Dual-Mode Training with Style Control and Quality Enhancement for Road Image Domain Adaptation.
WACV(2020)
摘要
Dealing properly with different viewing conditions remains a key challenge for computer vision in autonomous driving. Domain adaptation has opened new possibilities for data augmentation, translating arbitrary road scene images into different environmental conditions. Although multimodal concepts have demonstrated the capability to separate content and style, we find that existing methods fail to reproduce scenes in the exact appearance given by a reference image. In this paper, we address the aforementioned problem by introducing a style alignment loss between output and reference image. We integrate this concept into a multimodal unsupervised image-to-image translation model with a novel dual-mode training process and additional adversarial losses. Focusing on road scene images, we evaluate our model in various aspects including visual quality and feature matching. Our experiments reveal that we are able to significantly improve both style alignment and image quality in different viewing conditions. Adapting concepts from neural style transfer, our new training approach allows to control the output of multimodal domain adaptation, making it possible to generate arbitrary scenes and viewing conditions for data augmentation.
更多查看译文
关键词
style control,quality enhancement,road image domain adaptation,computer vision,autonomous driving,data augmentation,road scene images,style alignment loss,multimodal unsupervised image-to-image translation model,feature matching,image quality,neural style transfer,multimodal domain adaptation,dual-mode training
AI 理解论文
溯源树
样例
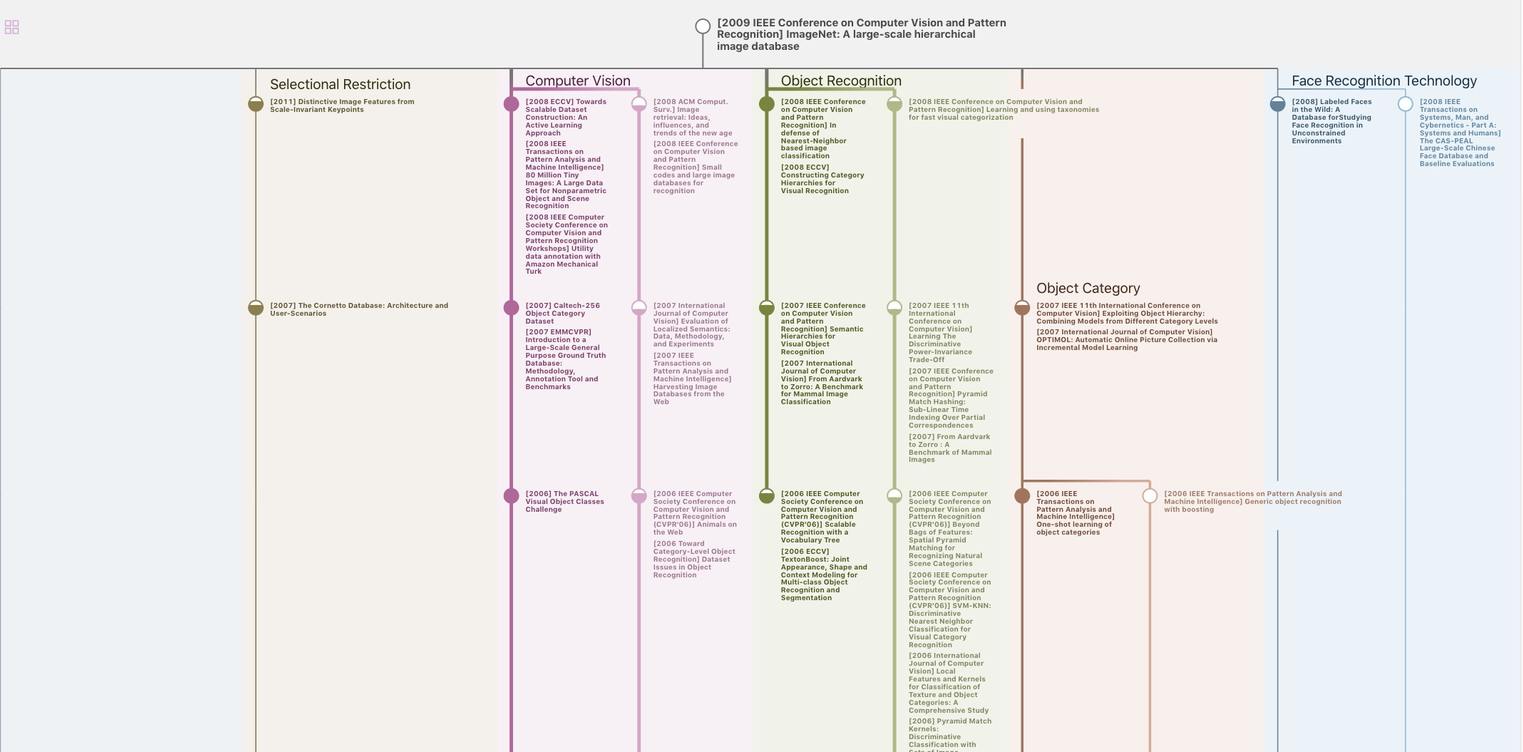
生成溯源树,研究论文发展脉络
Chat Paper
正在生成论文摘要