Learning Deep Models from Synthetic Data for Extracting Dolphin Whistle Contours
IJCNN(2020)
摘要
We present a learning-based method for extracting whistles of toothed whales (Odontoceti) in hydrophone recordings. Our method represents audio signals as time-frequency spectrograms and decomposes each spectrogram into a set of time-frequency patches. A deep neural network learns archetypical patterns (e.g., crossings, frequency modulated sweeps) from the spectrogram patches and predicts time-frequency peaks that are associated with whistles. We also developed a comprehensive method to synthesize training samples from background environments and train the network with minimal human annotation effort. We applied the proposed learn-from-synthesis method to a subset of the public Detection, Classification, Localization, and Density Estimation (DCLDE) 2011 workshop data to extract whistle confidence maps, which we then processed with an existing contour extractor to produce whistle annotations. The F1-score of our best synthesis method was 0.158 greater than our baseline whistle extraction algorithm (~25% improvement) when applied to common dolphin (Delphinus spp.) and bottlenose dolphin (Tursiops truncatus) whistles.
更多查看译文
关键词
Whistle contour extraction,deep neural network,data synthesis,acoustic,odontocetes
AI 理解论文
溯源树
样例
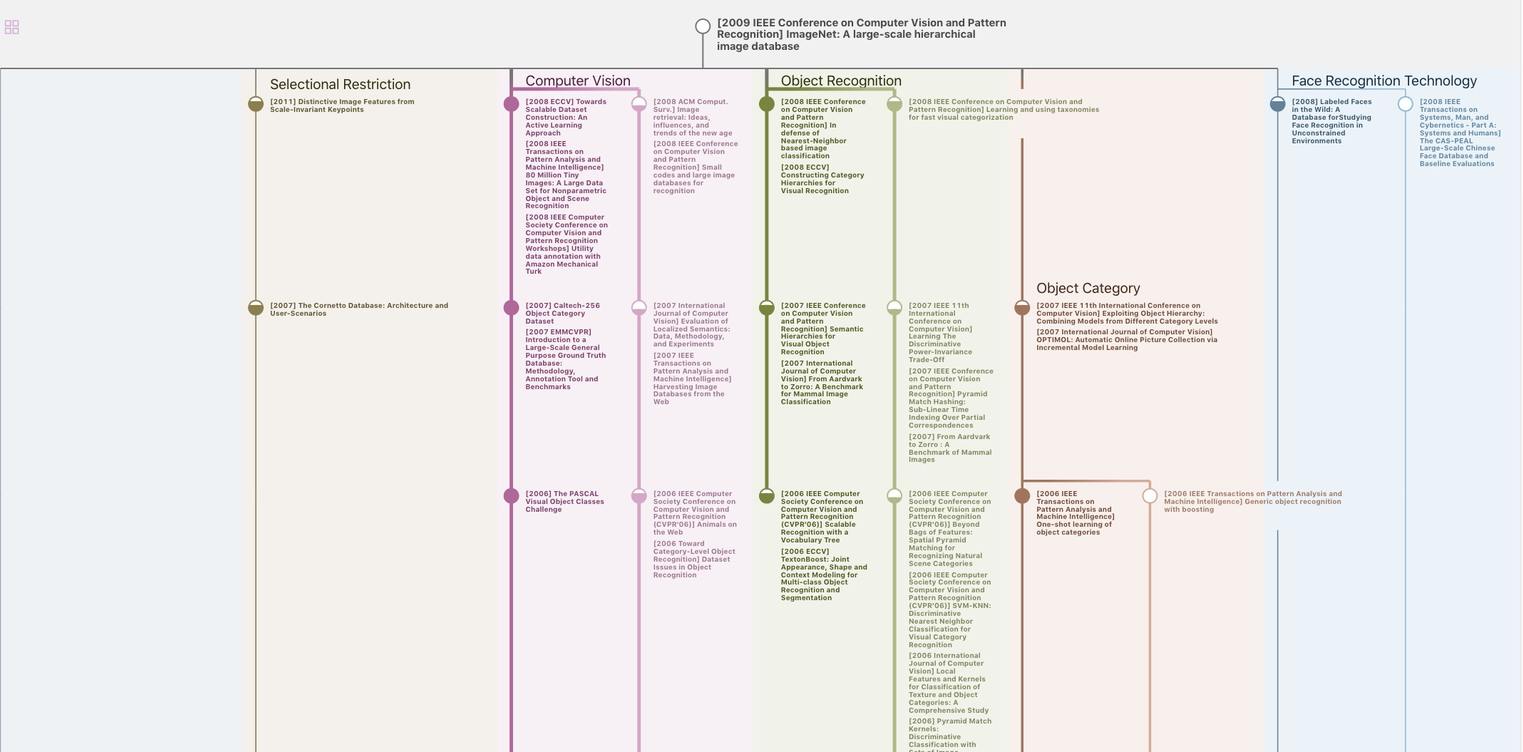
生成溯源树,研究论文发展脉络
Chat Paper
正在生成论文摘要