Deep Snow: Synthesizing Remote Sensing Imagery With Generative Adversarial Nets
ALGORITHMS, TECHNOLOGIES, AND APPLICATIONS FOR MULTISPECTRAL AND HYPERSPECTRAL IMAGERY XXVI(2020)
摘要
In this work we demonstrate that generative adversarial networks (GANs) can be used to generate realistic pervasive changes in RGB remote sensing imagery, even in an unpaired training setting. We investigate some transformation quality metrics based on deep embedding of the generated and real images which enable visualization and understanding of the training dynamics of the GAN, and provide a useful measure in terms of quantifying how distinguishable the generated images are from real images. We also identify some artifacts introduced by the GAN in the generated images, which are likely to contribute to the differences seen between the real and generated samples in the deep embedding feature space even in cases where the real and generated samples appear perceptually similar.
更多查看译文
关键词
Deep Learning, Generative Adversarial Networks, Machine Learning
AI 理解论文
溯源树
样例
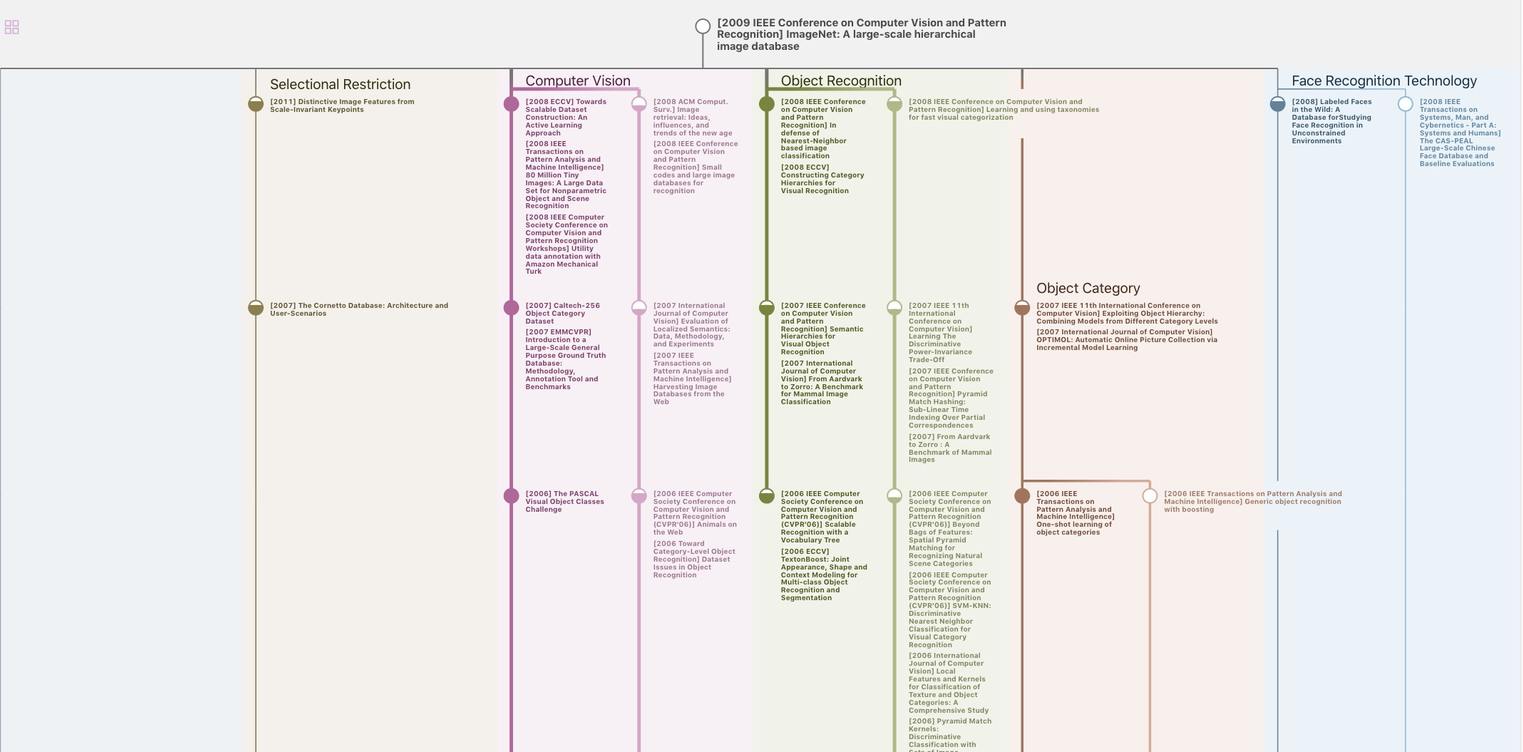
生成溯源树,研究论文发展脉络
Chat Paper
正在生成论文摘要