Transfer Learning via Contextual Invariants for One-to-Many Cross-Domain Recommendation
SIGIR '20: The 43rd International ACM SIGIR conference on research and development in Information Retrieval Virtual Event China July, 2020(2020)
摘要
The rapid proliferation of new users and items on the social web has aggravated the gray-sheep user/long-tail item challenge in recommender systems. Historically, cross-domain co-clustering methods have successfully leveraged shared users and items across dense and sparse domains to improve inference quality. However, they rely on shared rating data and cannot scale to multiple sparse target domains (i.e., the one-to-many transfer setting). This, combined with the increasing adoption of neural recommender architectures, motivates us to develop scalable neural layer-transfer approaches for cross-domain learning. Our key intuition is to guide neural collaborative filtering with domain-invariant components shared across the dense and sparse domains, improving the user and item representations learned in the sparse domains. We leverage contextual invariances across domains to develop these shared modules, and demonstrate that with user-item interaction context, we can learn-to-learn informative representation spaces even with sparse interaction data. We show the effectiveness and scalability of our approach on two public datasets and a massive transaction dataset from Visa, a global payments technology company (19% Item Recall, 3x faster vs. training separate models for each domain). Our approach is applicable to both implicit and explicit feedback settings.
更多查看译文
关键词
Cross-Domain Recommendation, Contextual Invariants, Transfer Learning, Neural Layer Adaptation, Data Sparsity
AI 理解论文
溯源树
样例
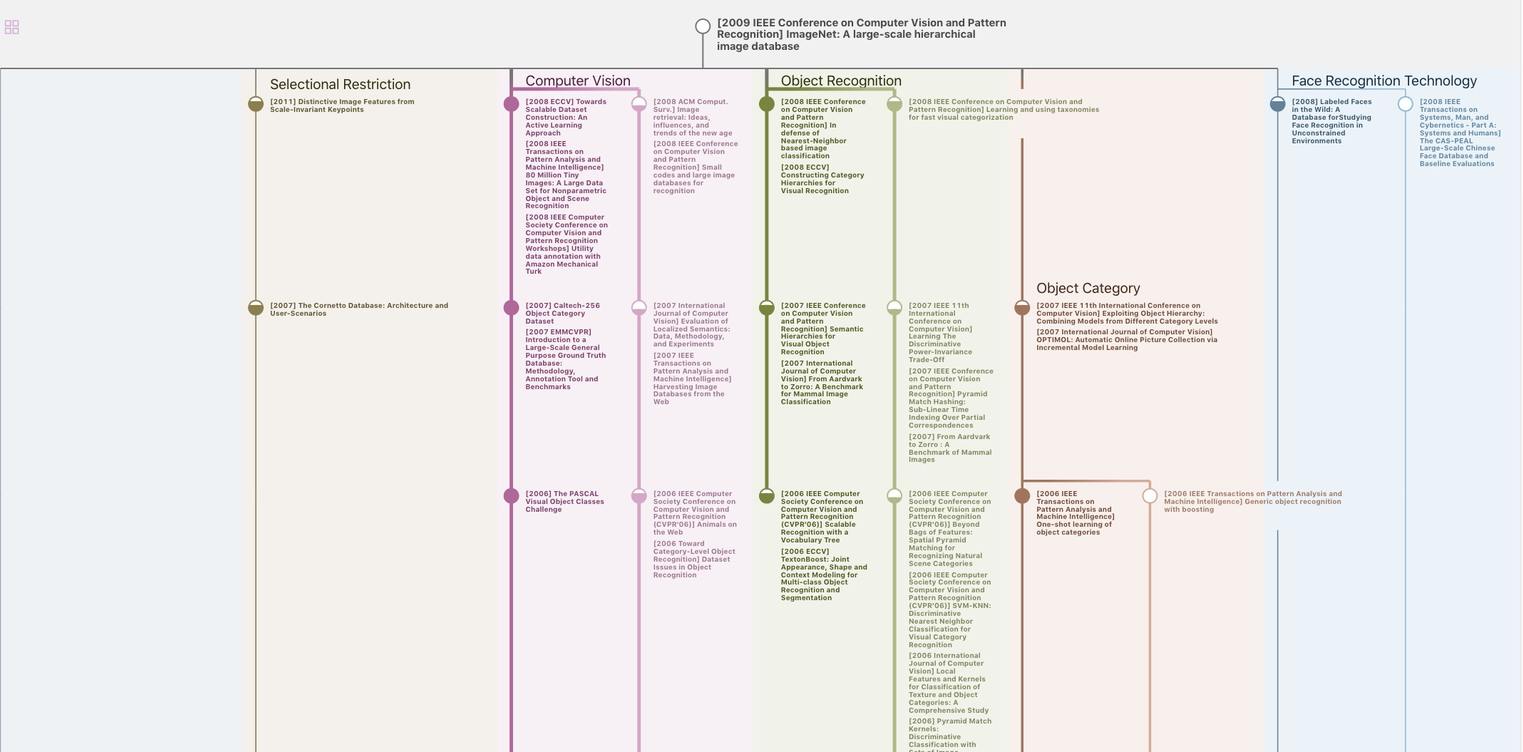
生成溯源树,研究论文发展脉络
Chat Paper
正在生成论文摘要