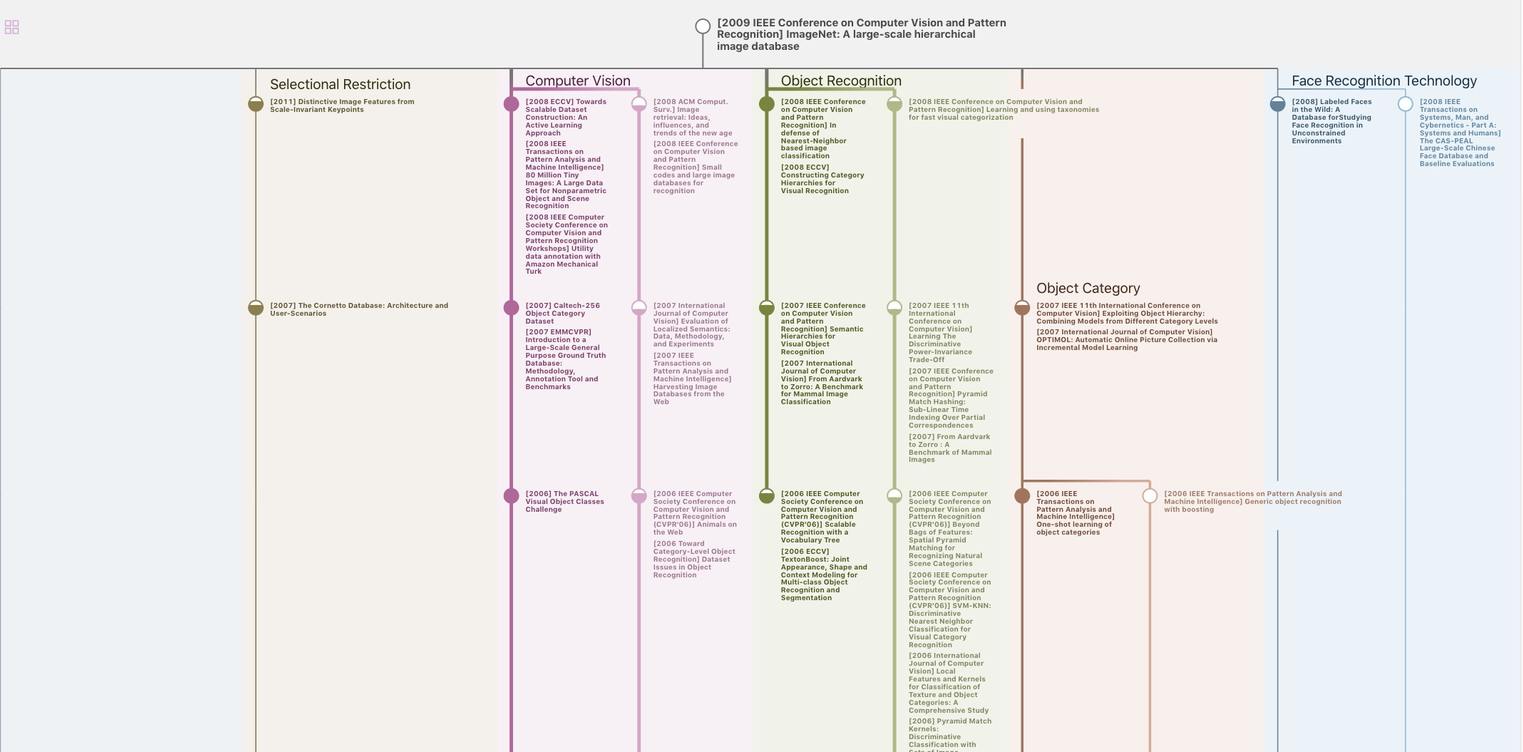
生成溯源树,研究论文发展脉络
Chat Paper
正在生成论文摘要