Combining forward with recurrent neural networks for hourly air quality prediction in Northwest of China
ENVIRONMENTAL SCIENCE AND POLLUTION RESEARCH(2020)
摘要
Data-driven statistical air quality prediction methods usually build models fast with moderate accuracy and have been studied a lot in recent years. However, due to the complexity of air quality prediction which usually involves multiple factors, such as meteorological, spatial, and temporal properties, it is still a challenge to propose a model with required accuracy. In this paper, we propose a hybrid ensemble model CERL to exploit the merits of both forward neural networks and recurrent neural networks that are designed for handling time serial data to predict air quality hourly. Measured air pollutant factors including Air Quality Index (AQI), PM 2.5 , PM 10 , CO, SO 2 , NO 2 , and O 3 are used as input to predict air quality from 1 to 8 h ahead. Based on the air quality prediction evaluation in Lanzhou and Xi’an, which are two important provincial capitals in Northwest China, CERL provides better performance over other baseline models. Moreover, as the step length increases, CERL has more obvious improvement. For example, the improvements of CERL in the 1-step, 3-step, 5-step, and 8-step prediction for PM 2.5 in Lanzhou are 1.82%, 8.01%, 9.98%, and 20.03%, respectively. The superiority of CERL is also proved by a hypothesis Diebold Mariano test with level of significance 5%.
更多查看译文
关键词
Air quality, Machine learning, Serial data, SSA, Sliding window, Neural network
AI 理解论文
溯源树
样例
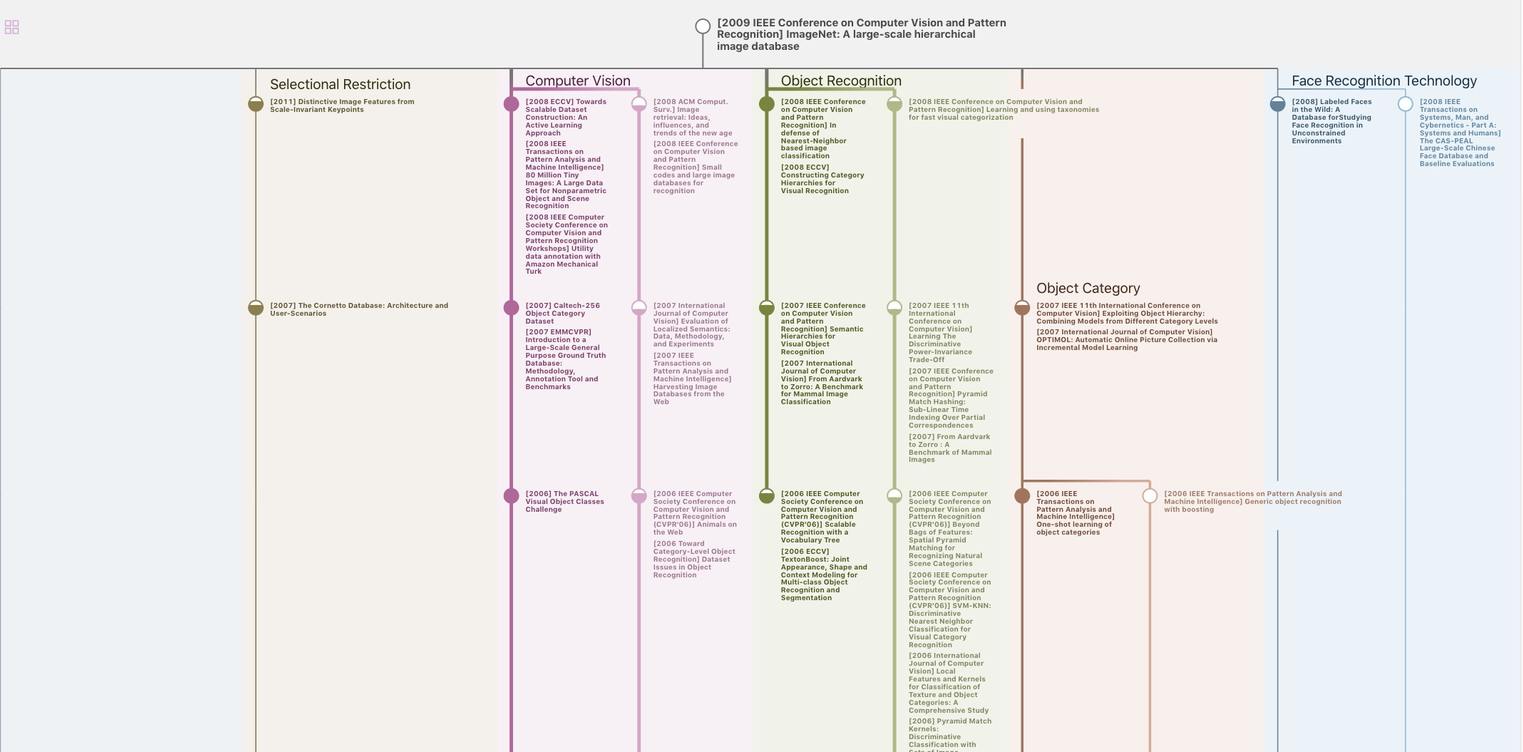
生成溯源树,研究论文发展脉络
Chat Paper
正在生成论文摘要