Training conformal predictors
COPA(2020)
摘要
Efficiency criteria for conformal prediction, such as \emph{observed fuzziness} (i.e., the sum of p-values associated with false labels), are commonly used to \emph{evaluate} the performance of given conformal predictors. Here, we investigate whether it is possible to exploit efficiency criteria to \emph{learn} classifiers, both conformal predictors and point classifiers, by using such criteria as training objective functions. The proposed idea is implemented for the problem of binary classification of hand-written digits. By choosing a 1-dimensional model class (with one real-valued free parameter), we can solve the optimization problems through an (approximate) exhaustive search over (a discrete version of) the parameter space. Our empirical results suggest that conformal predictors trained by minimizing their observed fuzziness perform better than conformal predictors trained in the traditional way by minimizing the \emph{prediction error} of the corresponding point classifier. They also have a reasonable performance in terms of their prediction error on the test set.
更多查看译文
关键词
conformal predictors,training
AI 理解论文
溯源树
样例
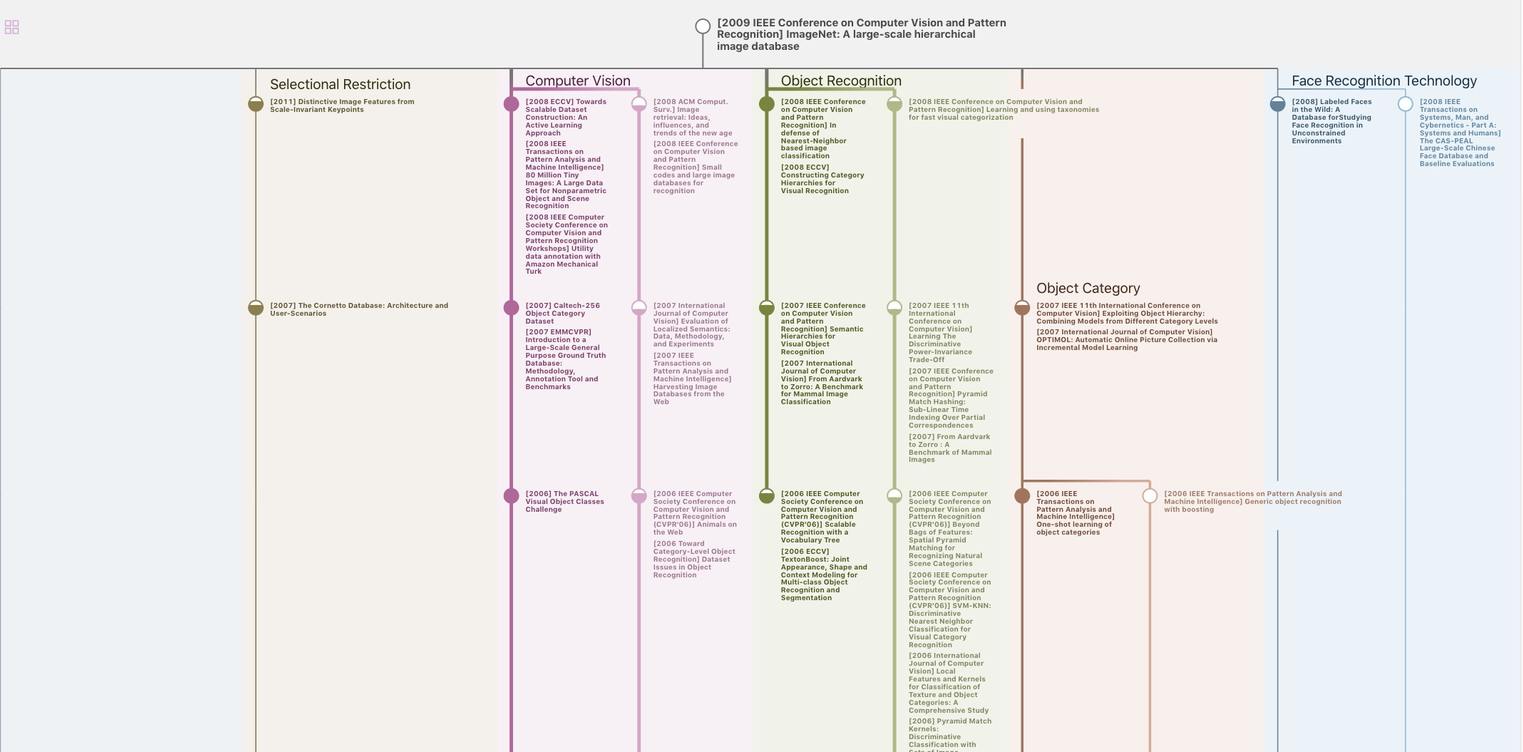
生成溯源树,研究论文发展脉络
Chat Paper
正在生成论文摘要