Gender Slopes: Counterfactual Fairness for Computer Vision Models by Attribute Manipulation
MM '20: The 28th ACM International Conference on Multimedia Seattle WA USA October, 2020(2020)
摘要
Automated computer vision systems have been applied in many domains including security, law enforcement, and personal devices, but recent reports suggest that these systems may produce biased results, discriminating against people in certain demographic groups. Diagnosing and understanding the underlying true causes of model biases, however, are challenging tasks because modern computer vision systems rely on complex black-box models whose behaviors are hard to decode. We propose to use an encoder-decoder network developed for image attribute manipulation to synthesize facial images varying in the dimensions of gender and race while keeping other signals intact. We use these synthesized images to measure counterfactual fairness of commercial computer vision classifiers by examining the degree to which these classifiers are affected by gender and racial cues controlled in the images, e.g., feminine faces may elicit higher scores for the concept of nurse and lower scores for STEM-related concepts.
更多查看译文
关键词
gender
AI 理解论文
溯源树
样例
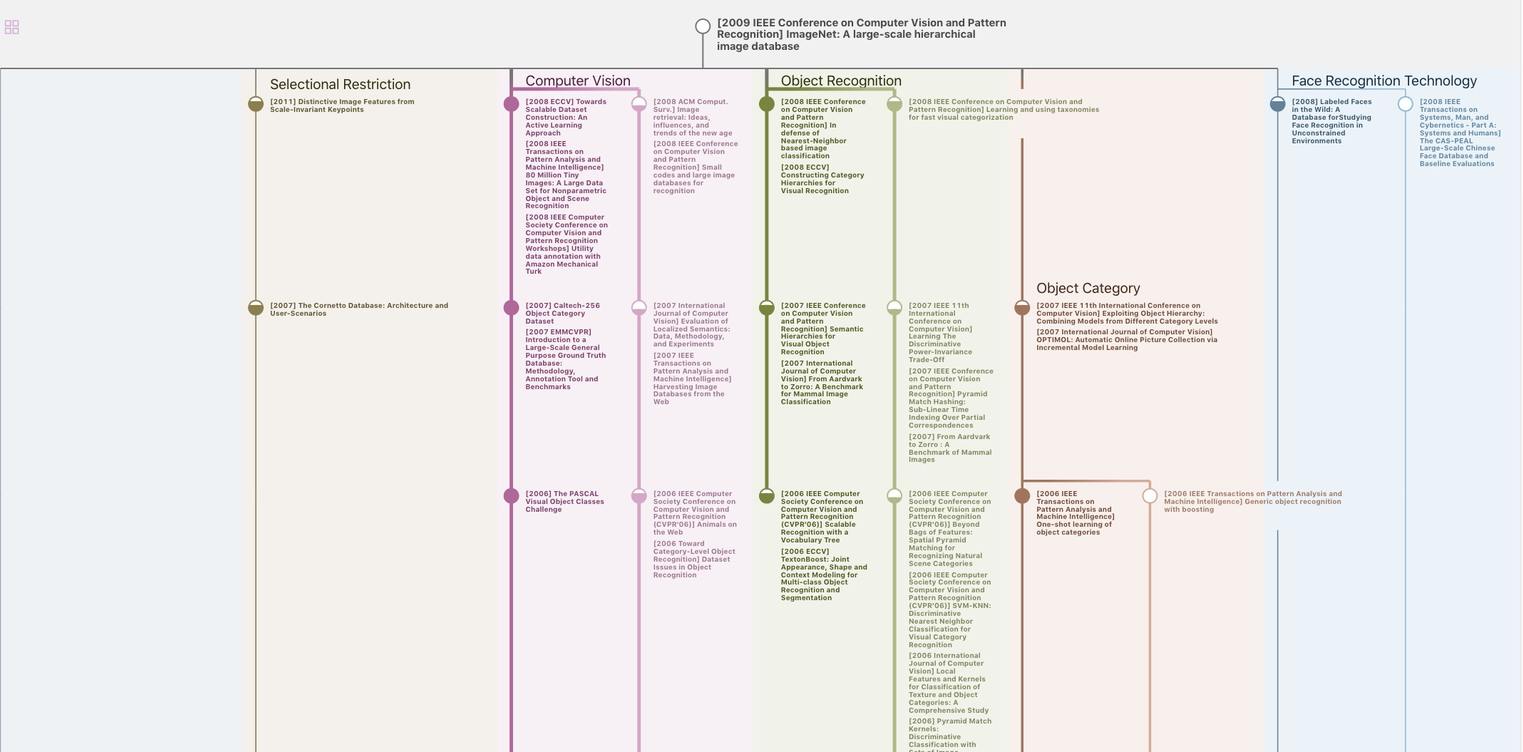
生成溯源树,研究论文发展脉络
Chat Paper
正在生成论文摘要