Combining Multiple Functional Connectivity Methods to Improve Causal Inferences
Periodicals(2021)
摘要
AbstractCognition and behavior emerge from brain network interactions, suggesting that causal interactions should be central to the study of brain function. Yet, approaches that characterize relationships among neural time series—functional connectivity (FC) methods—are dominated by methods that assess bivariate statistical associations rather than causal interactions. Such bivariate approaches result in substantial false positives because they do not account for confounders (common causes) among neural populations. A major reason for the dominance of methods such as bivariate Pearson correlation (with functional MRI) and coherence (with electrophysiological methods) may be their simplicity. Thus, we sought to identify an FC method that was both simple and improved causal inferences relative to the most popular methods. We started with partial correlation, showing with neural network simulations that this substantially improves causal inferences relative to bivariate correlation. However, the presence of colliders (common effects) in a network resulted in false positives with partial correlation, although this was not a problem for bivariate correlations. This led us to propose a new combined FC method (combinedFC) that incorporates simple bivariate and partial correlation FC measures to make more valid causal inferences than either alone. We release a toolbox for implementing this new combinedFC method to facilitate improvement of FC-based causal inferences. CombinedFC is a general method for FC and can be applied equally to resting-state and task-based paradigms.
更多查看译文
关键词
connectivity,causal inference,brain networks,functional connectivity,network neuroscience
AI 理解论文
溯源树
样例
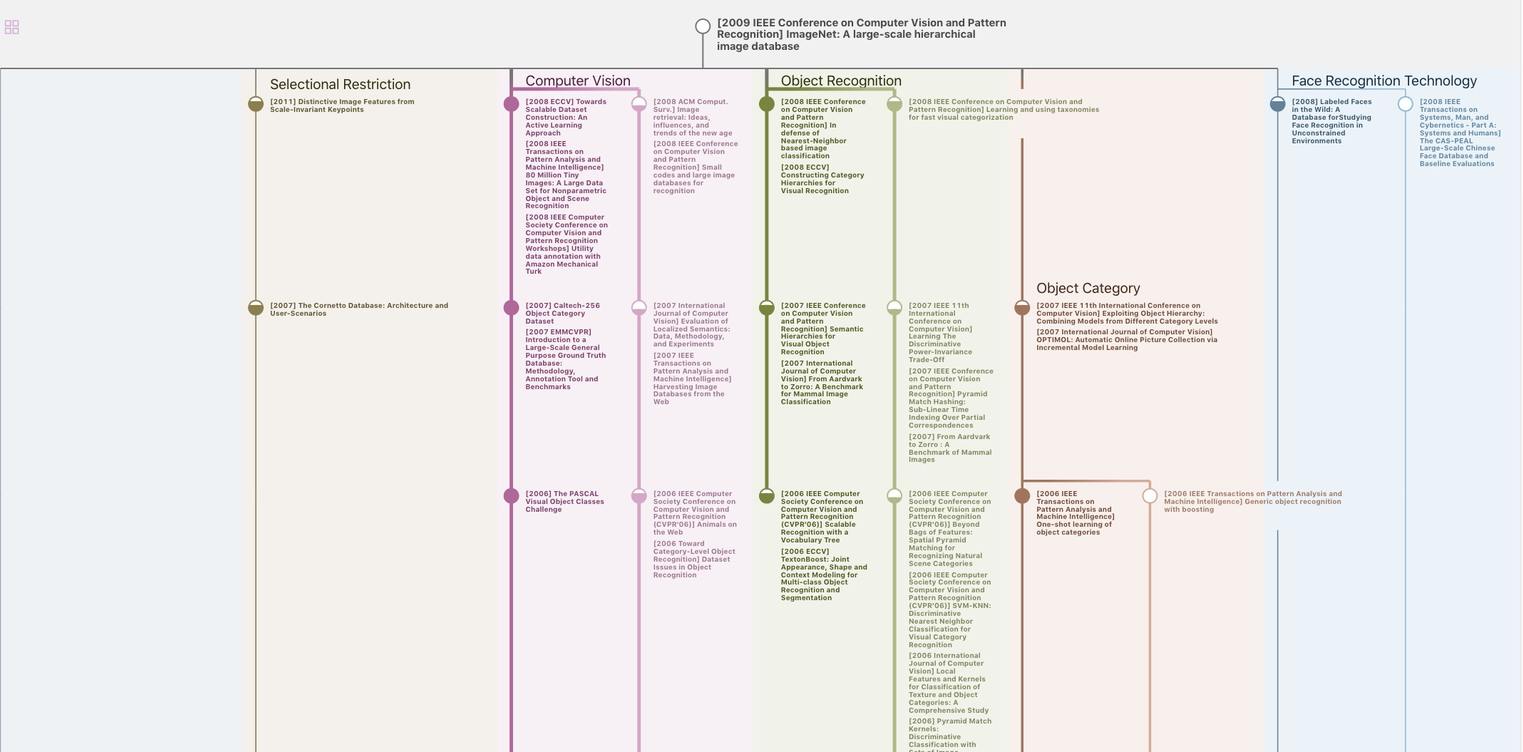
生成溯源树,研究论文发展脉络
Chat Paper
正在生成论文摘要