DDD20 End-to-End Event Camera Driving Dataset: Fusing Frames and Events with Deep Learning for Improved Steering Prediction
2020 IEEE 23rd International Conference on Intelligent Transportation Systems (ITSC)(2020)
摘要
Neuromorphic event cameras are useful for dynamic vision problems under difficult lighting conditions. To enable studies of using event cameras in automobile driving applications, this paper reports a new end-to-end driving dataset called DDD20. The dataset was captured with a DAVIS camera that concurrently streams both dynamic vision sensor (DVS) brightness change events and active pixel sensor (APS) intensity frames. DDD20 is the longest event camera end-to-end driving dataset to date with 51h of DAVIS event+frame camera and vehicle human control data collected from 4000km of highway and urban driving under a variety of lighting conditions. Using DDD20, we report the first study of fusing brightness change events and intensity frame data using a deep learning approach to predict the instantaneous human steering wheel angle. Over all day and night conditions, the explained variance for human steering prediction from a Resnet-32 is significantly better from the fused DVS+APS frames (0.88) than using either DVS (0.67) or APS (0.77) data alone.
更多查看译文
关键词
fused DVS+APS frames,human steering prediction,instantaneous human steering wheel angle,deep learning approach,intensity frame data,fusing brightness change events,urban driving,DAVIS event+frame camera,longest event camera end-to-end,active pixel sensor intensity frames,concurrently streams both dynamic vision sensor brightness,end-to-end driving dataset,automobile driving applications,dynamic vision problems,neuromorphic event cameras,improved steering prediction,fusing frames,DDD20 end-to-end event camera
AI 理解论文
溯源树
样例
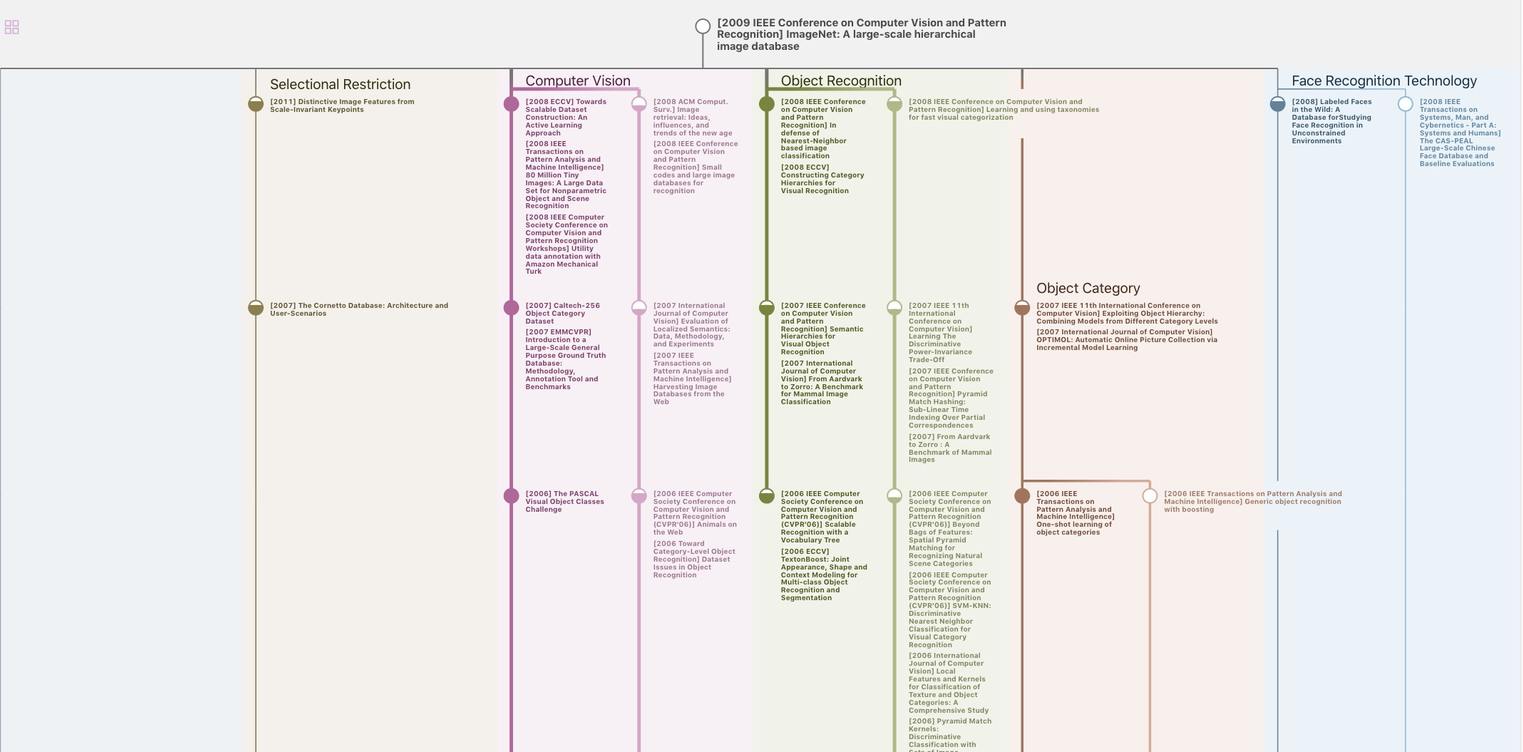
生成溯源树,研究论文发展脉络
Chat Paper
正在生成论文摘要