Multi-agent Adversarial Inverse Reinforcement Learning with Latent Variables
AAMAS '19: International Conference on Autonomous Agents and Multiagent Systems Auckland New Zealand May, 2020(2020)
摘要
We introduce an algorithm for inferring reward functions from expert human trajectories in multiagent environments. Current techniques exhibit poor sample-efficiency, lack stability in training, or scale poorly to large numbers of agents. We focus on settings with a large, variable number of agents and attempt to resolve these settings by exploiting similarities between agent behaviors. In particular, we learn a shared reward function using adversarial inverse reinforcement learning and a continuous latent variable. We demonstrate our algorithm on two real-world settings: traffic on highways and in terminal airspace.
更多查看译文
AI 理解论文
溯源树
样例
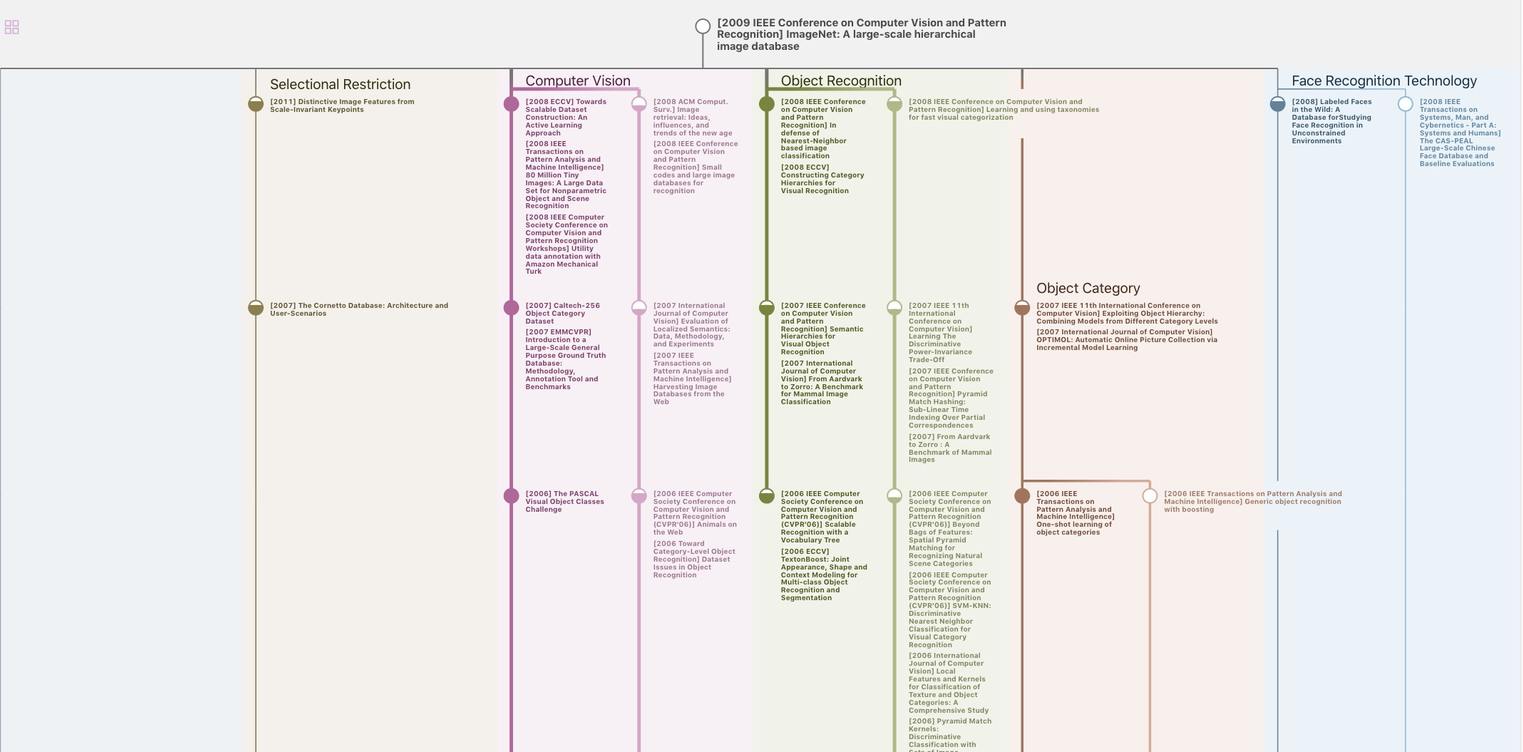
生成溯源树,研究论文发展脉络
Chat Paper
正在生成论文摘要