Learning Probabilistic Sentence Representations From Paraphrases
5TH WORKSHOP ON REPRESENTATION LEARNING FOR NLP (REPL4NLP-2020)(2020)
摘要
Probabilistic word embeddings have shown effectiveness in capturing notions of generality and entailment, but there is very little work on doing the analogous type of investigation for sentences. In this paper we define probabilistic models that produce distributions for sentences. Our best-performing model treats each word as a linear transformation operator applied to a multivariate Gaussian distribution. We train our models on paraphrases and demonstrate that they naturally capture sentence specificity. While our proposed model achieves the best performance overall, we also show that specificity is represented by simpler architectures via the norm of the sentence vectors. Qualitative analysis shows that our probabilistic model captures sentential entailment and provides ways to analyze the specificity and preciseness of individual words.
更多查看译文
关键词
probabilistic sentence representations,paraphrases,learning
AI 理解论文
溯源树
样例
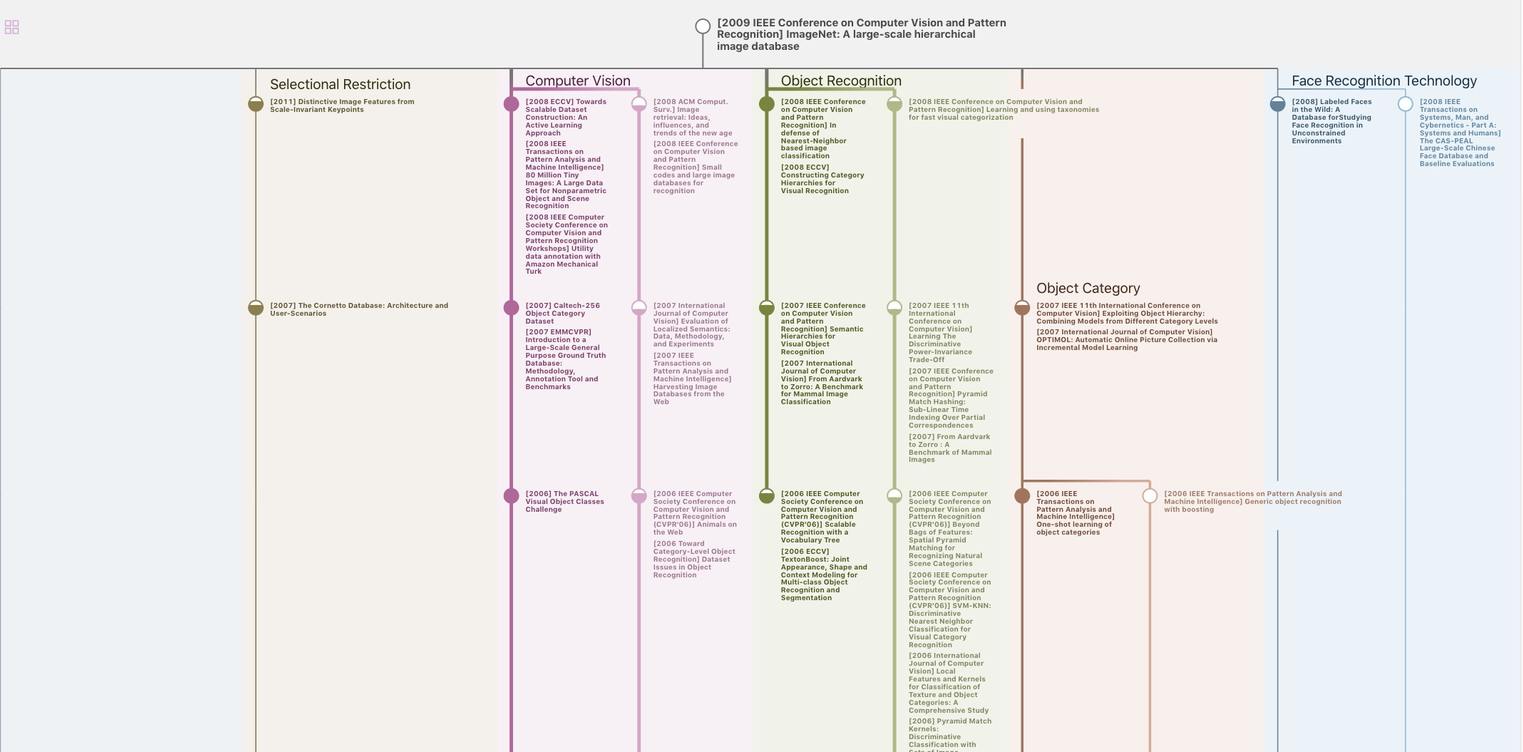
生成溯源树,研究论文发展脉络
Chat Paper
正在生成论文摘要