Matching Affinity Clustering: Improved Hierarchical Clustering at Scale with Guarantees
AAMAS '19: International Conference on Autonomous Agents and Multiagent Systems Auckland New Zealand May, 2020(2020)
摘要
Hierarchical clustering is a stronger extension of one of today's most influential unsupervised learning methods: clustering. The goal of this method is to create a hierarchy of clusters, thus constructing cluster evolutionary history and simultaneously finding clusterings at all resolutions. We propose four traits of interest for hierarchical clustering algorithms: (1) empirical performance, (2) theoretical guarantees, (3) balance (the minimum ratio between cluster sizes), and (4) scalability. While a number of algorithms are designed to achieve one to two of these traits at a time, there exist none that achieve all four.
Inspired by Bateni et al.'s scalable and empirically successful Affinity Clustering [NeurIPs 2017], we introduce Affinity's successor, Matching Affinity Clustering. Like its predecessor, Matching Affinity Clustering maintains strong empirical performance, even outperforming Affinity when the dataset is size 2n and clusters are balanced, and uses Massively Parallel Communication as its distributed model. Designed to maintain provably balanced clusters, we show that our algorithm achieves a (1/3-ε)-approximation for Moseley and Wang's revenue (the dual to Dasgupta's cost) when the data set is of size 2n, and a (1/9-ε)-approximation in general. We prove the former approximation is tight, and also that Affinity Clustering cannot do better than a 1/O(n)-approximation. In addition, we see that our algorithm empirically performs similarly to Affinity Clustering and k-Means, outperforming many state-of-the-art serial algorithms. Along the way, we also introduce an efficient k-sized maximum matching algorithm in the MPC model.
更多查看译文
AI 理解论文
溯源树
样例
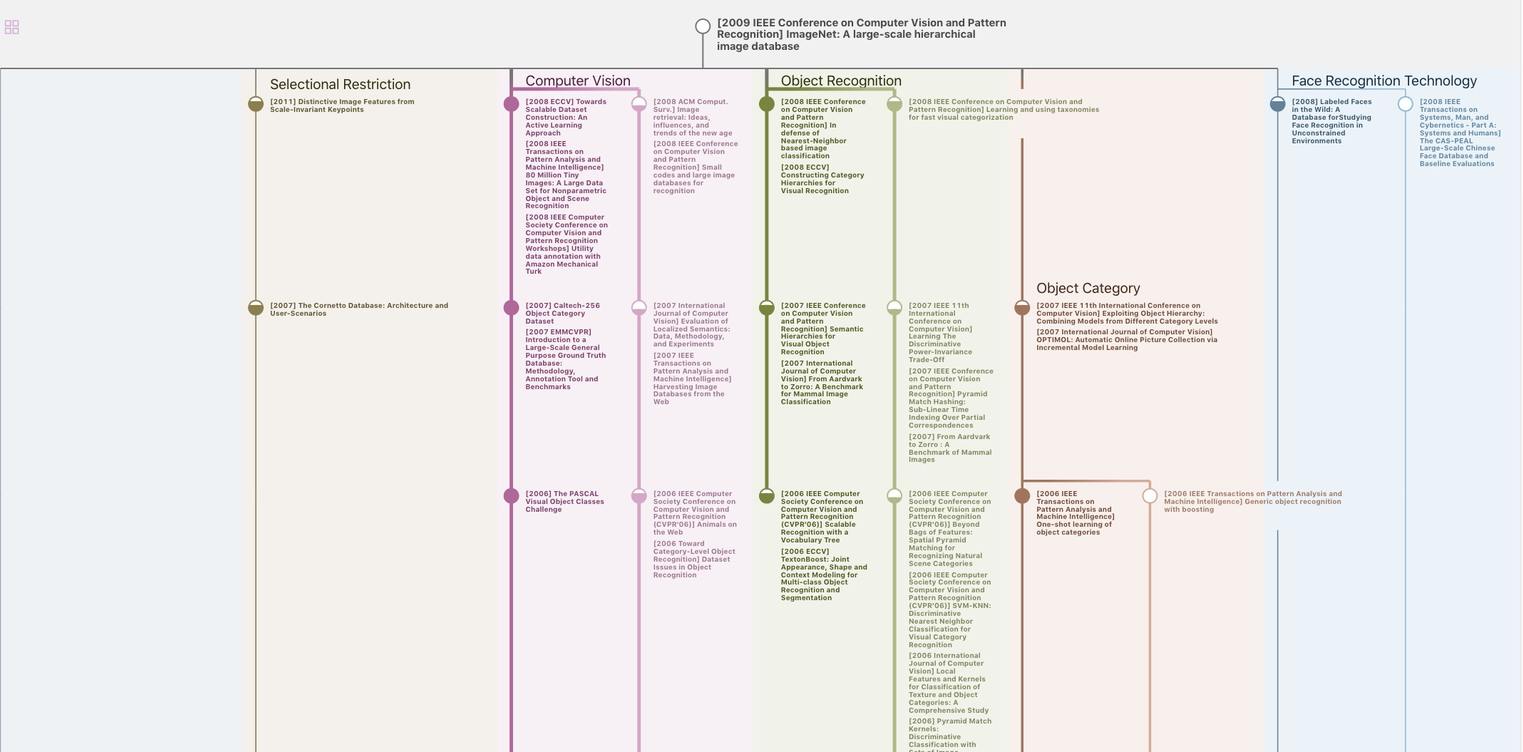
生成溯源树,研究论文发展脉络
Chat Paper
正在生成论文摘要