Preference-Based Image Generation
2020 IEEE WINTER CONFERENCE ON APPLICATIONS OF COMPUTER VISION (WACV)(2020)
摘要
Deep generative models are a set of promising methods, that are able to model complex data and generate new samples. In principle, they learn to map a random latent code sampled from a prior distribution into a high dimensional data space, such as image space. However, these models have limited utilities as the user has minimal control over what the network produces. Despite the success of some recent work in learning an interpretable latent code, the field still lacks a coherent framework to learn a fully interpretable latent code, without any random part for sample diversity. Consequently, it is generally hard, if not impossible, for a non-expert user to produce a desired image by tuning the random and interpretable parts of the latent code. In this paper, we introduce the Preference-Based Image Generation (PbIG), a new method to retrieve the corresponding latent code of the user's mental image. We propose to adopt preference-based reinforcement learning, which learns from a user's judgment of the generated images by a pre-trained generative model. Since the proposed method is completely decoupled from the training stage of the underlying generative models, it can easily be adopted by any method, such as GANs and VAEs. We evaluate the effectiveness of PbIG framework using a set of experiments on baseline datasets using a pretraind StackGAN++.
更多查看译文
关键词
pre-trained generative model,Preference-Based Image Generation,deep generative models,mental image,preference-based reinforcement learning,fully interpretable latent code learning,random latent code learning,PbIG,latent code retrieval,pretraind StackGAN++
AI 理解论文
溯源树
样例
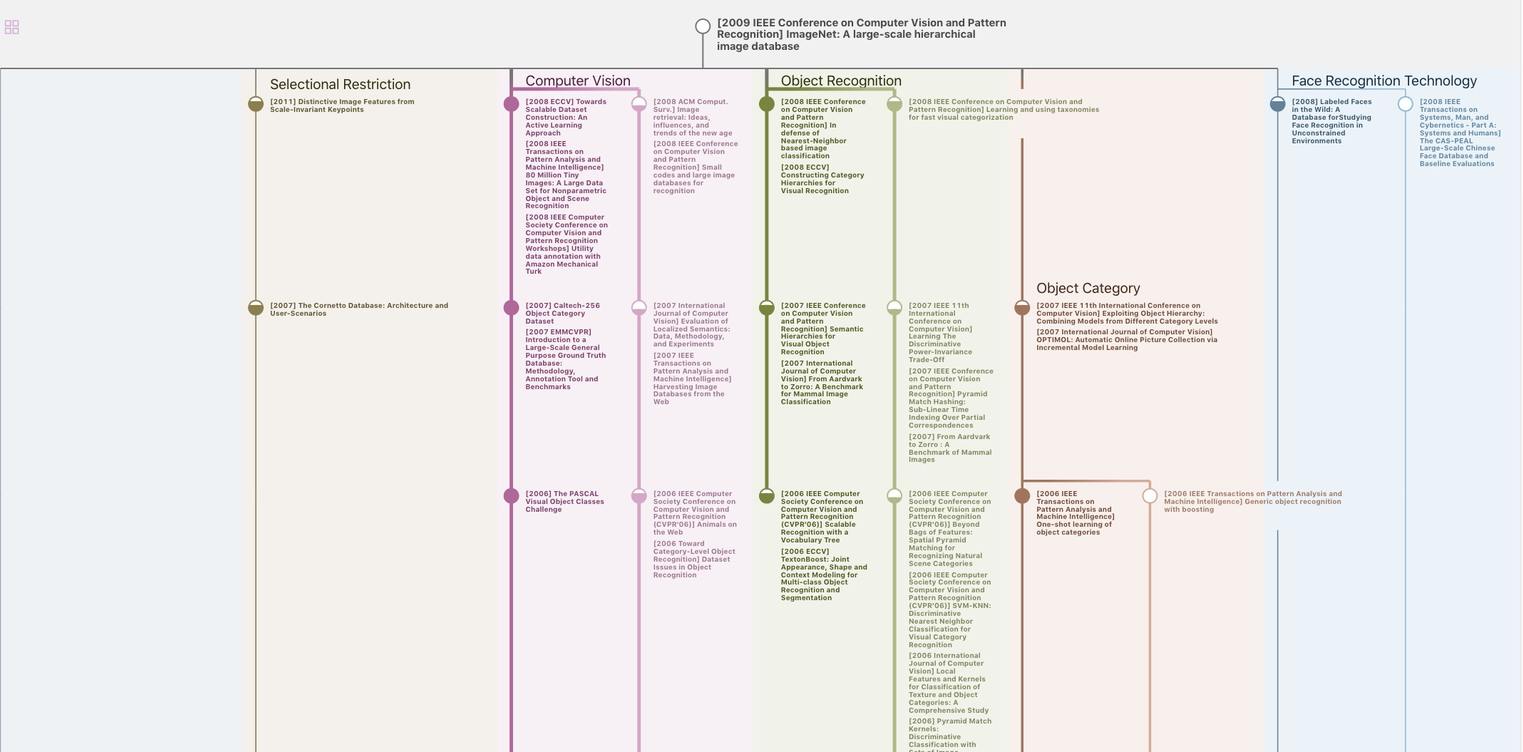
生成溯源树,研究论文发展脉络
Chat Paper
正在生成论文摘要