Adapting Style And Content For Attended Text Sequence Recognition
2020 IEEE WINTER CONFERENCE ON APPLICATIONS OF COMPUTER VISION (WACV)(2020)
摘要
In this paper, we address the problem of learning to perform sequential OCR on photos of street name signs in a language for which no labeled data exists. Our approach leverages easily-generated synthetic data and existing labeled data in other languages to achieve reasonable performance on these unlabeled images, through a combination of a novel domain adaptation technique based on gradient reversal and a multi-task learning scheme. In order to accomplish this, we introduce and release two new datasets - Hebrew Street Name Signs (HSNS) and Synthetic Hebrew Street Name Signs (SynHSNS) - while also making use of the existing French Street Name Signs (FSNS) dataset. We demonstrate that by using a synthetic dataset of Hebrew characters and a labeled dataset of French street name signs in natural images, it is possible to achieve a significant improvement on real Hebrew street name sign transcription, where the synthetic Hebrew data and real French data each overlap with different features of the images we wish to transcribe.
更多查看译文
关键词
natural images,Hebrew street name sign dataset,synthetic Hebrew street name sign dataset,French street name signs dataset,multitask learning,Hebrew street name sign transcription,Hebrew characters,synthetic dataset,domain adaptation,sequential OCR,attended text sequence recognition
AI 理解论文
溯源树
样例
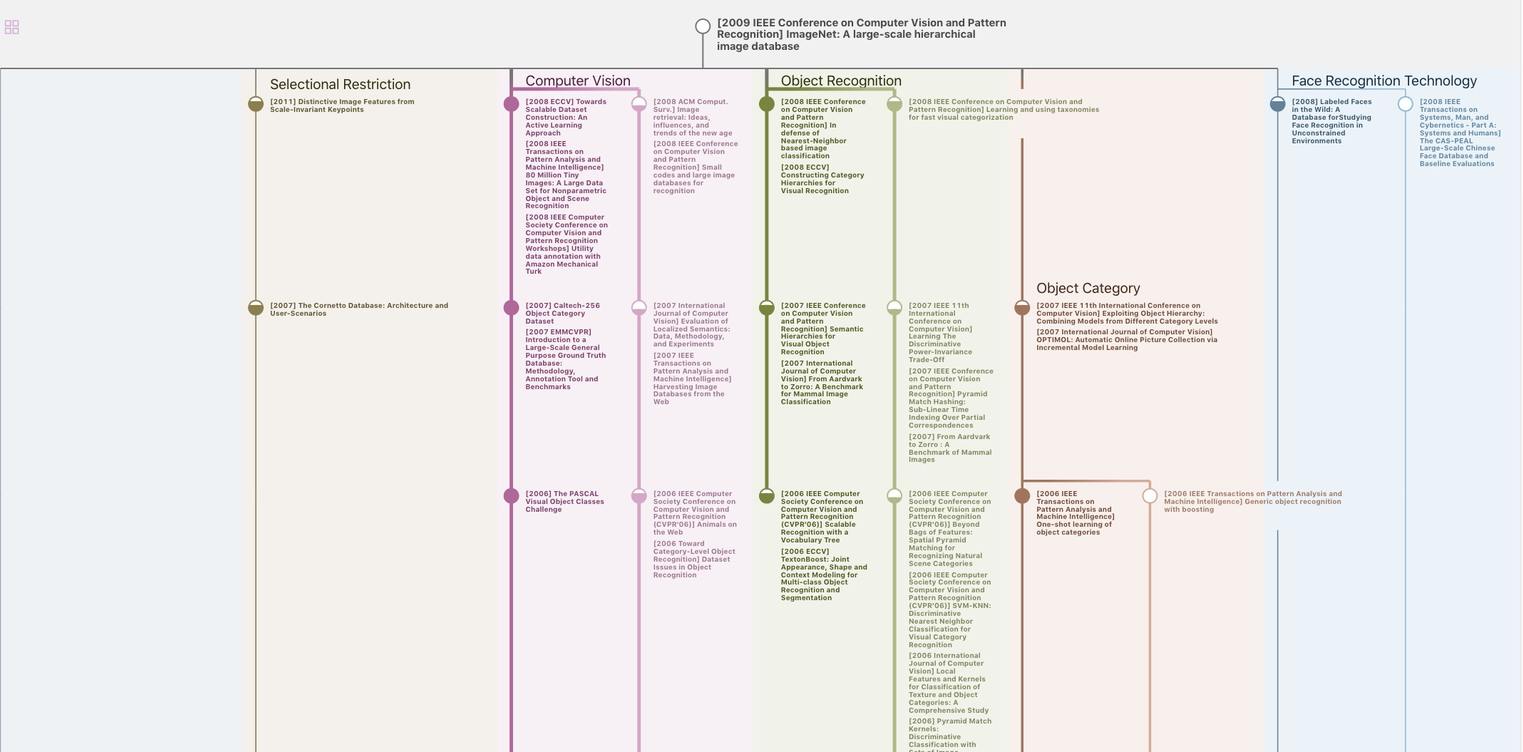
生成溯源树,研究论文发展脉络
Chat Paper
正在生成论文摘要