Spatio-Temporal Point Processes With Attention for Traffic Congestion Event Modeling
IEEE Transactions on Intelligent Transportation Systems(2022)
摘要
We present a novel framework for modeling traffic congestion events over road networks. Using multi-modal data by combining count data from traffic sensors with police reports that report traffic incidents, we aim to capture two types of triggering effect for congestion events. Current traffic congestion at one location may cause future congestion over the road network, and traffic incidents may cause spread traffic congestion. To model the non-homogeneous temporal dependence of the event on the past, we use a novel attention-based mechanism based on neural networks embedding for point processes. To incorporate the directional spatial dependence induced by the road network, we adapt the “tail-up” model from the context of spatial statistics to the traffic network setting. We demonstrate our approach’s superior performance compared to the state-of-the-art methods for both synthetic and real data.
更多查看译文
关键词
Traffic congestion,attention,point process
AI 理解论文
溯源树
样例
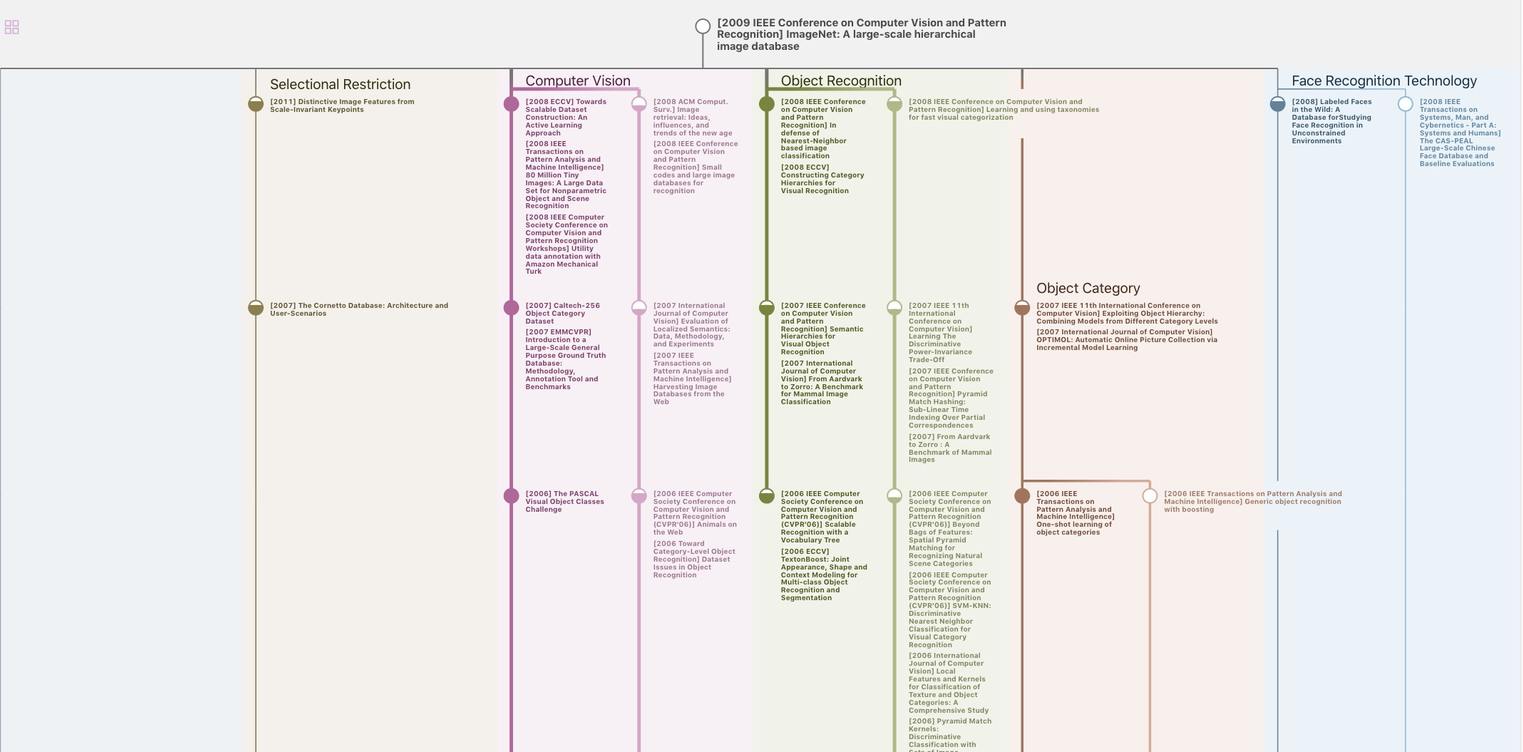
生成溯源树,研究论文发展脉络
Chat Paper
正在生成论文摘要