Unsupervised Severe Weather Detection Via Joint Representation Learning Over Textual and Weather Data
SETN Workshops(2020)
摘要
When observing a phenomenon, severe cases or anomalies are often characterised by deviation from the expected data distribution. However, non-deviating data samples may also implicitly lead to severe outcomes. In the case of unsupervised severe weather detection, these data samples can lead to mispredictions, since the predictors of severe weather are often not directly observed as features. We posit that incorporating external or auxiliary information, such as the outcome of an external task or an observation, can improve the decision boundaries of an unsupervised detection algorithm. In this paper, we increase the effectiveness of a clustering method to detect cases of severe weather by learning augmented and linearly separable latent representations.We evaluate our solution against three individual cases of severe weather, namely windstorms, floods and tornado outbreaks.
更多查看译文
关键词
weather
AI 理解论文
溯源树
样例
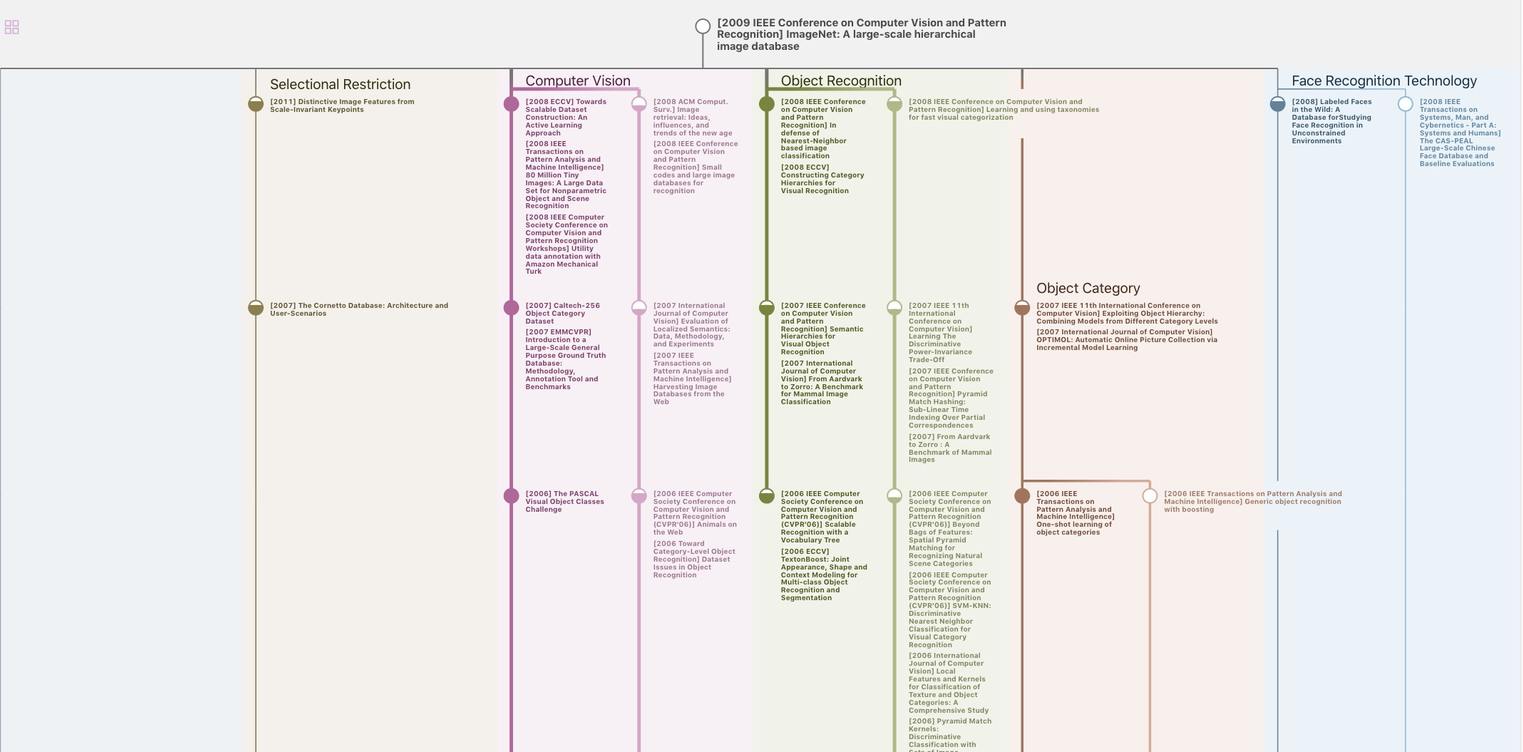
生成溯源树,研究论文发展脉络
Chat Paper
正在生成论文摘要