Viraal: Virtual Adversarial Active Learning For Nlu
2021 IEEE SPOKEN LANGUAGE TECHNOLOGY WORKSHOP (SLT)(2021)
摘要
This paper presents VirAAL, an Active Learning framework based on Adversarial Training. VirAAL aims to reduce the effort of annotation in Natural Language Understanding (NLU). VirAAL is based on Virtual Adversarial Training (VAT), a semi-supervised approach that regularizes the model through Local Distributional Smoothness. With that, adversarial perturbations are added to the inputs making the posterior distribution more consistent. Therefore, entropy-based Active Learning becomes robust by querying more informative samples without requiring additional components. The first set of experiments studies the impact of an adapted VAT for joint-NLU tasks within low labeled data regimes. The second set shows the effect of VirAAL in an Active Learning (AL) process. Results demonstrate that VAT is robust even on multi-task training, where the adversarial noise is computed from multiple loss functions. Substantial improvements are observed with entropy-based AL with VirAAL for querying data to annotate. VirAAL is an inexpensive method in terms of AL computation with a positive impact on data sampling. Furthermore, VirAAL decreases annotations in AL up to 80% and shows improvements over existing data augmentation methods. The code is publicly available.
更多查看译文
关键词
Spoken Language Understanding, Virtual Adversarial Training, Low Data Regime, Active Learning
AI 理解论文
溯源树
样例
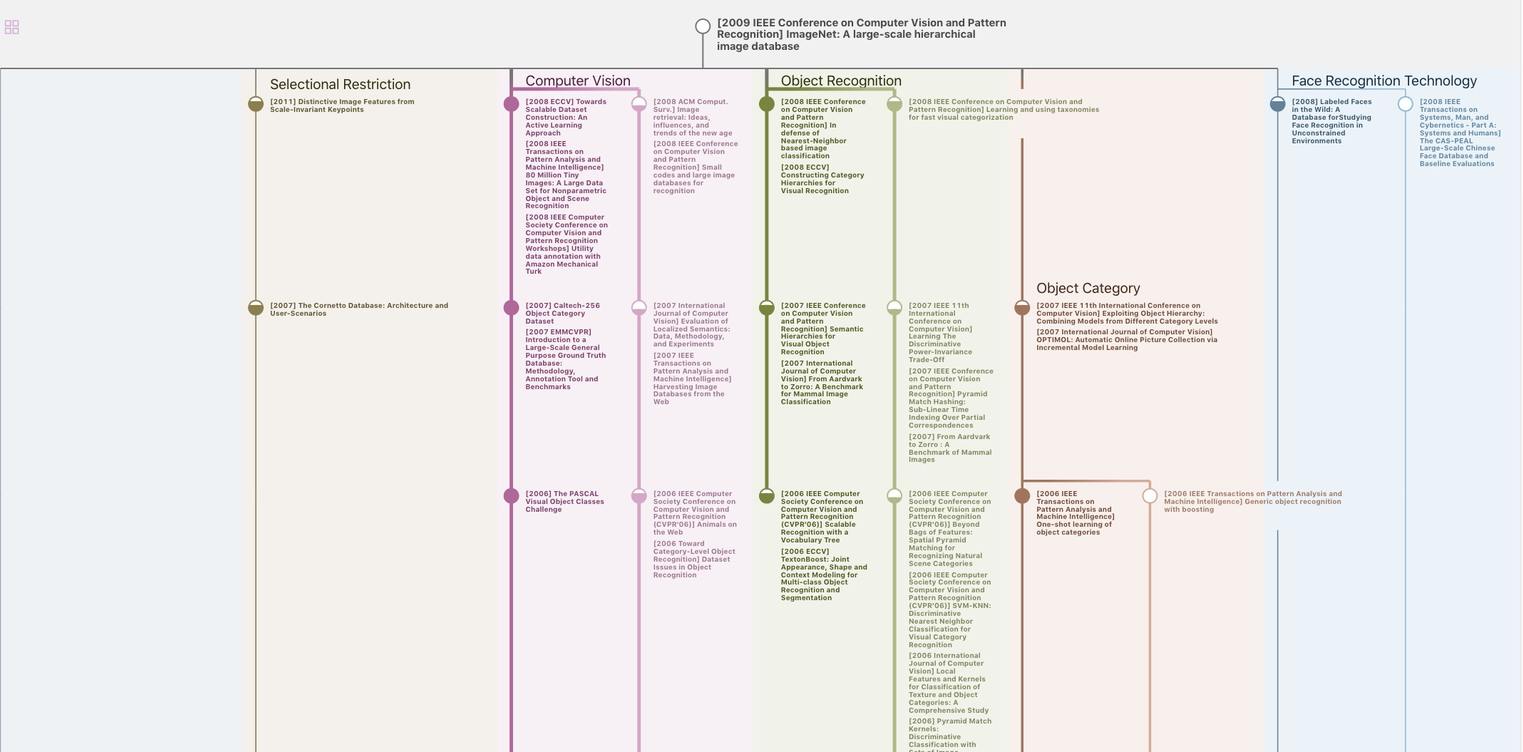
生成溯源树,研究论文发展脉络
Chat Paper
正在生成论文摘要