Contextual Embeddings: When Are They Worth It?
58TH ANNUAL MEETING OF THE ASSOCIATION FOR COMPUTATIONAL LINGUISTICS (ACL 2020)(2020)
摘要
We study the settings for which deep contextual embeddings (e.g., BERT) give large improvements in performance relative to classic pretrained embeddings (e.g., GloVe), and an even simpler baseline-random word embeddings-focusing on the impact of the training set size and the linguistic properties of the task. Surprisingly, we find that both of these simpler baselines can match contextual embeddings on industry-scale data, and often perform within 5 to 10% accuracy (absolute) on benchmark tasks. Furthermore, we identify properties of data for which contextual embeddings give particularly large gains: language containing complex structure, ambiguous word usage, and words unseen in training.
更多查看译文
关键词
contextual embeddings
AI 理解论文
溯源树
样例
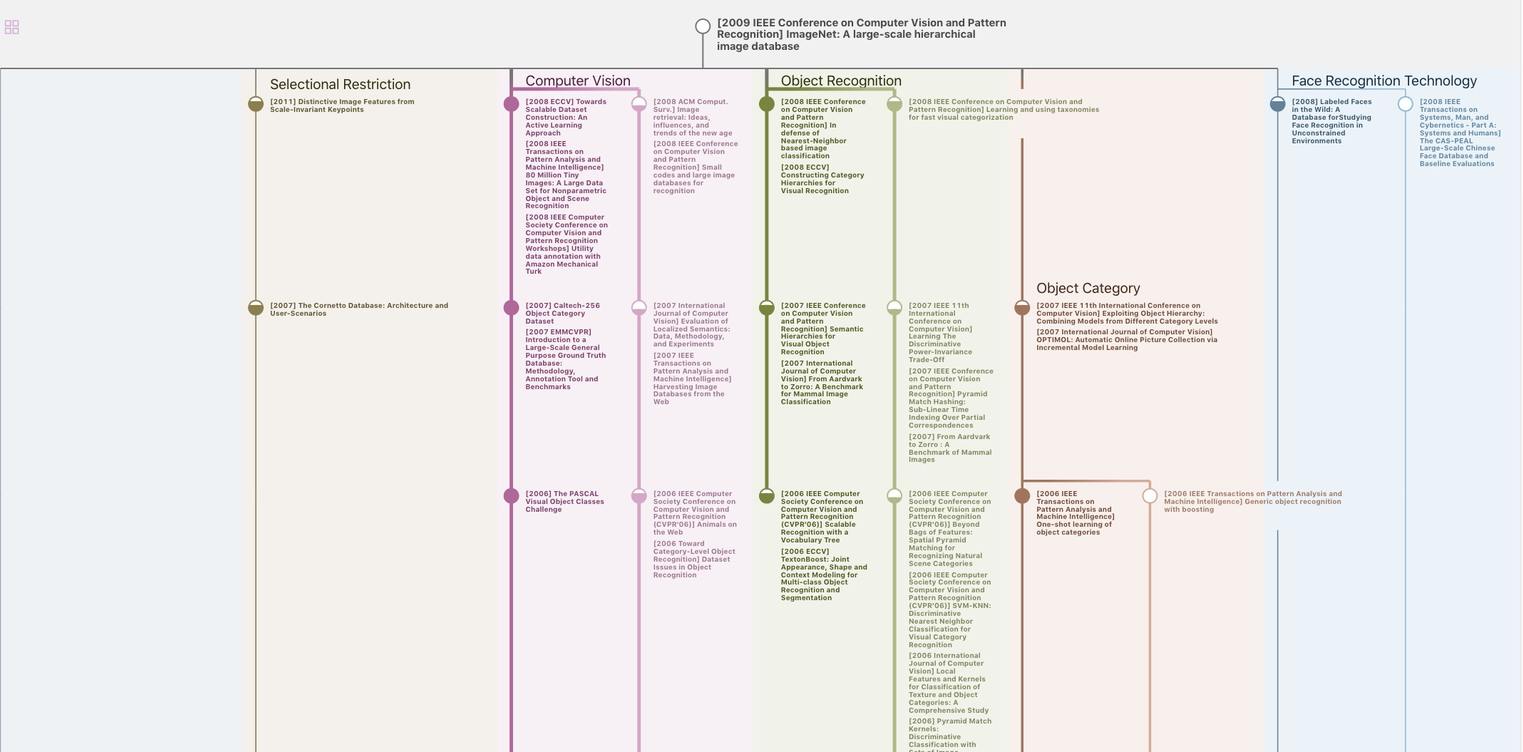
生成溯源树,研究论文发展脉络
Chat Paper
正在生成论文摘要