Super-resolution and Robust Sparse Continuous Fourier Transform in Any Constant Dimension: Nearly Linear Time and Sample Complexity
arxiv(2022)
摘要
The ability to resolve detail in the object that is being imaged, named by resolution, is the core parameter of an imaging system. Super-resolution is a class of techniques that can enhance the resolution of an imaging system and even transcend the diffraction limit of systems. Despite huge success in the application, super-resolution is not well understood on the theoretical side, especially for any dimension $d \geq 2$. In particular, in order to recover a $k$-sparse signal, all previous results suffer from either/both poly$(k)$ samples or running time. We design robust algorithms for any (constant) dimension under a strong noise model based on developing some new techniques in Sparse Fourier transform (Sparse FT), such as inverting a robust linear system, "eggshell" sampling schemes, and partition and voting methods in high dimension. These algorithms are the first to achieve running time and sample complexity (nearly) linear in the number of source points and logarithmic in bandwidth for any constant dimension, and we believe the techniques developed in the work can find their further applications on the Super-resolution and Sparse FT problem.
更多查看译文
关键词
sparse,fourier,multi-dimensional
AI 理解论文
溯源树
样例
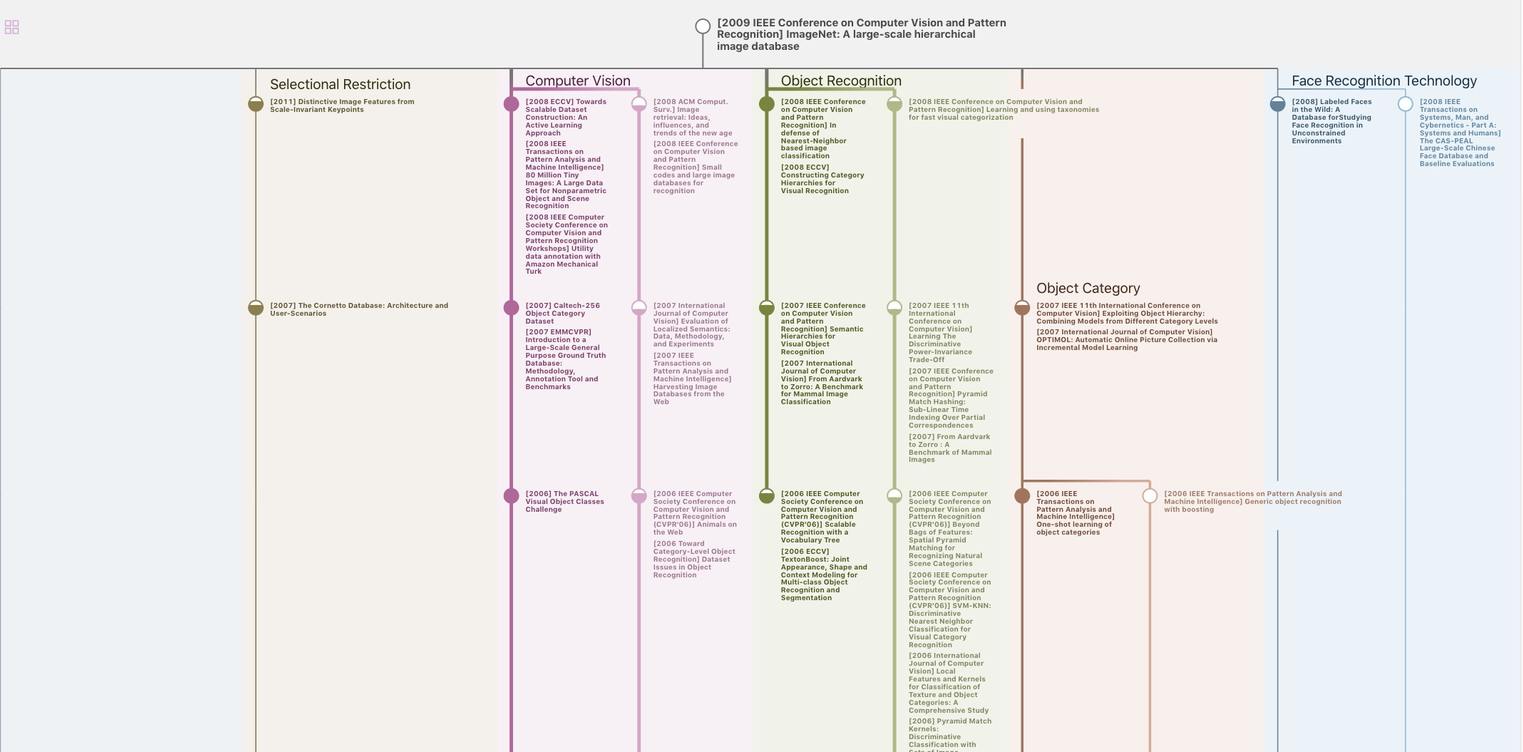
生成溯源树,研究论文发展脉络
Chat Paper
正在生成论文摘要