Efficient Wait-k Models for Simultaneous Machine Translation
INTERSPEECH(2020)
摘要
Simultaneous machine translation consists in starting output generation before the entire input sequence is available. Wait-k decoders offer a simple but efficient approach for this problem. They first read k source tokens, after which they alternate between producing a target token and reading another source token. We investigate the behavior of wait-k decoding in low resource settings for spoken corpora using IWSLT datasets. We improve training of these models using unidirectional encoders, and training across multiple values of k. Experiments with Transformer and 2D-convolutional architectures show that our wait-k models generalize well across a wide range of latency levels. We also show that the 2D-convolution architecture is competitive with Transformers for simultaneous translation of spoken language.
更多查看译文
关键词
translation,models,machine
AI 理解论文
溯源树
样例
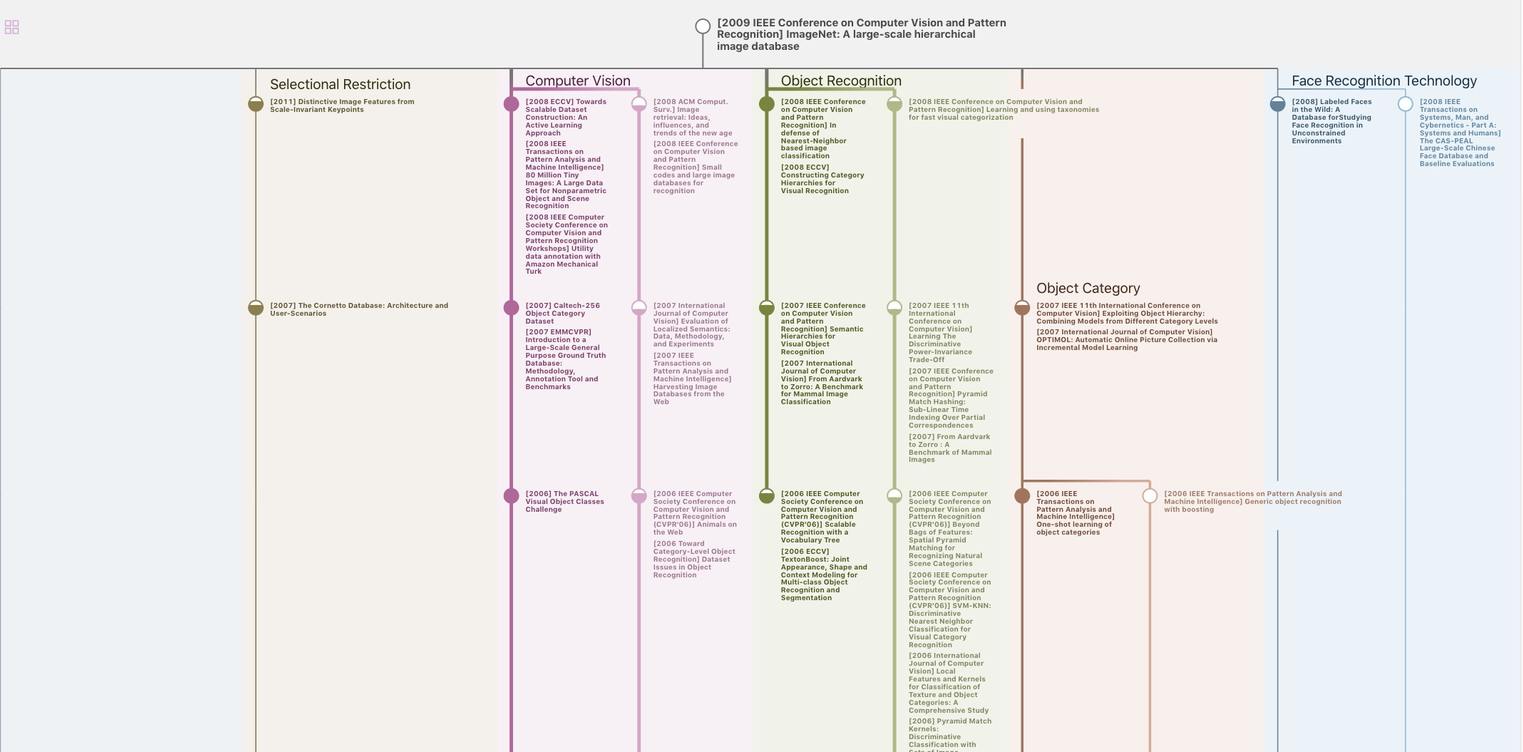
生成溯源树,研究论文发展脉络
Chat Paper
正在生成论文摘要