Reactive Global Minimum Variance Portfolios With K-Bahc Covariance Cleaning
arxiv(2023)
摘要
We introduce a covariance cleaning method which works well in the very high-dimensional regime, i.e. when there are many more assets than data points per asset. This opens the way to unconditional reactive portfolio optimization when there are not enough points to calibrate dynamical conditional covariance models, which happens, for example, when new assets appear in a market. The method is a k-fold boosted version of the Bootstrapped Average Hierarchical Clustering cleaning procedure for correlation and covariance matrices. We apply this method to global minimum variance portfolios and find that k should increase with the calibration window length. We compare the performance of k-BAHC with other state-of-the-art covariance cleaning methods, including dynamical conditional covariance (DCC) with non-linear shrinkage. Generally, we find that our method yields better Sharpe ratios after transaction costs than competing unconditional covariance filtering methods, despite requiring a larger turnover. Finally, k-BAHC yields better Global Minimum Variance portfolios with long-short positions than DCC in a non-stationary investment universe.
更多查看译文
关键词
Covariance matrix cleaning, portfolio optimization, global minimum variance portfolios, realized risk, dynamic conditional covariance
AI 理解论文
溯源树
样例
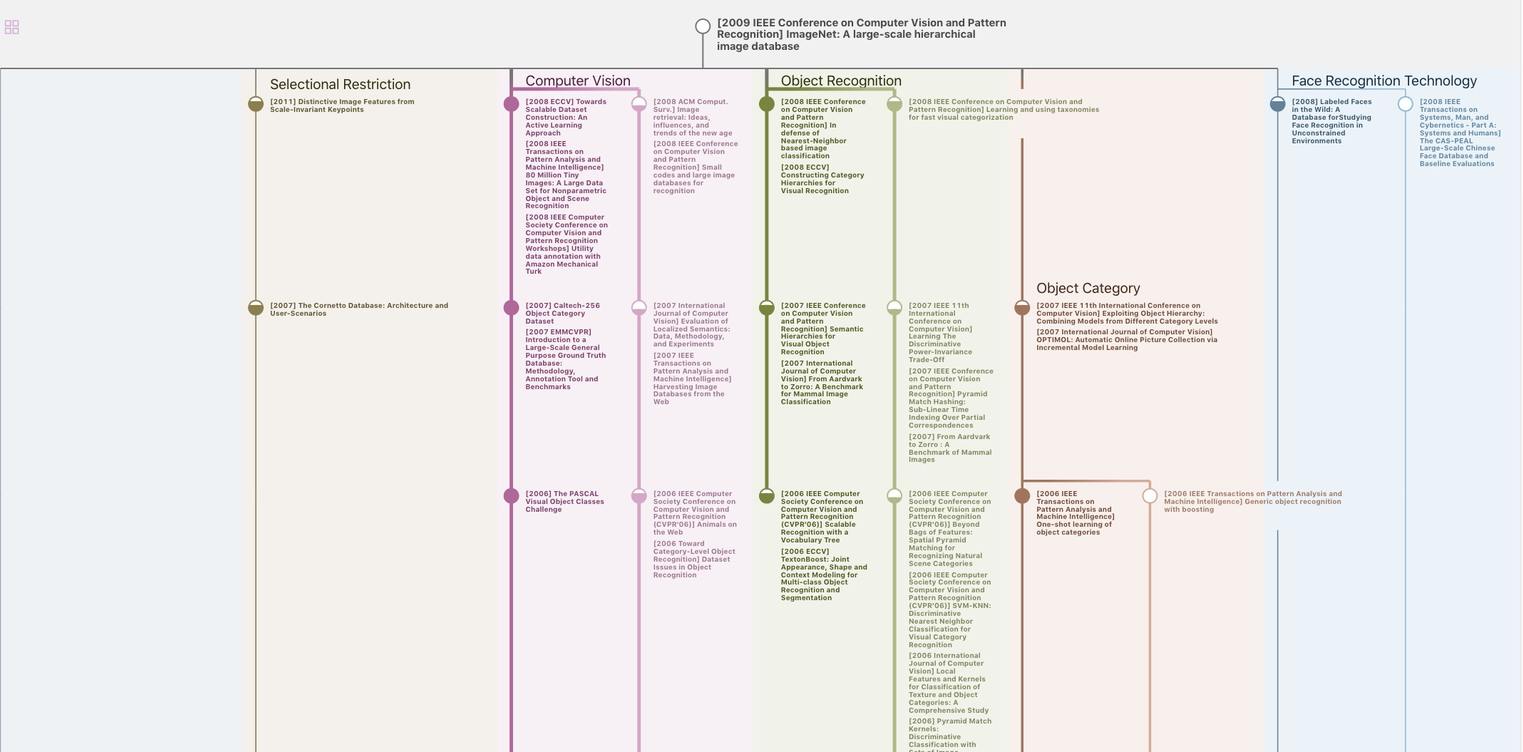
生成溯源树,研究论文发展脉络
Chat Paper
正在生成论文摘要