Action Image Representation: Learning Scalable Deep Grasping Policies with Zero Real World Data
ICRA(2020)
摘要
This paper introduces Action Image, a new grasp proposal representation that allows learning an end-to-end deep-grasping policy. Our model achieves $84\%$ grasp success on $172$ real world objects while being trained only in simulation on $48$ objects with just naive domain randomization. Similar to computer vision problems, such as object detection, Action Image builds on the idea that object features are invariant to translation in image space. Therefore, grasp quality is invariant when evaluating the object-gripper relationship; a successful grasp for an object depends on its local context, but is independent of the surrounding environment. Action Image represents a grasp proposal as an image and uses a deep convolutional network to infer grasp quality. We show that by using an Action Image representation, trained networks are able to extract local, salient features of grasping tasks that generalize across different objects and environments. We show that this representation works on a variety of inputs, including color images (RGB), depth images (D), and combined color-depth (RGB-D). Our experimental results demonstrate that networks utilizing an Action Image representation exhibit strong domain transfer between training on simulated data and inference on real-world sensor streams. Finally, our experiments show that a network trained with Action Image improves grasp success ($84\%$ vs. $53\%$) over a baseline model with the same structure, but using actions encoded as vectors.
更多查看译文
关键词
Action image representation,zero real world data,end-to-end deep-grasping policy,grasp quality,object-gripper relationship,deep convolutional network,Action Image representation,color images,depth images,combined color-depth,scalable deep grasping policy learning,salient feature extraction
AI 理解论文
溯源树
样例
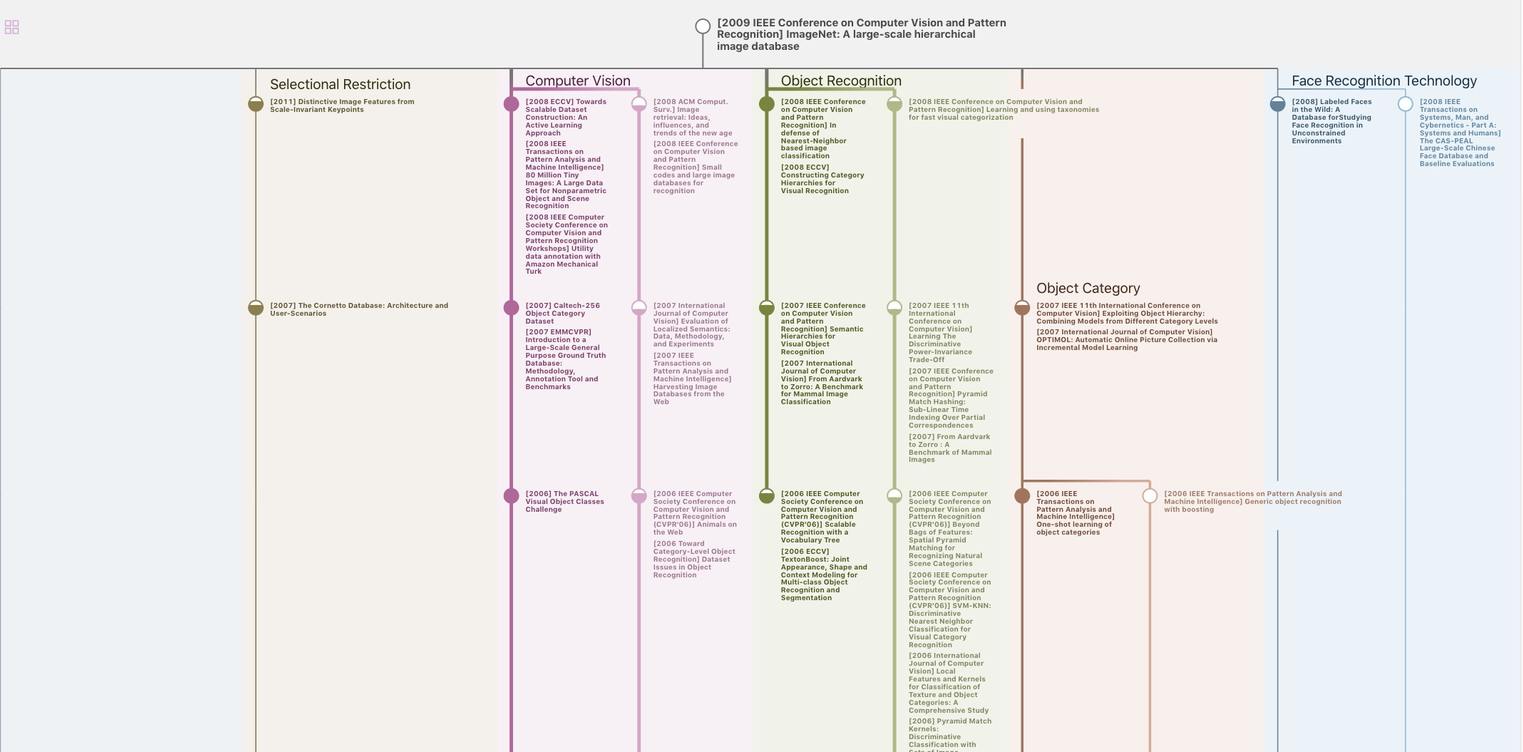
生成溯源树,研究论文发展脉络
Chat Paper
正在生成论文摘要