Intra- and Inter-Action Understanding via Temporal Action Parsing
2020 IEEE/CVF Conference on Computer Vision and Pattern Recognition (CVPR)(2020)
摘要
Current methods for action recognition primarily rely on deep convolutional networks to derive feature embeddings of visual and motion features. While these methods have demonstrated remarkable performance on standard benchmarks, we are still in need of a better understanding as to how the videos, in particular their internal structures, relate to high-level semantics, which may lead to benefits in multiple aspects, e.g. interpretable predictions and even new methods that can take the recognition performances to a next level. Towards this goal, we construct TAPOS, a new dataset developed on sport videos with manual annotations of sub-actions, and conduct a study on temporal action parsing on top. Our study shows that a sport activity usually consists of multiple sub-actions and that the awareness of such temporal structures is beneficial to action recognition. We also investigate a number of temporal parsing methods, and thereon devise an improved method that is capable of mining sub-actions from training data without knowing the labels of them. On the constructed TAPOS, the proposed method is shown to reveal intra-action information, i.e. how action instances are made of sub-actions, and inter-action information, i.e. one specific sub-action may commonly appear in various actions.
更多查看译文
关键词
action recognition,temporal parsing methods,intra-action information,action instances,inter-action information,specific sub-action,inter-action understanding,temporal action parsing,deep convolutional networks,derive feature embeddings,visual motion features,particular their internal structures,high-level semantics,recognition performances,sport videos,multiple sub-actions,temporal structures
AI 理解论文
溯源树
样例
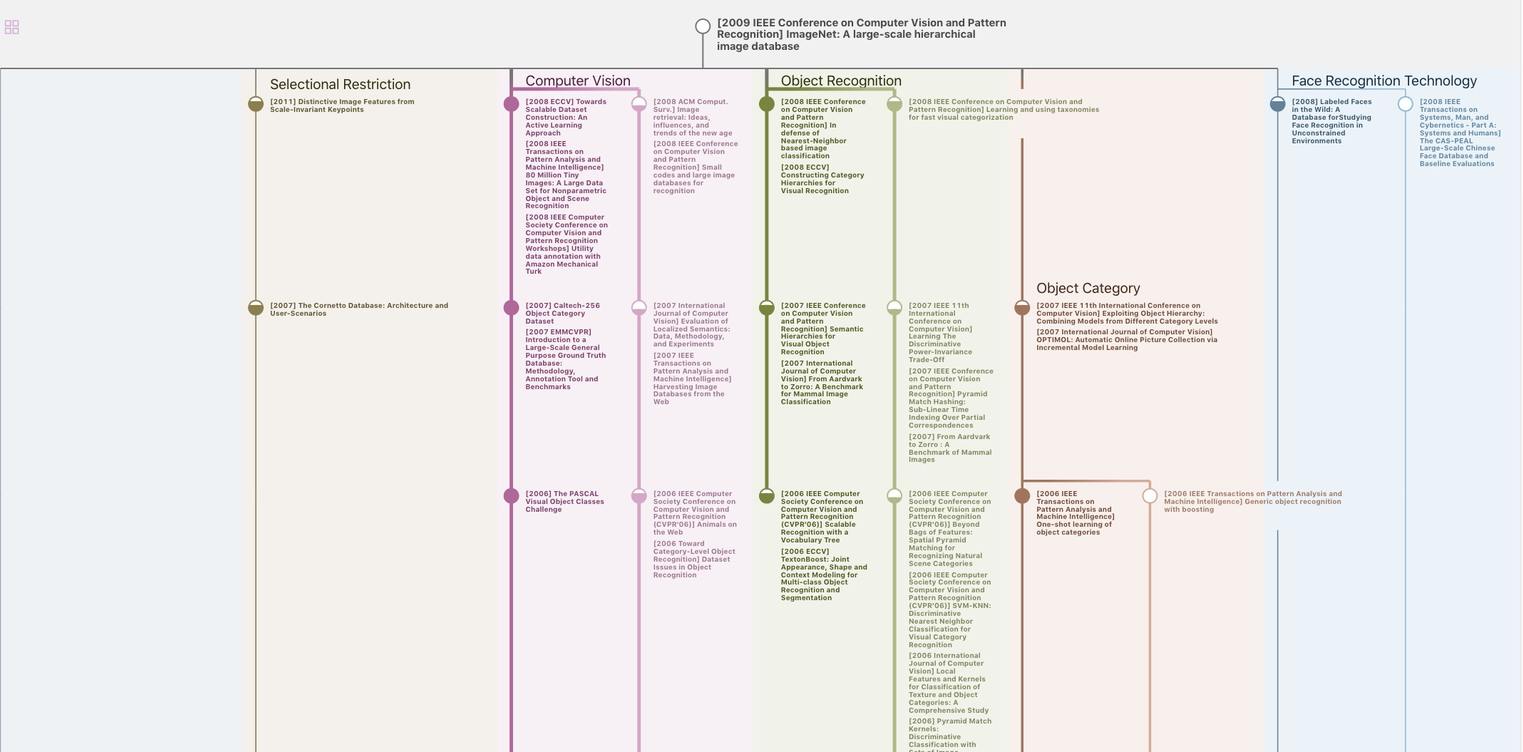
生成溯源树,研究论文发展脉络
Chat Paper
正在生成论文摘要