Visual Memorability for Robotic Interestingness via Unsupervised Online Learning
arxiv(2020)
摘要
In this paper, we explore the problem of interesting scene prediction for mobile robots. This area is currently underexplored but is crucial for many practical applications such as autonomous exploration and decision making. Inspired by industrial demands, we first propose a novel translation-invariant visual memory for recalling and identifying interesting scenes, then design a three-stage architecture of long-term, short-term, and online learning. This enables our system to learn human-like experience, environmental knowledge, and online adaption, respectively. Our approach achieves much higher accuracy than the state-of-the-art algorithms on challenging robotic interestingness datasets.
更多查看译文
关键词
Unsupervised, Online, Memorability, Interestingness
AI 理解论文
溯源树
样例
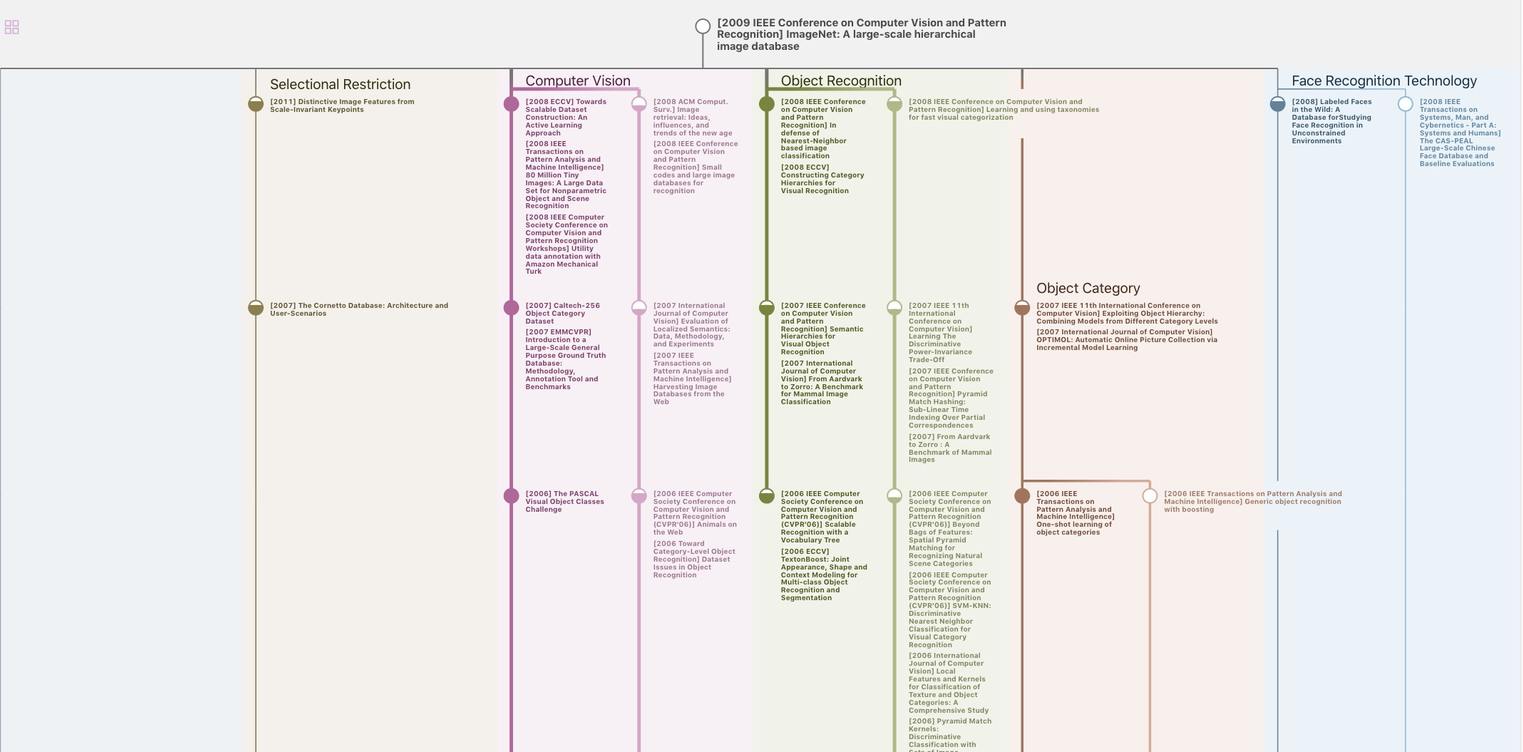
生成溯源树,研究论文发展脉络
Chat Paper
正在生成论文摘要