Learning To Recover From Multi-Modality Errors For Non-Autoregressive Neural Machine Translation
58TH ANNUAL MEETING OF THE ASSOCIATION FOR COMPUTATIONAL LINGUISTICS (ACL 2020)(2020)
摘要
Non-autoregressive neural machine translation (NAT) predicts the entire target sequence simultaneously and significantly accelerates inference process. However, NAT discards the dependency information in a sentence, and thus inevitably suffers from the multi-modality problem: the target tokens may be provided by different possible translations, often causing token repetitions or missing. To alleviate this problem, we propose a novel semi-autoregressive model RecoverSAT in this work, which generates a translation as a sequence of segments. The segments are generated simultaneously while each segment is predicted token-by-token. By dynamically determining segment length and deleting repetitive segments, RecoverSAT is capable of recovering from repetitive and missing token errors. Experimental results on three widely used benchmark datasets show that our proposed model achieves more than 4x speedup while maintaining comparable performance compared with the corresponding autoregressive model.
更多查看译文
关键词
translation,learning,multi-modality,non-autoregressive
AI 理解论文
溯源树
样例
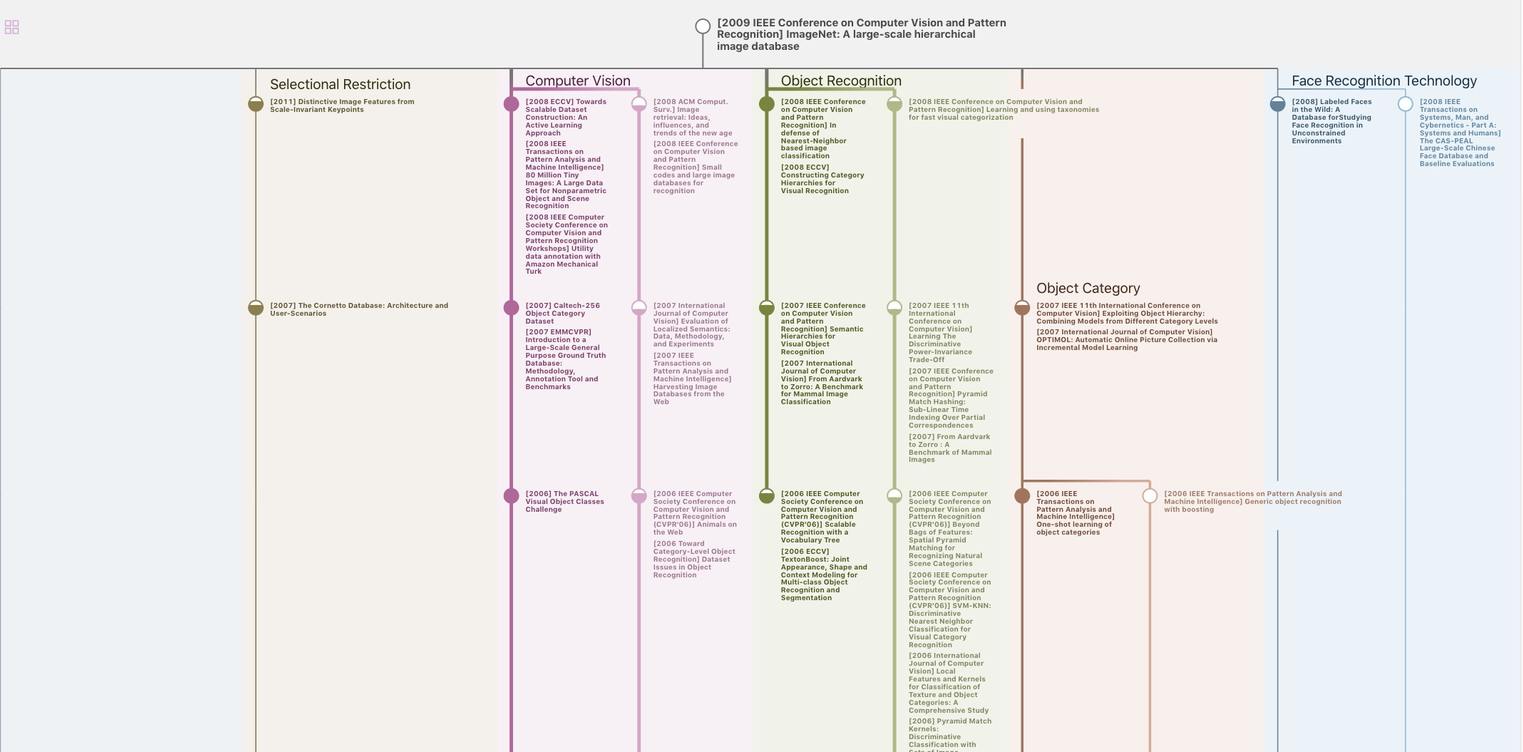
生成溯源树,研究论文发展脉络
Chat Paper
正在生成论文摘要